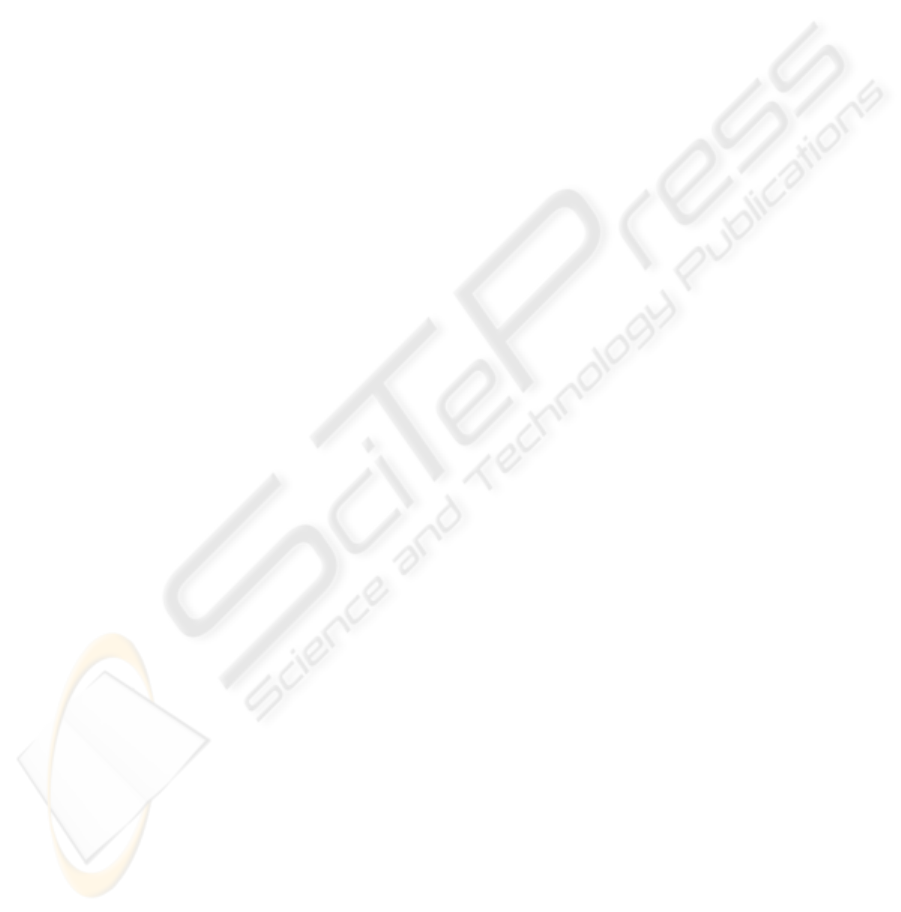
conditions. Flood routing techniques have been developed mainly for the study of
floods traveling through channels, reservoirs and lakes. The technique determines the
time and magnitude of flood peaks occurring at various points along a river as the
flood travels downstream. Presently, flood routing is employed for a wide variety of
problems associated with water use. Some of these include; (i) evaluating past floods
for which records are incomplete; (ii) determining hydrograph of channel flow from
hypothetical design floods on tributaries and upstream reaches of the main channels:
(iii) forecasting floods along the main course of a river, by use of predicted
hydrographs at key points in the drainage network; (iv) determining hydrographs
modified by reservoir storage; and (v) studying the effects of water resources
development on the downstream flow conditions.
Flood routing is carried out by solving the unsteady flow equations. These are
partial differential equations and for solution of these equations, many simplifying
assumptions such as uniform roughness, constant channel cross-section with constant
bed slope etc., are made. Without these assumptions, the relationship between
variables becomes more complex and the method of solution needs more data to
describe the channel and wave conditions. Faced with the limitations of time and
economy in the preparation of data, one has to resort to approximate methods of
solution. Along with the labor required for solving the equations, a tremendous work
is required for identification of some of the parameters required, (see for example
Ghumman A.R. 1996).
An effort is made in this study to use a neural networking technique and prepare a
flood-warning scheme for rivers in Pakistan. A reach of River Chenab between
Marala and Khanki is selected for this as it receives flood nearly every year for the
last so many years.
2 Neural Networking Models
Artificial Neural networks are increasingly used in predicting and forecasting water
resource variables (Nash, J.E. and Sutcliffe (1970), French et, M.N. (1992), Zhu, M.L.
and Fujita, M. (1994), Dawson C. W. & Wilby R. L.(2001), Yi-Ming Kuo (2003)).
Hydrologic models can be divided into three broad categories, namely: Physical
distributed models, lumped conceptual models and black box models.
Physical based distributed models require excessive field data whereas in case of
lumped conceptual models, large number of parameters and subsequent difficulty in
calibration is involved. Both of these models are used where detailed understanding of
the hydraulic phenomenon is necessary. Black box models do not contribute much in
enhancing the understanding of hydrological and hydraulic phenomena; nevertheless
in operational hydrology and hydraulic Engineering their usefulness is of utmost
importance. Neural Networking models can be considered as black box models. These
are easy to use and have comparatively less data requirements. This is the reason why
they are becoming popular and are recently being used in the field of Water
10