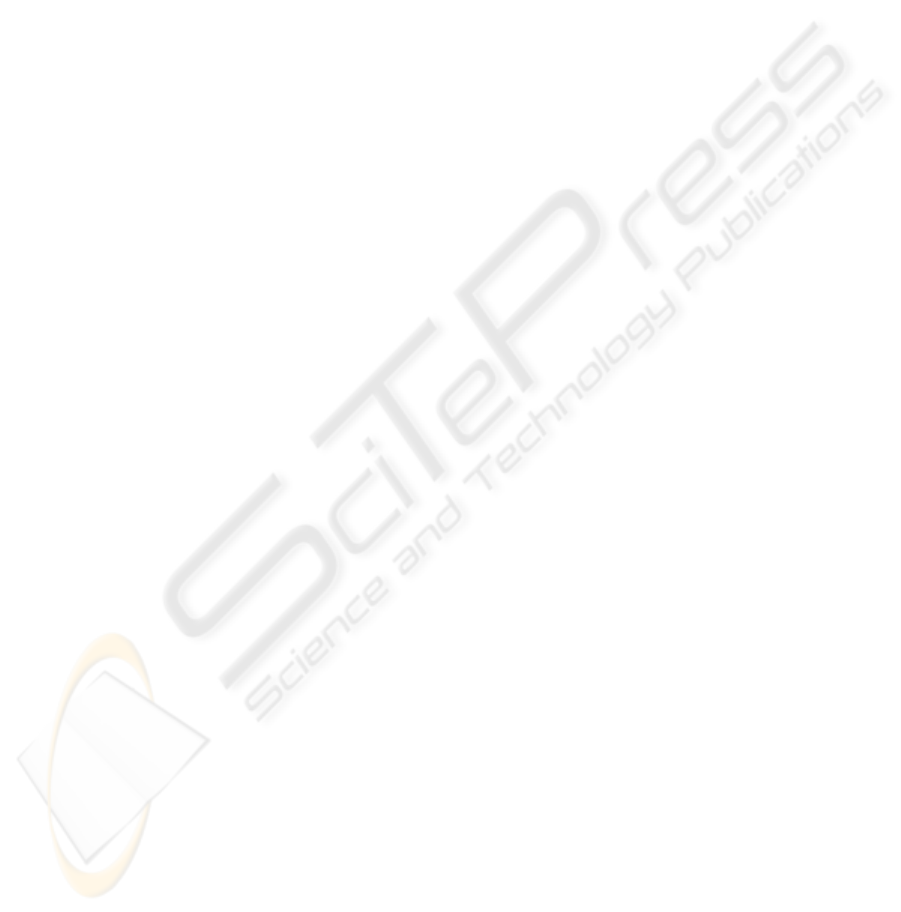
6 Conclusion and Future Work
We have shown the application of two types of artificial neural networks for signature
verification. It can be seen (Tab. 1) that classification of genuine and forgery signatures
is reliable if the parameters of networks are trained by a sufficient number of dutifully
prepared training patterns. The achieved FRR and FAR are fully comparable with the
results obtained by standard statistical or structural methods, the wide range testing of
both types of ANNs will be carried out in the future.
In our future work, we plan to focus on the following tasks which could improve
the results of verification process:
– including the new valuable features to the feature vector describing signature,
– optimal setting of the parameters of the input and interface layer of ART-2,
– optimal setting of the thresholds l
t
and Q
t
of SOFM verifier,
– checking the possibility of the application of other neural networks (supervised or
unsupervised learned).
References
1. Mautner, P., Matousek, V., Rohlik, O., Kempf, J.: Signature Verification Using Unsupervised
Learned Neural Networks, Proceeding of the 1st IAPR-TC3 Workshop on Artificial Neural
Network in Pattern Recognition, Florence, Italy, 2003
2. Plamondon, R., Lorrette, G.: Automatic Signature Verifications and Writer Identification-The
State of the ART, Pattern Recognition, Vol 22, No. 2, 1989
3. Koivisto, L.: Quinquennial Report 1994-1998, Neural Network Research Centre and Labo-
ratory of Computer and Information science,FIN-02015, Finland, 1999
4. Fiesler, E., Beale, R.:Handbook of Neural computation, Oxford University Press, New York,
Oxford, 1997
5. Rohlik, O., et al.:A New Approach to Signature Verification: Digital Data Acquisition Pen ,
Neural Network World, Vol. 11, No. 5, pp. 493-501,2001
6. Pitnerr. S, Kamarthi, S. V.: Feature Extraction from Wavelet coefficients for Pattern Recog-
nition Tasks, IEEE Transactions on PAMI, Vol. 21, No. 1, 1999
7. Fausett, L.: Fundamentals of Neural Networks, Prentice-Hall, New Jersey, 1994.
8. Carpenter, G. A., Grossberg, S.: ART-2: Self-organization of Stable Category Recognition
Codes for Analog Input Patterns, Applied Optics, No. 26, pp. 4919-4930, 1987
9. Plamondon, R.: Progress in Automatic Signature Verification, World Scientific Publishing
Co. Pte. Ltd., Singapore, 1994
10. Tseng, L. Y., Huang, T. H.: An on-line Chinese Verification Scheme Based on the ART-1
Neural Network, in Int. Joint Conf. on Neural Networks, Baltimore, Maryland, Jun. 1992, pp
624-630
11. I-pen producer homepage,
http://www.inductum.com/
12. OTM technology overview
http://www.omtech.com/
13. E-pen documentation
http://www.mediaforte.com.sg/products/graphic/epen/
14. N-Scribe product documentation
http://www.n-scribe.com/
48