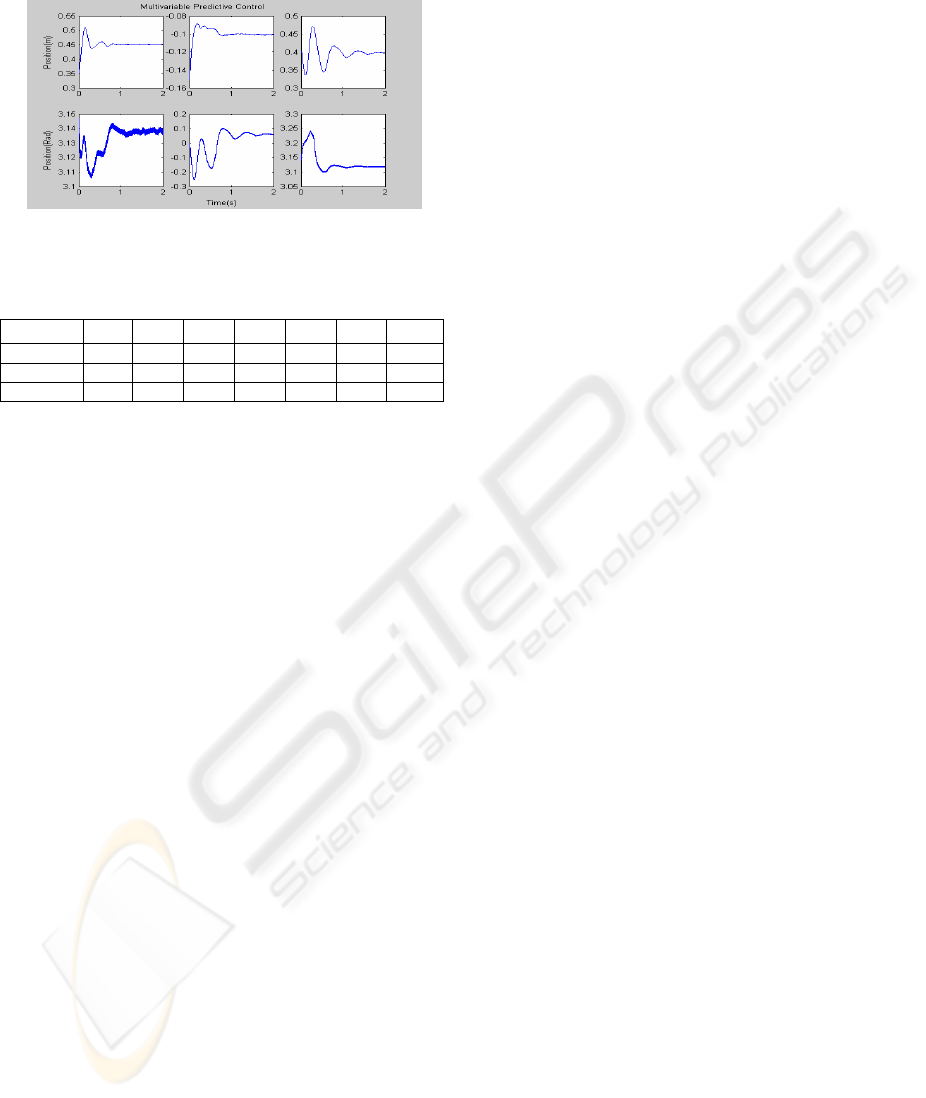
mainly in turn of z and for rotation of the end-
effector.
Figure 6: Results of a 2D architecture using a MPC
controller .
Table 1: r.m.s. values for the control algorithm
SSR
T
x
T
y
T
z
θ
x
θ
y
θ
z
error
PI 2.50 2.40 1.20 2.20 0.30 0.47 1.51
GPC 2.14 0,81 0.22 1.84 0.22 0.02 0.87
MPC 1.36 0.67 3.01 0.76 6.3 6.21 3.04
Table 1 presents the computer errors for each
algorithm which reveals the best performance for the
GPC.
4 CONCLUSIONS
A vision control system for a six degrees of freedom
robot was studied.
A prediction error method was used to identify the
Robot dynamics and implement a predictive control
algorithm ( MPC and GPC).
The controllers (PI, GPC and MPC) were used to
control the robot in a 2D visual servoing
architecture.
The three different algorithms always converge to
the desired position. In general we can conclude that
in visual servoing is obvious the good performance
of both predictive controllers.
The examples show also that the 2D algorithm
associated with the studied controllers allow to
control larger displacements than those referred in
(Gangloff 99).
From the analysis of r.m.s error presented in Table 1
we can conclude the better performance of the GPC.
In spite of better MPC results for the translation in
the xy plane when compared to the GPC, the global
error is worse. These results can still eventually be
improved through a refinement of the controllers
parameters and of the identification procedure. In the
visual servoing trajectory is obvious the good
perfomance of this approach. The identification
procedure has a great influence on the results.
The evaluation of the graphical trajectories and the
computed errors allow finally concluding that the
GPC vision control algorithm leads to the best
performance.
5 FUTURE WORKS
In future works, another kind of controllers such as
intelligent, neural and fuzzy will be used. Other
algorithms to estimate the joints coordinates should
be tested. These algorithms will be applied to the
real robot in visual servoing path planning.
Furthermore others target (no coplanars) and other
visual features should be tested.
ACKNOWLEDGEMENTS
This work is partially supported by the “Programa de
Financiamento Plurianual de Unidades de I&D
(POCTI), do Quadro Comunitário de Apoio III” by
program FEDER and by the FCT project
POCTI/EME/39946/2001.
REFERENCES
Bemporad, A. and M. Morari 1999. Robust Model
Predictive Control: A Survey. Automatic Control
Laboratory, Swiss Federal Institute of
Technology(ETH) Physikstrass
Camacho, E. F. and C. Bordons 1999. Model Predictive
Control. Springer Berlin
Clarke, D.W., C.Mohtafi, and P.S.Tuffs. Generalized
Predictive Control-Part I. The Basic Algorithm,
Automatica, Vol.23, Nº2, pp.137-148
Corke, P. 1996. Visual Control of Robots. Willey
Corke, P. 1994. A Search for Consensus Among Model
Parameters Reported for the Puma 560 In Proc. IEEE
Int. Conf.. Robotics and Automation, pages 1608-1613,
San Diego.
Ferreira, P. e Caldas, P. (2003). 3D and 2D Visual
servoing Architectures for a PUMA 560 Robot, in
Proceedings of the 7
th
IFAC Symposium on Robot
Control, September 1-3, , pp 193-198.
Gangloff, J. 1999. Asservissements visuel rapides D’ un
Robot manipulateur à six degrés de liberté. Thèse de
Doutorat de L’ Université Louis Pasteur.
Lee, J.H,. and B. Cooley-Recent advances in model
predictive control.In:Chemical Process Control,
Vol.93, no. 316. pp201-216b. AIChe Syposium Series
– American Institute of Chemical Engineers.
Mezouar, Y. and F. Chaumette 2001. Images Interpolation
for Image- Based control Under Large Displacements.
Proceedings of the European Control Conference, pp.
2904-2909
.
ICINCO 2004 - ROBOTICS AND AUTOMATION
478