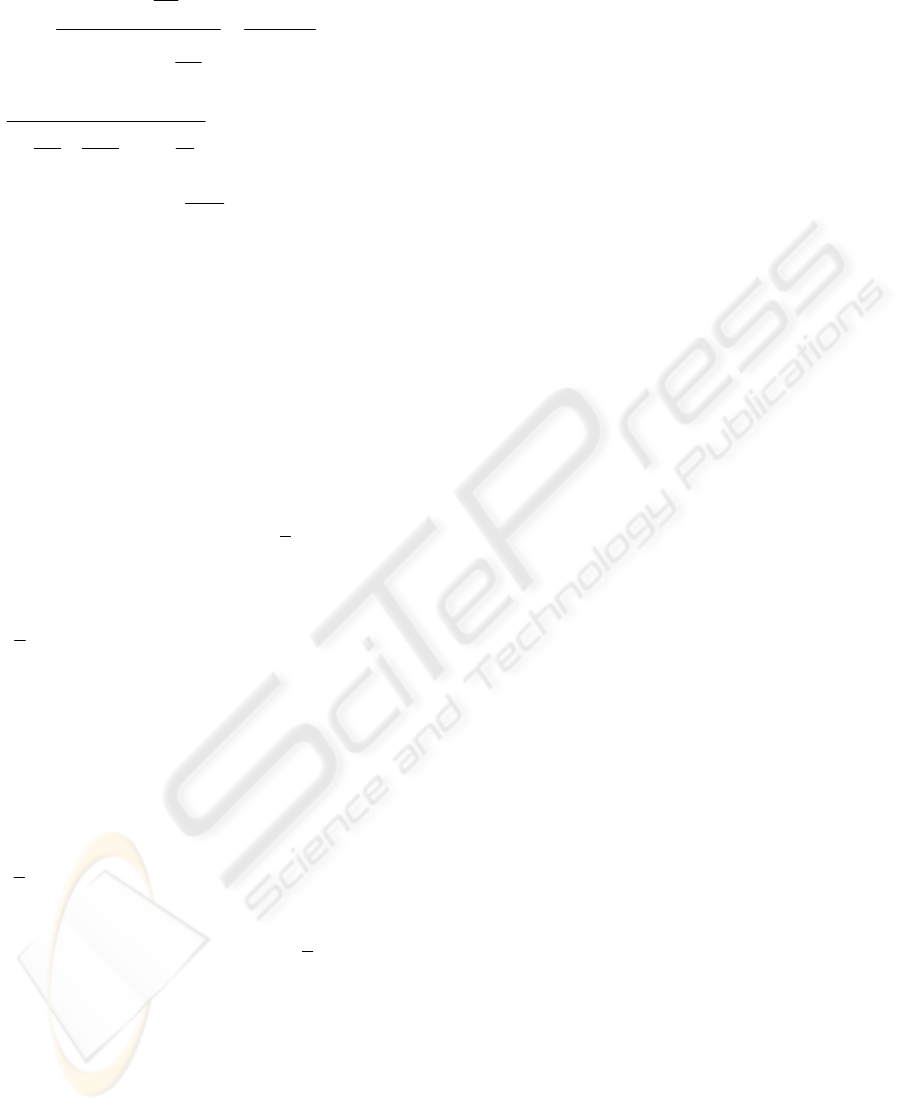
∑
∑
−
=
−
=
λ
=
⎟
⎟
⎠
⎞
⎜
⎜
⎝
⎛
λ−λ
⎟
⎟
⎠
⎞
⎜
⎜
⎝
⎛
λ−
=
1
0
1
0
X
X
)1(
)1(
)(
n
i
i
n
n
i
i
c
i
n
c
n
R
R
R
R
nD
⎟
⎠
⎞
⎜
⎝
⎛
⋅⋅⋅++λ
=
−
XX
1
X
1
1
1
1
+
nn
(14)
where
c
R
R
)(1X λ-=
.
Since
, , and ,
then 0 < X < 1. So, the D(n) becomes a monotonous
decrease for n. Therefore, the probability
of (13) is a monotonous increase
function for n. Lemma 1-1 is proved.
10 <λ≤ 110 ≤λ−<
RR
c
>
)|(
ncn
SEP
Remark: Lemma 1-1 indicates that, if the
number of times of the unfitting n increases, the
probability that the structural change has occurred
increases. This meets our intuition clearly.
Lemma 1-2.
The conditional probability
)|(
1 nn
SSP
+
is a
decrease function for n.
Proof. Based on the model in Fig. 3, we have
))|(1)(1()|(
1 ncnnn
SEPRSSP −−=
+
)|()1(
ncnc
SEPR−+
(15)
The first term in the RHS of (15) shows the
probability that the fitting occurs for the (n+1)-th
time observed data when the structure is unchanged.
The second term shows the probability that the
fitting occurs for the (n+1)-th time observed data
when the structure changed.
From (15), we have
))(|(1)|(
1 cncnnn
RRSEPRSSP −+−=
+
(16)
By Lemma 1-2,
is an increase
function, and
, therefore,
)|(
ncn
SEP
c
RR <
)|(
1 nn
SSP
+
is a
decrease function for n. Lemma 1-2 is proved.
Remark: Lemma 1-2 indicates that, if the
number of times of continuous unfitting increases,
the probability of the fitting for the next observed
data after those continuous unfitting decreases. This
is intuitively clear, because, by Lemma 1-1, the
probability of the structural change increases if the
number of times of the continuous unfitting
increases.
REFERENCES
R.Jana and S.Dey, 2000. Change detection in Teletraffic
Models. IEEE Trans. Signal Processing, vol.48, No.3,
pp.846-853, March 2000.
R.M.Gagliardi and I.S.Reed, 1965. On the Sequential
detection of Emerging Targets. IEEE Trans.
Information Theory, vol.6, pp.260-263, April 1965.
R.J.Di Francesco, 1990. Real-Time Speech segmentation
Using Pitch and Convexity Jump Models. IEEE Trans.
Acoustics, Speech, Signal Processing, vol.38, pp.741-
748, May 1990.
A.S.Willsky, 1996. A survey of design methods for failure
detection in dynamic systems. Automatica, Vol.12. pp.
601-611, Sept. 1996.
D.Kauame and J.P.remenieras et al., 1996. Effect of The
Compensation In Abrupt Model Change Detection
Problem.
Proc. of IEEE Digital Signal Processing
Workshop, pp.311-314, Sept. 1996.
Chow,G.C., 1960. Tests of Equality Between Sets of
Coefficients in Two Linear Regressions.
Econometrica, Vol.28, No.3, pp.591-605, July 1960.
S.MacDougall, A.K.Nandi and R.Chapman, 1998.
Multisolution and hybrid Bayesian algorithms for
automatic detection of change points.
Proc. of IEEE
Visual Image Signal Processing, vol.145, No.4,
pp.280-286, August 1998.
V.V.Veeravalli and A.G.Tartakovsky, 2002. Asymptotics
of quickest change detection procedures under a
Bayesian criterion.
ITW2002, pp.100-103, Bangalore,
India, Oct. 2002.
E.S.Page, 1954. Continuous inspection schemes.
Biometrika, Vol. 41, pp.100-115, 1954.
C.Han,P.k.Willet and D.A.Abraham, 1999. Some methods
to evaluate the performance of Page's test as used to
detect transient signals. IEEE Trans. Signal
processing, vol.47, No.8, pp.2112-2127, August 1999.
S.D.Blostein, 1991. Quickest detection of a time-varying
change in distribution. IEEE Trans. Information
Theory, vol.37, No.4, pp.1116-1122, July 1991.
Y.Liu, S.D.Blostein, 1994. Quickest detection of an abrupt
change in a random sequence with finite change-time.
IEEE Trans. Information Theory, vol.40, No.6,
pp.1985-1993, Nov. 1994.
M.Basseville and I.V.Nikiforov, 1993. Detection and
Application, Prentice Hall, Inc., April 1993.
M.Basseville, 1988.Detecting Changes in signals and
systems– A survey. Automatica,Vol.24, pp.309-
326, 1988.
N.R.Draper, H.Smith, 1996. Applied Regression Analysis,
John Wiley & Sons Inc, 1966.
NEW METHOD FOR STRUCTURAL CHANGE DETECTION OF TIME SERIES AS AN OPTIMAL STOPPING
PROBLEM
31