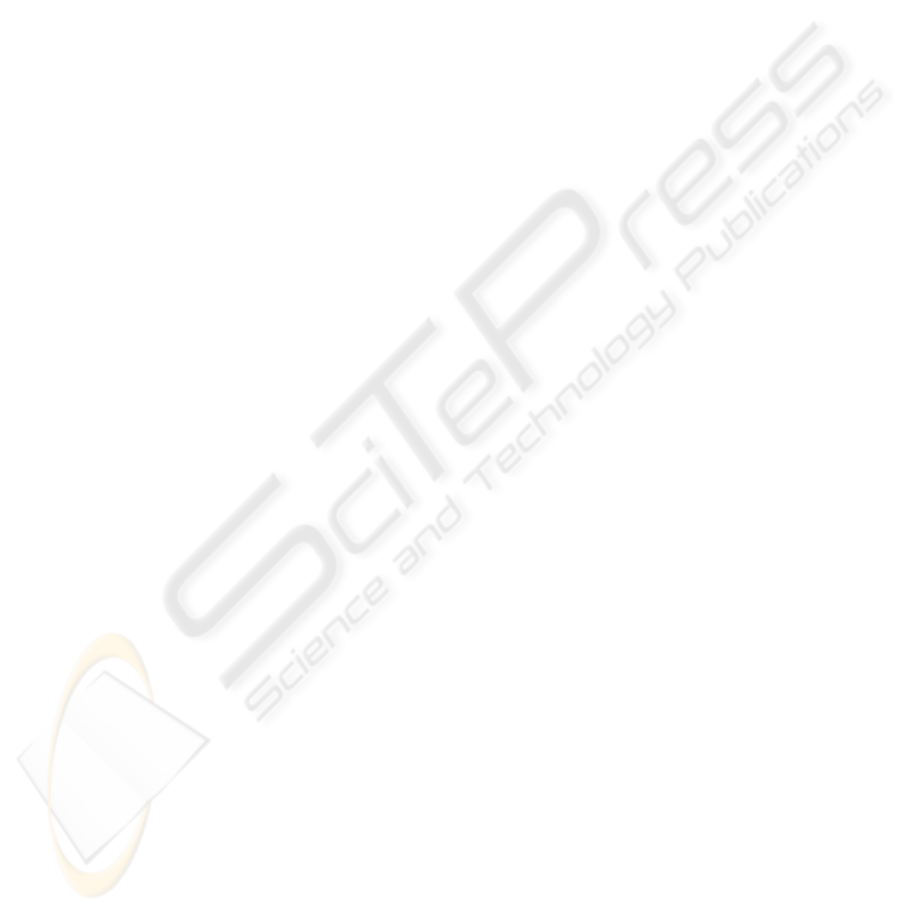
is much higher than any published result with
system condition number as high as nearly 390.
Furthermore, it is assumed that each tap weight
of the channel undergoes an independent
stationary stochastic process with each
parameter fluctuating around its nominal value
with a uniform probability distribution over the
interval
()
kkkk
hhhh %8,%8 +− , in
addition to the white noise disturbance of
variance 0.001 at the channel output. Mont
Carlo simulation experiment of 1000
independent trials is conducted to obtain an
ensemble-averaged learning curve. All adaptive
algorithms have shown a good robust
performance against the time varying, random
Gaussian impulse response coefficient
fluctuations specified above. The filtered-X
LMS with the optimal
2
H initialization has
been shown to have the fastest convergence
rate and best performance.
(5). A more severe non-stationary situation was
tested by abruptly increasing the channel
impulse response coefficient by 35% of its
nominal value. The conventional adaptive LMS
algorithm begins to diverge while the filtered-X
LMS algorithm with or without optimal
initialization still maintains a good robust
performance. The conventional RLS algorithm
has an acceptable performance, which matches
the observation that when the time variation of
the channel is not small, the RLS algorithm
will have a tracking advantage over the LMS
algorithm (R.D.Gitlin et al., 1992). The filtered-X
RLS algorithm has a better robust performance.
The performance improvement by using the
proposed techniques is significant and hence,
the effectiveness of the new method has been
verified.
5 CONCLUSIONS
The contributions of this paper are: we are compared
the LMS with DCT-LMS and RLS for adaptive
equalizer first, then we will be conducted on how to
speed up the convergence rate of LMS based
algorithm while keeping the increased in-line
computational burden as low as passible, we will
overcome the slow convergence problem while
keeping the simplicity of the LMS based algorithm,
and the H
2
Optimal initialization has been applied in
adaptive equalizer for communication systems.
There still exists many open problems. For
instance, the analysis of the stability margin of
the filtered-X LMS was conducted in a heuristic
manner. Can we extend this to a general case
such as a discrete time MIMO case? What about
the filtered-X RLS algorithm? Can we apply the
ideas to other adaptive equalization techniques
such as decision-feedback equalization, etc.? What
happens if we use the
∞
H optimal
initialization instead of the
2
H optimal
initialization? Another very active area of
equalization is wireless communication where the
phenomenon of fast multiple-path fading
(Rayleigh fading) is very challenging. As
indicated in the simulation, rapid and not so
small channel variations can cause the
conventional LMS algorithm to diverge. It will
be interesting and challenging, therefore, to
apply the new techniques presented here to those
areas in the future.
REFERENCES
R.D.Gitlin, J.F. Hayes, and S.B. Weinstein, Data
communication principles, Plenum Press, New York,
1992.
E.A. Lee and D.G. Messerschmitt, Digital
communication, Second Edition, Kluwer Academic
Publishers, 1994.
S. Haykin, Adaptive filter theory, Third Edition Edition,
Prentice Hall Information and System Sciences
Series, 1996.
David S. Bayard, "LTI representation of adaptive
systems with tap delay-line regressors under
sinusoidal excitation," "Necessary and sufficient
conditions for LTI representations of adaptive
systems with sinusoidal regressors," Proceedings of
the American Control Conference, Albuquer, New
Mexico, June 1997, pp. 1647-1651, pp. 1642-1646.
Steven L. Gay, "A fast converging, low complexity
adaptive filtering algorithm," Proceedings of 1993
IEEE Workshop on Applications of Signal
Processing to Audio and Acoustics, New Paltz, NY,
USA, 1993, pp.4-7.
S.Elliott and P. Nelson. "Active noise control," IEEE
Signal Processing Magazine, Oct. 1993.
Markus Rupp and Ali H. Sayed, "Robust FXLMS
algorithms with improved convergence performance,"
IEEE Trans. on Speech, Audio Processing, vol.6,
no.1, Jan.1998, pp.78-85.
E.A. Wan, Adjoint LMS: an efficient alternative to the
Filtered-X LMS and multiple error LMS algorithms.
IEEE International Conference on Acoustics, Speech,
ICETE 2004 - GLOBAL COMMUNICATION INFORMATION SYSTEMS AND SERVICES
134