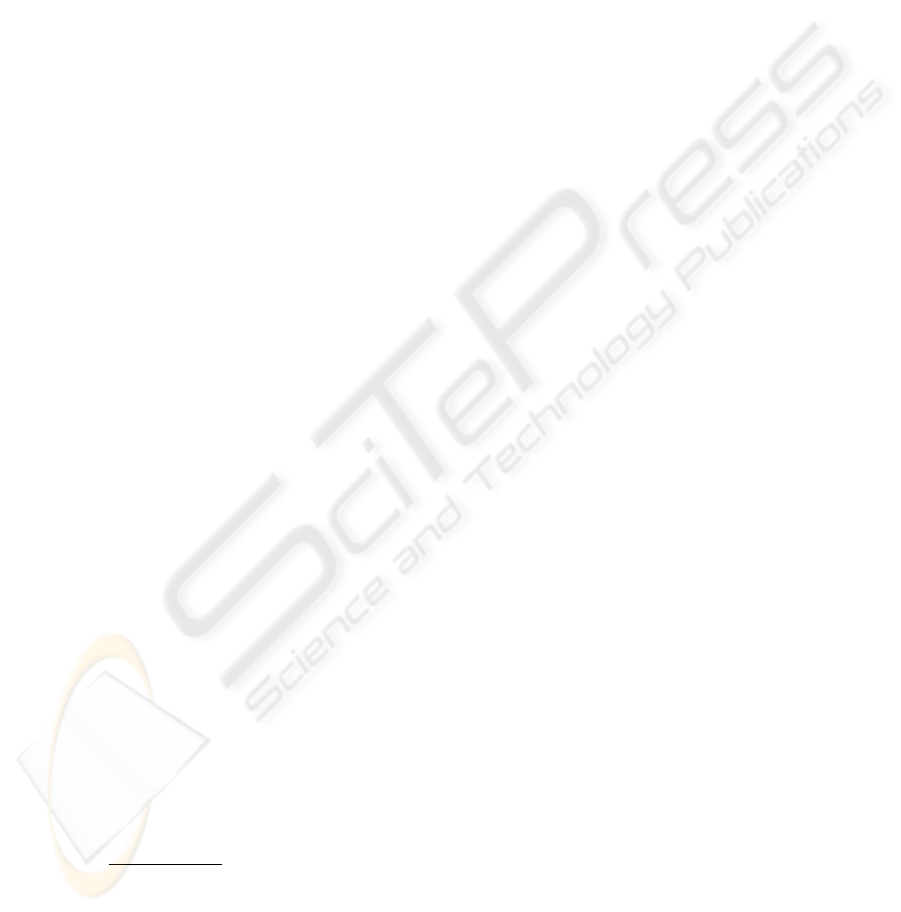
On the Capability of Neural Networks to Approximate
the Neyman-Pearson Detector. A Theoretical Study
P. Jarabo-Amores
?
, R. Gil-Pita, M. Rosa-Zurera, and F. L
´
opez-Ferreras
Departamento de Teor
´
ıa de la Se
˜
nal y Comunicaciones,
Escuela Polit
´
ecnica Superior, Universidad de Alcal
´
a
Ctra. Madrid-Barcelona, km. 33.600, 28805, Alcal
´
a de Henares - Madrid (SPAIN).
Abstract. In this paper, the application of neural networks for approximating
the Neyman-Pearson detector is considered. We propose a strategy to identify
the training parameters that can be controlled for reducing the effect of approx-
imation errors over the performance of the neural network based detector. The
function approximated by a neural network trained using the mean squared-error
criterion is deduced, without imposing any restriction on the prior probabilities
of the clases and on the desired outputs selected for training, proving that these
parameters play an important role in controlling the sensibility of the neural net-
work detector performance to approximation errors. Another important parameter
is the signal-to-noise ratio selected for training. The proposed strategy allows to
determine its best value, when the statistical properties of the feature vectors are
known. As an example, the detection of gaussian signals in gaussian interference
is considered.
1 Introduction
The objective of this paper is to study the capability of neural networks to approximate
a Neyman-Pearson detector. This detector maximices the probability of detection (P
D
),
while maintaining the probability of false alarm (P
F A
) lower than or equal to a spec-
ified value. The characteristics of such a detector are reflected in its ROC (Receiver
Operating Characteristic) curve, that relates P
D
to P
F A
[1].
Ruck et al. [2], and Wan [3], demonstrated that a neural network can be used to
approximate the optimum bayessian classifier when trained using the mean squared-
error criterion.
In previous works, neural networks have been proposed for approximating the
Neyman-Pearson detector in different environments [4][5]. These works highlighted the
strong dependence of the neural network-based detector performance on the signal-to-
noise ratio selected for training (TSNR). They also observed that the difference between
the neural detector performance and the Neyman-Pearson detector one, depends on the
desired P
F A
, and so, on the corresponding detection threshold.
Recently, some attempts to reduce the dependence of the neural detector perfor-
mance on TSNR have been carried out [6], based on the use of a complex pre-processing
?
This work has been supported by the “Consejer
´
ıa de Educaci
´
on de la Comunidad de Madrid”
(SPAIN), under Project 07T/0036/2003 1
Jarabo-Amores P., Gil-Pita R., Rosa-Zurera M. and López-Ferreras F. (2004).
On the Capability of Neural Networks to Approximate the Neyman-Pearson Detector - A Theoretical Study.
In Proceedings of the First International Workshop on Artificial Neural Networks: Data Preparation Techniques and Application Development, pages
67-74
DOI: 10.5220/0001150100670074
Copyright
c
SciTePress