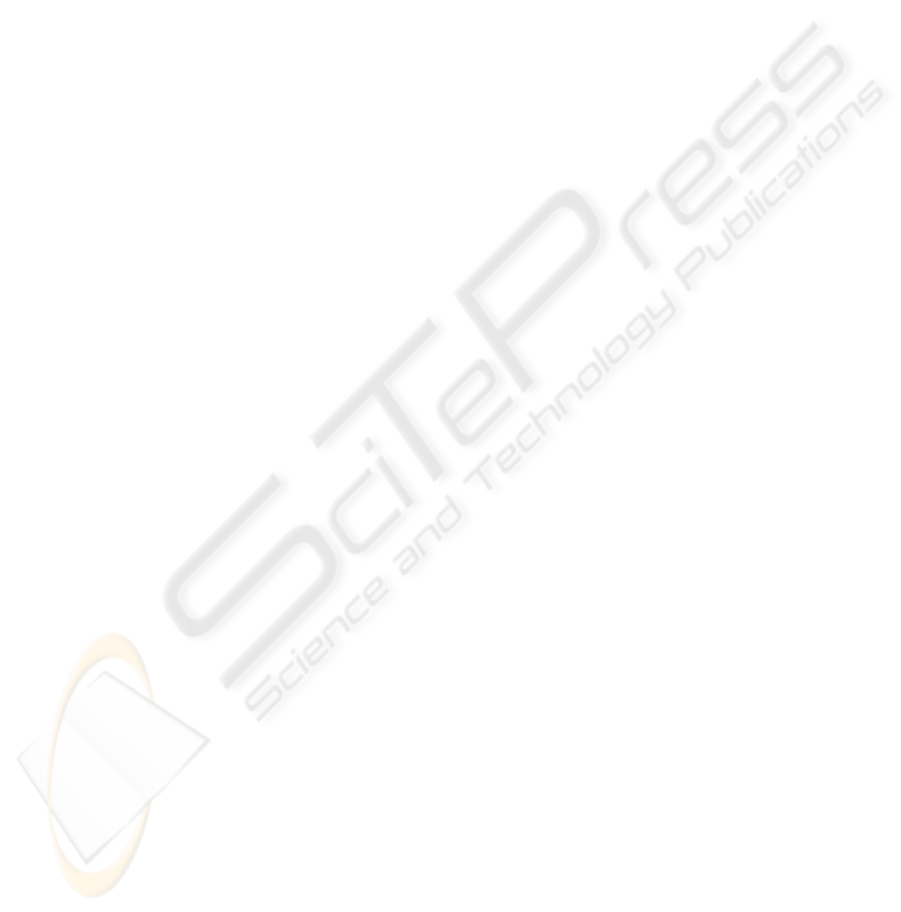
for the application of the model to be worthwhile the
probability values for its nodes must be meaningful.
A comparative approach between these probability
values and the assessment of each e-commerce
system must be formulated. In other words, when
the model estimates the probability values of the
quality characteristics of an e-commerce system, one
must be able to classify this system and ascertain the
specific fields that need to be improved. The paper
provides the boundaries and the scales of these
values that were concluded from experimental
measurements to a number of e-commerce systems.
As a result, it provides a non-subjective way of
characterizing an e-commerce system according to
the quality characteristics with which this method is
concerned. In this way, the use of this model can
easily lead to conclusions and determine specific
corrective actions needed to be set in order to
improve the quality of the system.
In this case study a number of e-commerce
systems were measured following the proposed
method. In detail, the data of this method were based
on the assessment of 120 different Business to
Consumer (B2C) e-commerce systems. The
selection of these sites was a representative list
randomly sampled from the entire list of the Greek
and international e-commerce systems, which were
available the day of this study. For each of these
systems the aforementioned model was used, by
defining the values in its child nodes. In this way,
the probability values for all the intermediate nodes
were estimated.
After collecting the measurement data, the next
step was the analysis of the results. Firstly, the
normal distribution of the data for all the quality
characteristics and sub-characteristics was checked
in order to ensure their validity. As previously
mentioned, the aim of this research was the
determination of the possible boundaries and scales
of the measurement data, so as to define in an easy
and non-subjective way which quality characteristics
of an e-commerce system have high or low scores.
In other words, the measurement data must be
grouped in different clusters that characterize how
good or bad a system is. In order to define these
clusters, three alternative approaches could be
followed: a) setting a priori the values that define the
boundaries of the clusters, even before conducting
the experimental measurements to the number of e-
commerce systems used in this research, b) setting
these values with the use of percentages of the
measurement results and c) estimating these values
by judging from the measurement results themselves
and their possible distribution to clusters.
Although all of the alternative approaches of
analyzing the data are acceptable, the third one was
chosen, since it provides more representative rates.
Moreover, the analysis of the data showed that they
were clearly distributed in different clusters. In this
way the desired boundaries of the different scales for
each quality characteristic were defined with more
accuracy. Following this approach in the analysis,
these boundaries can be defined regardless of the
number of the e-commerce systems that were
measured in this research.
The benefits of this analysis are noticeable. First
of all, it provides an easy and non-subjective way to
rank an e-commerce system according not only to
the overall quality, but for each quality characteristic
or sub-characteristic as well. In this way, it
obviously shows which corrective actions may be
followed to improve the quality of the system.
Moreover, using this analysis, developers are able to
determine the e-commerce characteristics on which
they must focus, in order to achieve a desired value
for the quality of the system that they develop.
4 DATA ANALYSIS
By applying the model at 120 e-commerce systems,
measurement results were collected for the overall
quality, the quality characteristics and sub-
characteristics of the presented method. The
measurement results, as presented hereinafter,
correspond with the node probability values offered
from the method’s tool for the state “Good”. The
entire set of probability values for all states can be
found in the web site of the Software Quality
Research Group of the Hellenic Open University
(SQRG-HOU, 2004).
The results for the overall quality were
distributed normally and are presented in Table 1
(Normality test Kolmogorov-Smirnov Significance
level(Nom. test K-S s.l.)= 0.1; Mean (m)=0.56;
Standard deviation(Std)= 0.24), and they were
distinguished in 3 categories A, B, C.
• Category A includes a small number of
measurement values because in this category
were included the e-commerce systems that
satisfy strict criteria for the overall quality, the
quality characteristics and sub-characteristics.
And as the nature of the e-commerce system is
to give emphasis to some of the above quality
characteristics it is extremely difficult to
achieve high measurement results in all sub-
characteristics.
• Category B comprises e-commerce systems that
satisfy a number of criteria for the overall
quality. Although these systems appear to have
an acceptable probability value for quality,
corrective actions can be followed in order to
ICETE 2004 - GLOBAL COMMUNICATION INFORMATION SYSTEMS AND SERVICES
28