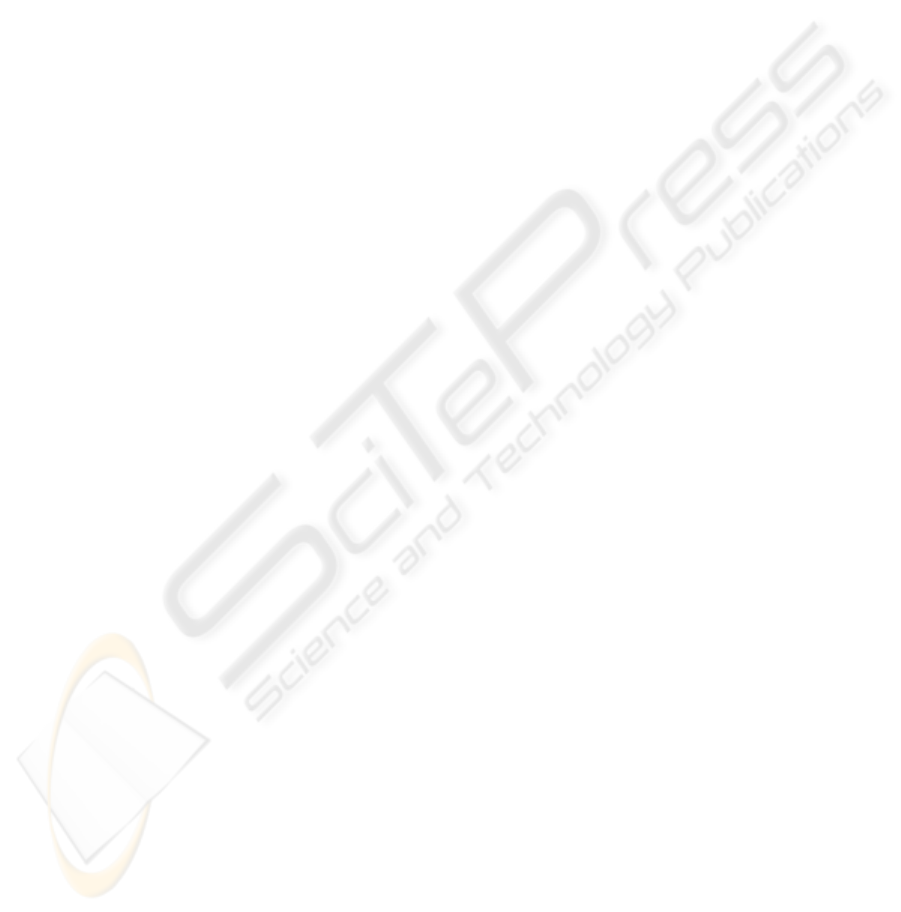
M
3
RT µ-IEPM mechanism replaces the PE or point-
estimate approach in the predecessor. The M
3
RT
predicts the mean, namely, M
i
of the data
distribution being worked on. It differs from the PE
computation by the following: a) it is faster,
smoother and more accurate, b) it works with a fixed
F (flush limit) number of data samples, and c) it is
integrative with the M
i-1
(predicted mean in the last
cycle) feedback but the PE has no feedback loop.
The stability of the M
i
convergence, however,
reduces the sensitivity of the popularity ratio that is
required for producing accurate, responsive dynamic
cache size tuning. With the aim to improve this
sensitivity the integrative equation (3.6) for σ
i
is
proposed. For E-MACSC the calculation of the
popularity ratio is based on equation (3.7) instead of
using M
i
directly. The preliminary simulation results
confirm that the E-MACSC is far more efficacious
in maintaining the given hit ratio than the MACSC
approach. In addition the hit ratio by the E-MACSC
tends to be higher than the given value. This leads
to: shorter information retrieval RTT, less timeouts
and thus retransmissions by the clients, more
network backbone bandwidth freed for public
sharing, and better system throughput in general. In
contrast the hit ratio by the MACSC tuner oscillates
and can be much lower than the given value. The
analysis of the preliminary E-MACSC experience
confirms that its efficacy depends on a few factors
though, namely, the α value, the choice of the flush
limit, and the average IAT of the data samples.
Therefore, the planned activity for the next phase in
the research is to study the impacts of these factors
thoroughly. Different possible ways to neutralize the
negative effect of some of these factors on dynamic
cache tuning performance will be explored and
scrutinized.
ACKNOWLEDGEMENTS
The authors thank the Hong Kong Polytechnic
University and the Department of Computing for the
research funding: H-JZ91
REFERENCES
Aggarwal, C., Wolf, J. L. and Philip S. Yu, 1999. Caching
on the Word Wide Web. In IEEE Transactions on
Knowledge and Data Engineering, 11(1)
Allan, K. Y. Wong, May, T. W. Ip and Richard, S. L. Wu,
2003. A Novel Dynamic Cache Size Adjustment
Approach for better Retrieval Performance over the
Internet, Journal of Computer Communications, 26(14)
Allan, K.Y. Wong, Tharam, S. Dillon, Wilfred, W.K. Lin
and May, T.W. Ip, 2001. M2RT: A Tool Developed
for Predicting the Mean Message Response Time for
Internet Channels, Computer Networks, Vol. 36
Breslau, L., Cao, P., Li, F., Phillips, G. and Shenker, S.,
1999. Web Caching and Zipf-like Distributions:
Evidence and Implications, In INFOCOM’99, Vol. 1
Chis, J. A., 1992. Introduction to Simulation and
Modeling - GPSS/PC, Prentice Hall
Cottrel, L., Zekauskas, M., Uijterwaal, H. and McGregor,
T., 1999. Comparison of Some Internet Active End-to-
End Performance Measurement Projects,
http://www.slac.stanford.edu/comp/net/wan-
mon/iepm-cf.html
Jin Shudong and Bestavros, A., 2000. Popularity-Aware
Greedy Dual-Size Web Proxy Caching Algorithms,
Proc. of the Int’l Conf. on Distributed Computing
Systems
Paxson, V., Floyd, S., 1995. Wide area traffic: The Failure
of Poisson Modeling, In IEEE/ACM Transactions on
Networking, 3(3)
Richard, S.L Wu, May, T.W. Ip and Allan, K.Y. Wong,
2003. LDC-CM: A Novel Model for Dynamic Cache
Size Adjustment, In Proceeding of the International
Conference on Internet Computing, Vol. 2, Las Vegas
USA
Stefan Podlipnig and Laszlo Böszörmenyi, 2003. A
Survey of Web Cache Replacement Strategies, In
ACM Compputing Surveys, Vol. 35, NO. 4, 374-398
Intel Vtune, Retrieved from
http://developer.intel.com/software/products/vtune/
Wessels, D., 2001. Web Caching, O’Reilly & Associates
Inc.
Zipf Curves and Website Popularity, Retrieved from
http://www.useit.com/alertbox/zipf.html
E-MACSC: A NOVEL DYNAMIC CACHE TUNING TECHNIQUE TO MAINTAIN THE HIT RATIO PRESCRIBED
BY THE USER IN INTERNET APPLICATIONS
159