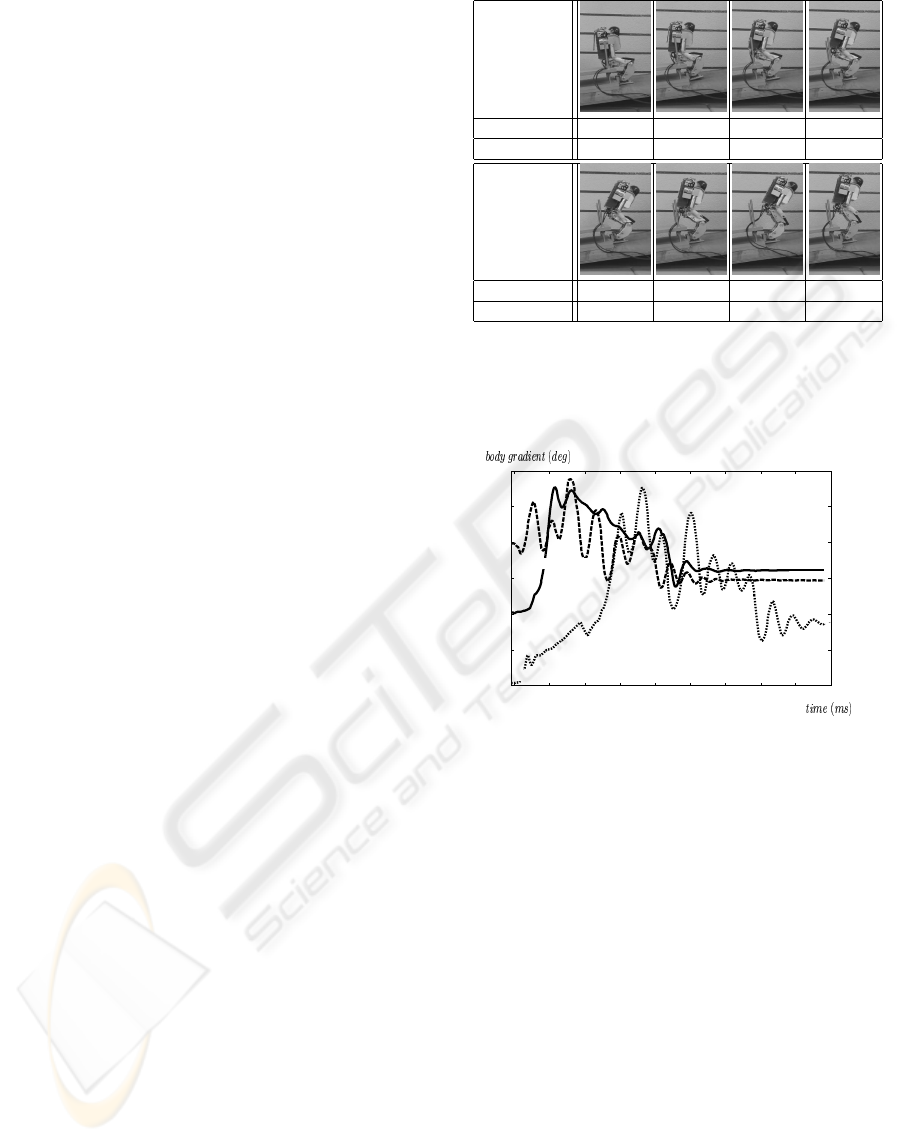
this particular case, the system can generate the stand
up motion with only GP
5
. Figure 9 shows the snap-
shots of a standing motion by our system, where the
gradient of ground is 10.0 (deg) backward. In this
particular case, the system can generate the stand up
motion with only GP
1
and GP
3·4
. Figure 10 shows
the trajectory of the gradient of HOAP-1 body when
it executes the motions obtained by search. In the fig-
ure, solid, dashed and dotted lines show the trajectory
of the gradient of HOAP-1’s body standing up from
chair on the flat, the forward slope and the backward
slope, respectively. The two broken lines, at the be-
ginning of the motion, show that the gradients of the
body on the slopes are both different from that on the
flat. The difference corresponds to the gradient of the
slope. This is obvious, for HOAP-1 is sitting on a
chair on the slope. Through the movement, the differ-
ence of the gradient is attenuated gradually. The re-
sults indicates that the motion control adapts the mo-
tion to the different environments.
5 CONCLUSION
This paper proposed a concept learning-based ap-
proach to motion control for humanoid robots. The
motion generation system had been implemented with
decision tree learner C4.5 and depth first search tech-
nique. Some stable and anti-tumble motions to stand
up from a chair were performed by humanoid robot
HOAP-1. In future work, we will dedicate to the im-
provement of our system for more complex motions
and to the investigation of the relations of the suitable
number between guideposts and examples for learn-
ing.
REFERENCES
Capi, G., Nasu, Y., and Barolli, L. (2002). A new gait op-
timization approach based on genetic algorithm for
walking biped robots and a neural networks imple-
mentation. Journal of IPSJ, 43(4):1039–1049.
Kuroki, Y., Ishida, T., Nagasaka, K., and Yamaguchi, J.
(2002). A small biped walking entertainment robot
sdr-4x with a highly integrated motion control. In
Proc. of the 20-th conf. of Robotics Society of Japan,
page 1C34. (in Japanese).
Morimoto, J. and Doya, K. (2000). Acquisition of stand-up
behavior by a real robot using hierarchical reinforce-
ment learning. In Proc. of International Conference
on Machine Learning, pages 623–630.
Murase, Y., Yasukawa, Y., Sakai, K., and et al. (2001).
Design of a compact humanoid robot as a plat-
form. In Proc. of the 19-th conf. of Robotics
guidepost ID 0 1 1 1
time (msec) 0 1000 2000 3000
guidepost ID 5 5 5 FINAL
time (msec) 3500 4250 5000 6250
Figure 9: The snapshot of a standing motion generated by
the system, where the ground has gradient of 10.0 (deg)
backward.
-10
-5
0
5
10
15
20
0 1000 2000 3000 4000 5000 6000 7000 8000 9000
flat
10.0 (deg) forward
10.0 (deg) backward
Figure 10: Trajectory of gradient of the body changing gra-
dient of the ground.
Society of Japan, pages 789–790. (in Japanese),
http://pr.fujitsu.com/en/news/2001/09/10.html.
Noritake, K., Kato, S., Yamakita, T., and Itoh, H. (2003).
A motion generation system for humanoid robots – tai
chi motion –. In Proc. of International Symposium on
Micromechatoronics and Human Science, pages 265–
269.
Quinlan, J. R. (1993). C4.5: Programs for Machine Learn-
ing. Morgan Kauffman.
Taga, G. (1995). A model of the nueo-musculo-skeletal sys-
tem for human locomotion, i. emergence of basic gait.
Biological Cybernetics, 73:97–111.
Vukobratovic, M., Frank, A. A., and Juricic, D. (1970).
On the stability of biped locomotion. IEEE Trans. on
Biomedical Engineering, 17(1):25–36.
ICINCO 2004 - ROBOTICS AND AUTOMATION
338