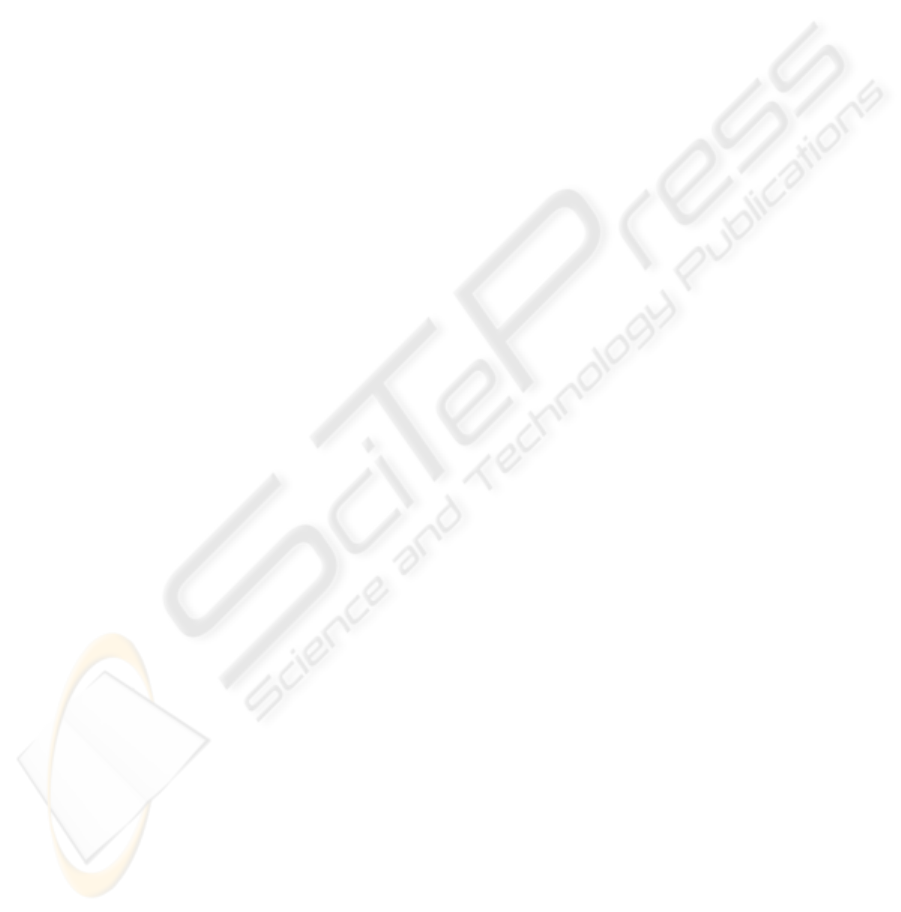
5 CONCLUSIONS
In this paper we foster deeper studies in the
management of contextual information in robotic
vision. In the first part, we proposed an operative
definition of “context” to identify the variable parts
of a perceptive system susceptible of becoming
inappropriate in case of contextual changes: models,
operators, and decision policies.
In the second part, we described a novel Bayesian
framework (i.e. Context Commutation) to implement
contextual opportunistic switching. Dedicated
algorithms, called daemons, observe some
environmental features showing a correlation with
system performance ratings rather than with the
target signal (e.g. people tracking). When such
features change, the system commutes its state to a
more reliable configuration.
Critical points in Context Commutation are
mainly related to its Bayesian framework.
Parameters like sensor models of daemons and
coefficients of the transition matrix need thorough
tuning and massive training data. An error in such
parameters would corrupt correct contextual
switching.
Possible points for future work are: i) exploring
switching reliability with incorrect parameters, ii)
studying Context Commutation with more than eight
states, iii) extending the framework to perceptive
systems including sensors other than solely vision.
REFERENCES
Coutelle, C., 1995. Conception d’un système à base
d’opérateurs de vision rapides, PhD thesis (in French),
Université de Paris Sud (Paris 11), Paris, France.
Crowley, J.L., J. Coutaz, G. Rey, P. Reignier, 2002.
Perceptual Components for Context Aware
Computing. In Proc. UBICOMP2002, Sweden,
available at http://citeseer.nj.nec.com/541415.html.
Dessoude, O., 1993. Contrôle Perceptif en milieu hostile:
allocation de ressources automatique pour un système
multicapteur, PhD thesis (in French), Université de
Paris Sud (Paris 11), Paris, France.
Draper, B.A., J.Bins, K.Baek, 1999. ADORE: Adaptive
Object Recognition. In Proc. ICVS99, pp. 522-537.
Dubes, R. C., Jain, A. K., 1989. Random Field Models in
Image Analysis. In J. Applied Statistics, v. 16, pp.
131-164.
Ebner, M., A. Zell, 1999. Evolving a task specific image
operator. In Proc. 1
st
European Wshops on
Evolutionary Image Analysis, Signal Processing and
Telecommunications, Göteborg, Sweden, Springer-
Verlag, pp. 74-89.
Horswill, I., 1995. Analysis of Adaptation and
Environment. In Artificial Intelligence, v.73(1-2), pp.
1-30, 1995.
Kittler, J., J. Matas, M. Bober, L. Nguyen, 1995. Image
interpretation: Exploiting multiple cues. In Proc. Int.
Conf. Image Processing and Applications, Edinburgh,
UK, pp. 1-5.
Kruppa, H., M. Spengler, B. Schiele, 2001. Context-driven
Model Switching for Visual Tracking. In Proc. 9
th
Int.
Symp. Intell. Robotics Sys., Toulouse, France.
Lombardi, P., 2003. A Model of Adaptive Vision System:
Application to Pedestrian Detection by Autonomous
Vehicles. PhD thesis (in English), Università di Pavia
(Italy) and Université de Paris XI (France).
Merlo, X., 1988. Techniques probabilistes d’intégration et
de contrôle de la perception en vue de son exploitation
par le système de décision d’un robot, PhD thesis (in
French), Université de Paris Sud (Paris 11), Paris,
France.
Rabiner, L.R., 1989. A tutorial on hidden Markov models.
In Proceedings of the IEEE, vol. 77, pp. 257-286.
Rimey, R.D., 1993. Control of Selective Perception using
Bayes Nets and Decision Theory. Available at http://
citeseer.nj.nec.com/rimey93control.html.
Roli, F., G. Giacinto, S.B. Serpico, 2001. Classifier Fusion
for Multisensor Image Recognition. In Image and
Signal Processing for Remote Sensing VI, Sebastiano
B. Serpico, Editor, Proceedings of SPIE, v. 4170,
pp.103-110.
Rosenfeld, A., R.A. Hummel, S.W. Zucker, 1976. Scene
labeling by relaxation operations. In IEEE Trans. Syst.
Man Cybern., v. 6, pp. 420-433.
Shekhar, C., S. Kuttikkad, R. Chellappa, 1996.
KnowledgeBased Integration of IU Algorithms. In
Proc. Image Understanding Workshop, ARPA, v. 2,
pp. 1525-1532, 1996.
Stauffer, C., W.E.L. Grimson, 1999. Adaptive Background
Mixture Models for Real-Time Tracking. In Proc.
IEEE Conf. Comp. Vis. Patt. Rec. CVPR99, pp. 246-
252.
Strat, T.M., 1993. Employing Contextual Information in
Computer Vision. In Proc. DARPA93, pp. 217-229.
Tissainayagam, P., D. Suter, 2003. Contour tracking with
automatic motion model switching. In Pattern
Recognition.
Torralba, A., K.P. Murphy, W.T. Freeman, M.A. Rubin,
2003. Context-based vision system for place and
object recognition. In Proc. ICCV’03, available at
http://citeseer.nj.nec.com/torralba03contextbased.html.
Toyama, K., E.Horvitz, 2000. Bayesian Modality Fusion:
Probabilistic Integration of Multiple Vision
Algorithms for Head Tracking. In Proc. ACCV’00, 4
th
Asian Conf. Comp. Vision, Tapei, Taiwan, 2000.
ICINCO 2004 - SIGNAL PROCESSING, SYSTEMS MODELING AND CONTROL
142