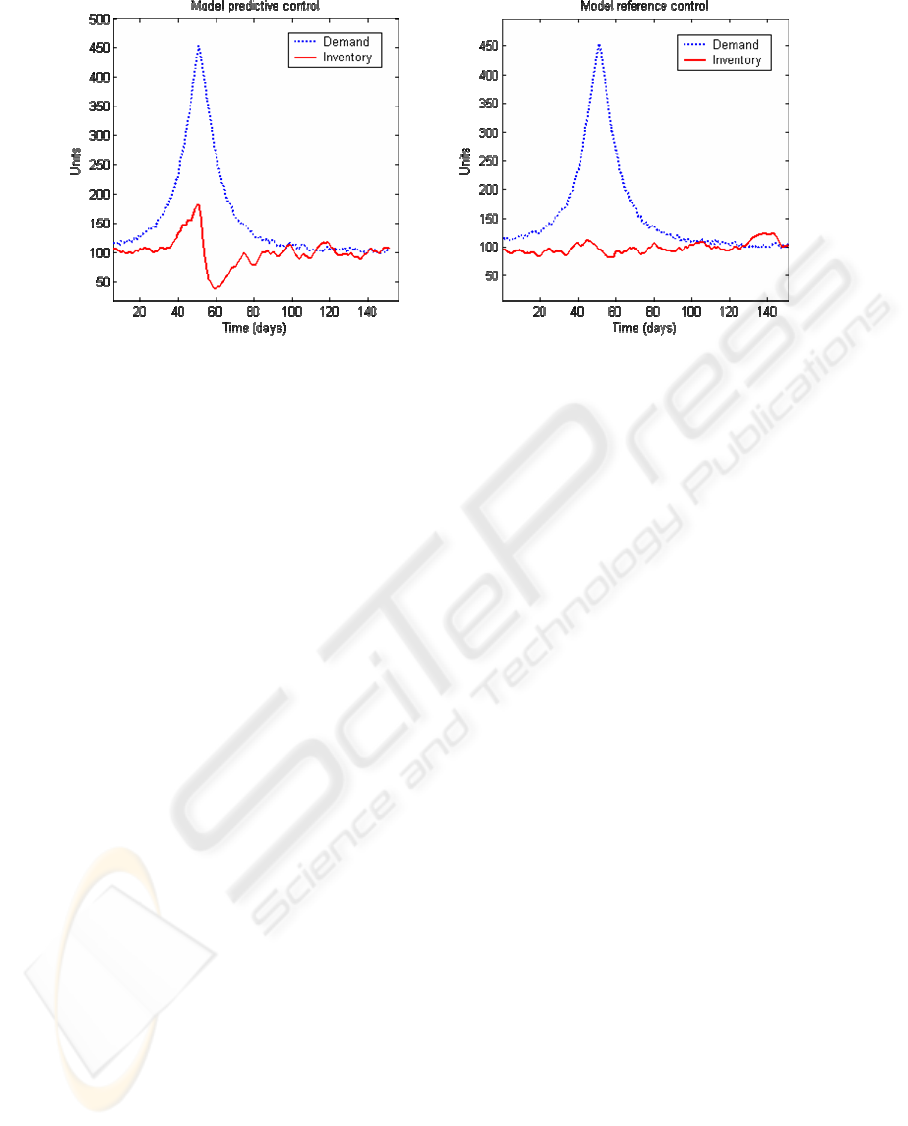
4 CONCLUSIONS
In this study we presented a solution to the
problematic determining of the cost parameter
penalizing changes made in the control in model
predictive controller used in business related
systems. The model reference control was studied
and simulations performed to demonstrate the
abilities and advanteges of this control method. It
has been shown, that the model reference control
method is an effective way to control an inventory
and most of all that the method allows us to avoid
the problematic parameter λ in the equation 1. This
reduction of a very problematic parameter is most
definitely inevitable if any kind of practical solutions
are ever desired. Therefore all future research
concerning business related control should concider
this. It should also be kept in mind that any
reduction of tuneable parameters can be seen as an
advantage.
Also we showed in this study that the model
reference control is at least as applicable in
inventory control as model predictive control if not
even better. The simple, yet effective and smooth
response model reference control provides suits
perfectly to the unstable and variating environment
of business related systems. It can also be said that
the filter-like behaviour is desireable in order to
reduce the bullwhip behaviour, but further research
is needed on this field.
A new supply chain simulator interface was also
presented and used in the simulations of this study.
The set of universal supply chain blocks gives an
opportunity of testing and simulating the perforance
of different control methods or even different forms
of supply chains without reprogramming the basic
elements of inventory and production.
REFERENCES
Figure 5: The inventory responses of both controllers to a gaussian-shaped demand pattern.
Camacho, E. F. and Bordons, C., 2002, Model Predictive
Control.
Springer-Verlag London Limited
Hennet, J.-C., 2003. A bimodal scheme for multi-stage
production and inventory control. In Automatica, Vol
39, pp. 793-805.
Koivisto, H., Kimpimäki, P., Koivo, H., 1991. Neural
predictive control – a case study. In 1991 IEEE
International Symposium on Intelligent Control. IEEE
Press.
Lambert, E. P., 1987. Process Control Applications of
Long-Range Prediction. PhD Thesis, University of
Oxford. Trinity.
Maciejowski, J. M., 2002, Predictive Control with
Constraints. Pearson Education Limited
Rivera, D. E., Braun, M. W., Flores, M. E., Carlyle, W.
M., Kempf, K. G., 2003. A Model predictive control
framework for robust management of multi-product,
multi-echelon demand networks. In Annual Reviews in
Control, Vol. 27, pp. 229-245.
Towill, D. R., 1996. Industrial dynamics modeling of
supply chains. In International Journal of Physical
Distribution & Logistics Management, Vol. 26, No. 2,
pp.23-42.
Tzafestas, S., Kapsiotis, G., Kyriannakis, E., 1997. Model-
based predictive control for generalized production
problems. In Computers in Industry, Vol. 34, pp. 201-
210.
Ydstie, B. E., Grossmann, I. E., Perea-López, E., 2003, A
Model predictive control strategy for supply chain
optimization. In Computers and Chemical
Engineering, Vol 27, pp. 1202-1218.
ICINCO 2004 - INTELLIGENT CONTROL SYSTEMS AND OPTIMIZATION
134