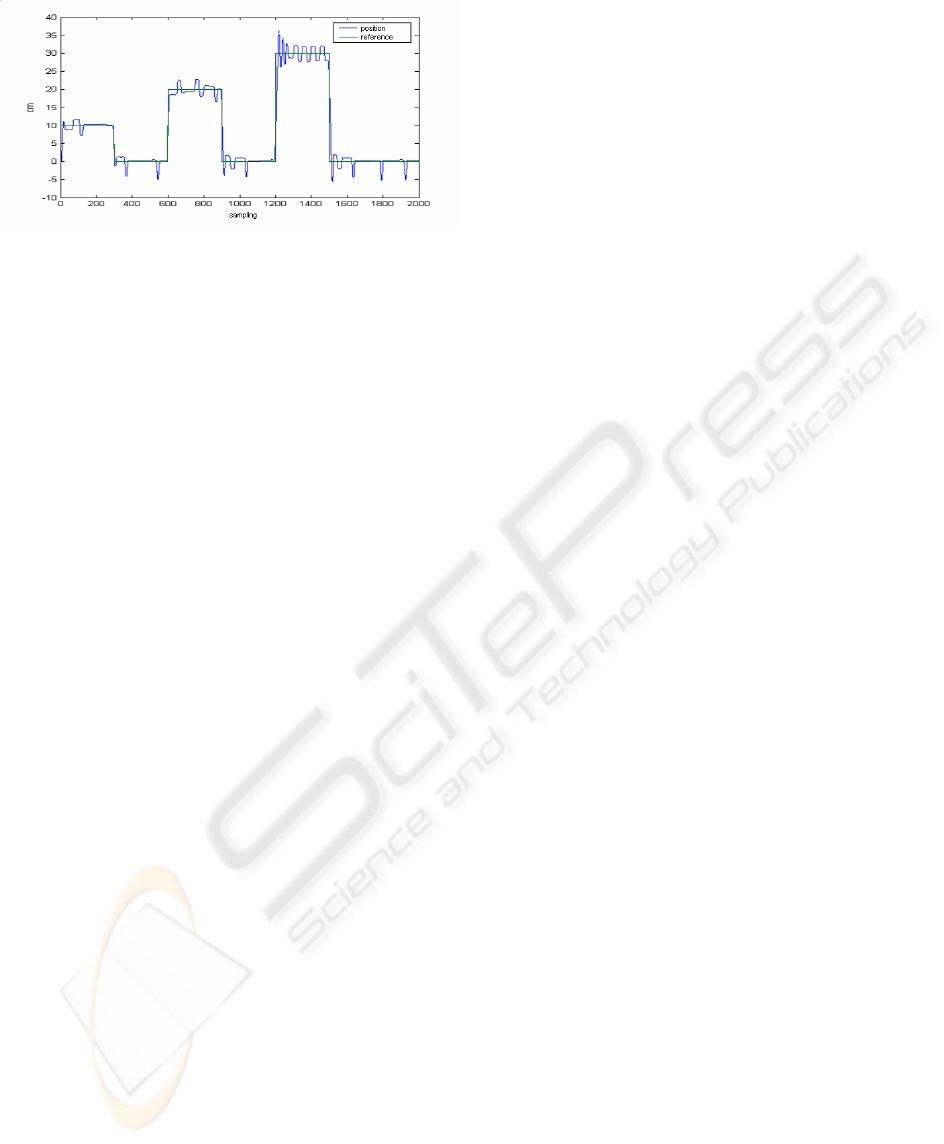
Figure 8: The Worst Response
(Prediction Horizon: 20, Control Horizon: 10,
Lambda:20)
It is observed that in some positions the system
response goes away from the reference and again
starts to follow. This can be explained related to the
high dry friction values in those regions. In the mean
time steady-state errors are eliminated by this
control algorithm as seen in Figure 7.
6 EXPERIMENTAL
INSTALLATION
The system consists of a magnetically coupled
rodless pneumatic cylinder with high precision
guide (SMC CY1HT32, stroke 0.5 m, diameter
0.032 m), two three-way electropneumatic
servovalves (SMC VEP 3121), a magnetic linear
scale (SONY Magnescale SR10-060A, a computer
having a 1.6 GHz microprocessor, 256 MB RAM
and a data acquisition card (Advantech PCL-
812PG). Matlab - Simulink data acquisition software
is used under Windows 98 operating system.
7 CONCLUSION
In this paper we considered a system identification
and a real-time DMC position control on o
pneumatic system. We observed a steady-state error
from the previous studies on the same test bench
with PD controller. In order to eradicate this error
we used Model Predictive Control algorithm.
In Matlab software, it can be seen that there is a
MPC Toolbox which cannot be used in real-time
applications. So we prepared a new real-time usable
Simulink algorithm for unconstrained SISO systems.
The step response coefficients, which are
necessary for the DMC algorithm, were calculated
off-line in this study. It can be said that a self-tuning
DMC application will increase the system’s
performance and will efface the need of an operator.
REFERENCES
Koç, I.M., 1998. Accurate and Robust Pneumatic Position
Control, Master Thesis, Istanbul Technical University,
Institute of Science and Technology, Istanbul.
Cihan, S., 1999. Pneumatic Position Control, Master
Thesis, Istanbul Technical University, Institute of
Science and Technology, Istanbul.
Zorlu, A., 2002. Experimental Modelling and Simulation
of a Pneumatic System, PhD. Thesis, Istanbul
Technical University, Institute of Science and
Technology, Istanbul.
Karahan U., Özsoy C., Kuzucu A., 2003.The real-time
position control of a pneumatic system with rapid
prototyping approach, IFAC Conference, Istanbul.
Camacho E.F., Bordons C., 2000. Model Predictive
Control, Springer-Verlag, London.
Camacho E.F., Bordons C., 1995. Model Predcitive
Control in the Process Industry, Springer-Verlag,
London.
Morari M., 1993. Model predictive control:Multivariable
control technique of choice in the 1990s?, California
Institude of Technology, CIT/CDS 93-024, Technical
Reports.
Qin S.J., Badgwell T.A., 1997. An overview of industrial
model predictive control technology, Chemical
Process Control-AIChE Symposium Series, 232-256
Cutler C. R., Ramaker B. L., 1979. Dynamic matrix
control-a computer control algorithm, AIChE National
Meeting, Houston.
De Keyser R.M.C., Van De Velde G.A., Dumortier
F.A.G., 1988. A comparative study of self-adaptive
long-range predictive control methods, Automatica,
Vol 24, No 2, 149-163.
Pandian, S.R., Hayakawa, Y., Kanazawa, Y., Kamayama,
Y., Kawamura, S., 1997. Practical design of a sliding
mode controller for pneumatic actuators, Transactions
of the ASME, Journal of Dynamic Systems,
Measurement, and Control, 119, 666-674.
Morari M., Ricker N.L., 1998. Matlab Model Predictive
Control Toolbox user’s guide version 1.0, The
Mathworks Inc., Natick.
Ljung, L., 2002. Matlab System Identification Toolbox
user’s guide version 5.0.2, The Mathworks Inc.,
Natick.
Mathworks, 2002. Matlab Real-Time Workshop user’s
guide version 5.0, The Mathworks Inc., Natick.
REAL-TIME POSITION CONTROL OF A PNEUMATIC SYSTEM USING MODEL PREDICTIVE CONTROL
113