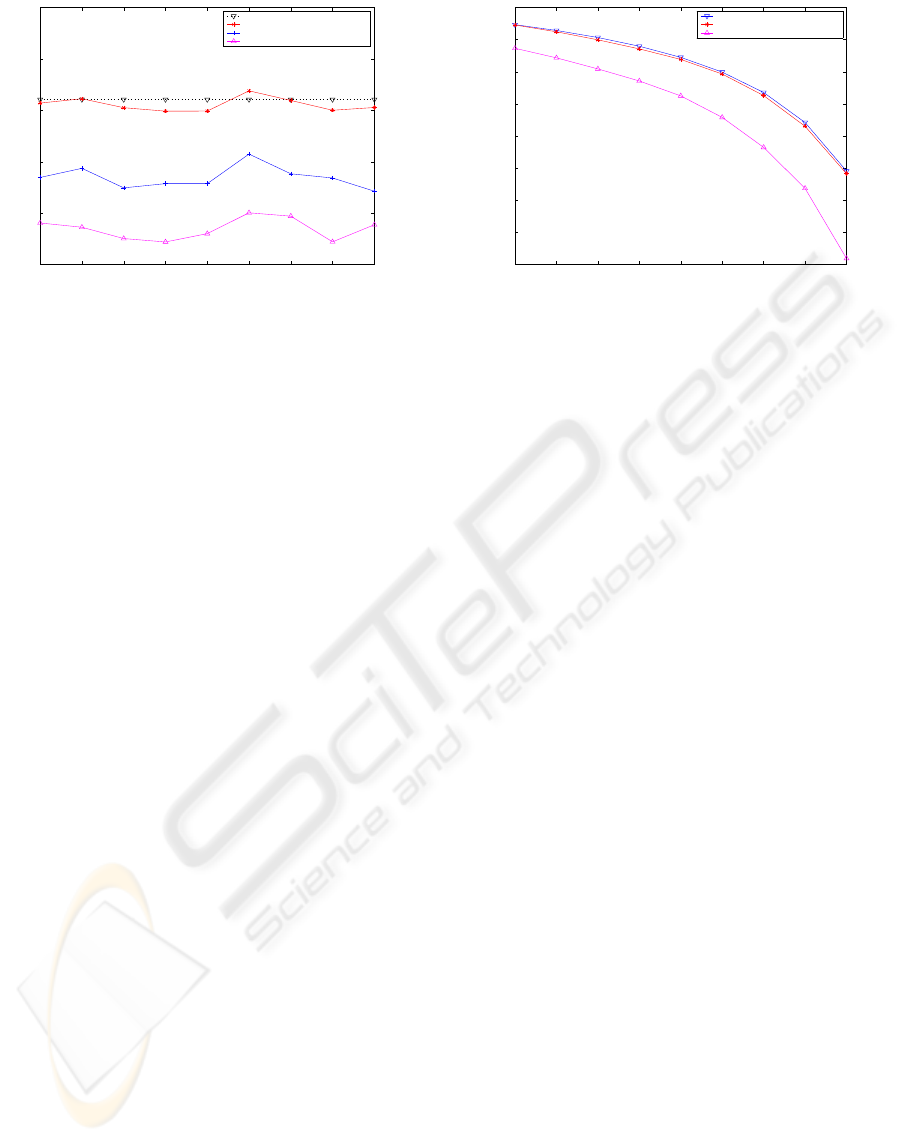
100 200 300 400 500 600 700 800 900
150
180
210
240
270
300
time (s)
revenue
Analytic
Simulated under optimal weights
Simulated under given weights
Simulated under proportional weights
Figure 5: Revenue comparison as function of time, for the
case, load factor ρ = 1 and b
1
= 200, k
1
=10000, b
2
= 150,
k
2
= 5000, b
3
= 80, k
3
= 2000.
that measurement period. Unless stated otherwise, we
shall hereafter refer to the latter as revenue. It is ob-
served that the largest revenue is achieved under our
optimal weights compared with those achieved un-
der the proportional and given weights and it is quite
close to the analytic value of maximum revenue by
Eq. (15). Since the parameters used in Eq. (15) are
constant in this case, the analytic value remains con-
stant; whereas, for the mean delay of packets by simu-
lations is variable, the simulated revenue varies along
with the time. Fig. 5 shows that the revenue obtained
under our optimal weights is very close to the ana-
lytic value, which demonstrates the effectiveness of
our approach for revenue maximization.
Next we examine the performance of our revenue-
maximizing approach for the case that the same pric-
ing functions are used and different traffic intensi-
ties are fed into the packet scheduler. Fig. 6 shows
the simulation results, where the x-axis represents the
load factor and the y-axis represents the revenue.
First focusing on our revenue-maximizing ap-
proach, we can see that the revenues obtained under
our optimal weights are extremely close to those of
analytic forms for light and medium loads, and both
decrease along with the load factor. This is as ex-
pected because the increase of the traffic load fed into
the scheduler incurs the increase of the mean delay
and thus the decrease of the obtained revenue. At
heavier loads both curves start to level off sharper
as the penalties start to grow faster. Compared with
our approach, the proportional assignment scheme
achieves less revenues at all traffic loads. And it de-
creases even sharper for heavier load as the penal-
ties incurred under the proportional weights are much
larger than the ones under our optimal weights for the
same load and grow faster.
1 1.5 2 2.5 3 3.5 4 4.5 5
−500
−400
−300
−200
−100
0
100
200
300
Load factor
revenue
Analytic
Simulated under optimal weights
Simulated under proportional weights
Figure 6: Revenue comparison as function of load factor ρ,
for the case, b
1
= 200, k
1
=10000, b
2
= 150, k
2
= 5000,
b
3
= 80, k
3
= 2000.
4.2 The second set of simulations
In the second set of simulations, the same simulations
are made for three different linear pricing functions:
b
1
= 200, k
1
= 5000, for gold class, b
2
= 120, k
2
=
2000, for silver class, and b
3
= 40, k
3
= 500, for
bronze class, to evaluate the performance robustness
of our approach for revenue maximization. Figs. 7
and 8 present the simulation results.
It is observed in Fig. 7 that the revenue obtained
under our optimal weights is the largest and it is also
close to the analytic value by Eq. (15). Since the slope
k
i
of class i in this case is less than the one in the first
set of simulations, the revenue obtained from class i
will decrease more slowly along with the increase of
mean delay in this case, leading to the revenue curves
in Fig. 8 level off smoother for the same load com-
pared with the ones in Fig. 6. Similarly, the largest
revenue is obtained under our optimal weights for all
traffic loads and it is very close to the curve of analytic
maximum revenue. Therefore, it is demonstrated that
our revenue-maximizing approach is effective for any
linear pricing functions.
5 CONCLUSIONS
In this paper, we explored the problem of max-
imizing revenues under multi-class Service-Level-
Agreements. In particular, we considered the opti-
mization problem of maximizing the revenue attained
in a network node under linear pricing scenario. A
revenue-aware scheduling approach was introduced,
which has the closed-form solution to the optimal
weights for revenue maximization derived from rev-
enue target function by Lagrangian optimization ap-
proach. The simulations demonstrated the revenue
maximization ability of our approach.
ICETE 2004 - SECURITY AND RELIABILITY IN INFORMATION SYSTEMS AND NETWORKS
138