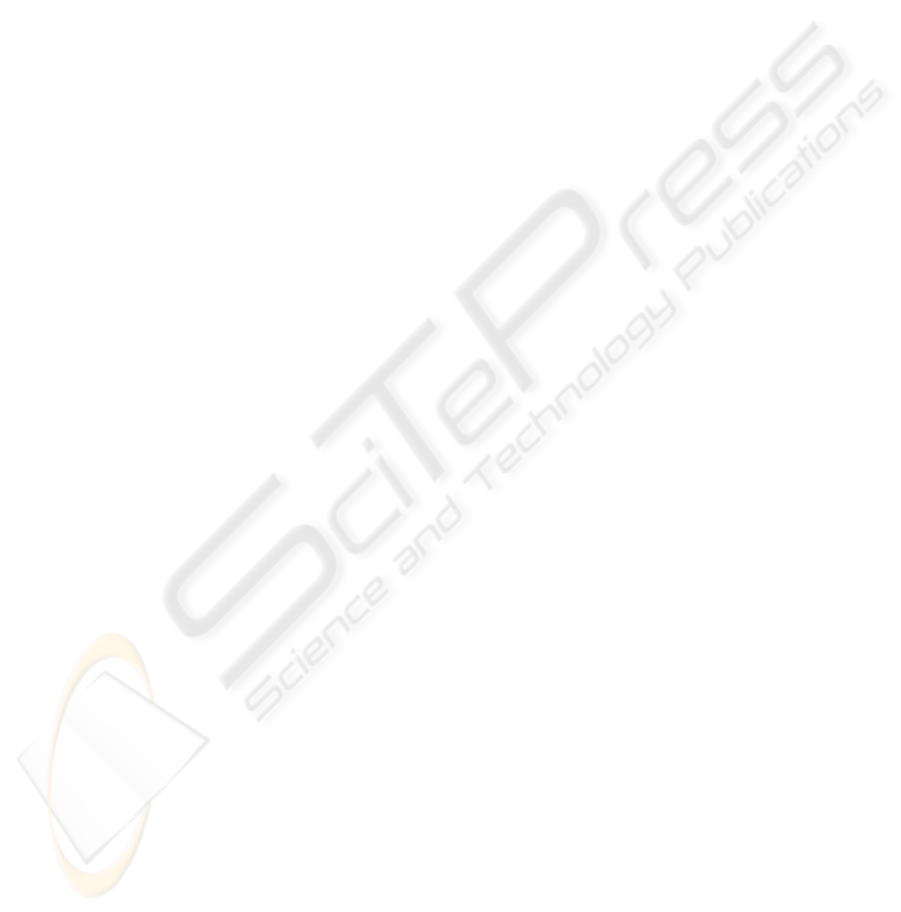
2.3 Intuitive data quality
The intuitive definition of data quality is "fitness for
use" (Bruckner & Schiefer 2000;Wang & Strong
1996a) for the "data consumer" (Strong et al. 1997).
This demonstrates relativity and subjectivity. As
what can be interpreted as a reaction to the relativity
the intuitive approach to data quality are often
primarily focused on metrics and figures: firstly,
metrics to describe the extension of the data quality
problem; secondly, metrics of a guess or estimate of
the (financial) effect of poor data quality; and lastly
the proportion of errors in the data that are causing
these problems.
The relativity of data quality is important as the
rationale for the establishment of the data warehouse
exactly is to bring the same data into many different
contexts (applications) utilized by many different
users (Tayi & Ballou 1998).
The weakness of the intuitive approach is that
there is no stated and clear definition of the concept
"data quality"; however some quality dimensions are
identified: accuracy, currentness, completeness, and
consistency (Fox et al. 1994).
2.4 Empirical data quality
The user perspective is underlying the intuitive
approach but is made explicit when Wang and
Strong (1996b) are pursuing a methodological well-
based exploratory empirical study of data quality
from a user perspective by applying marketing
methodology and viewing data as a product and the
user as a consumer. The obtained many quality
descriptors were processed by use of factor analysis
and grouped into four target categories: Intrinsic,
Contextual, Representational, and Accessability.
The concept of dimensions implies
unsubstitutability. This is demonstrated by the
conspicuous ineptness of assertions like "The data
are absolutely fitting for the task, but they are not
accessible", or "The data arrived in time, but they
are impossible to understand". All dimensions have
to be present – and can be so in varying degrees - or
the data will be "unfit for use".
2.5 Ontological data quality
The structure and categories within the area of data
quality are not guaranteed to arise from the intuitive
or the empirical approach. A theoretical approach
from a systems-design viewpoint is done by Wand
and Wang (1996) who build their argumentation on
the view that the information system (IS) delivers a
representation of the real world system (RW). From
the information system the user makes an inferred
interpretation of the real world, but is also capable of
making a direct observation of the real world. The
two views of the real world can lead to deficiencies
of data and "inconformity" between the two views.
The mapping between the information system and
the real world system leads to three categories of
defectiveness: Incomplete, Ambiguous, and
Meaningless. In its simple forms the extremes
implies that the RW has states not found in the IS
(incomplete) or the IS has states not existing in the
RW (meaningless). Ambiguity arises when a state in
the IS is covering more than one state in the RW.
Ambiguity precludes the inverse mapping from the
information system to the real world.
3 QUALITY DECISIONS
With the determination of both the empirical and the
theoretical developed dimensions it is fruitful to
return to the original starting point that data quality
should improve our acting. "A good decision is an
action we take that is logically consistent with the
alternatives we perceive, the information we have,
and the preferences we feel" (Howard 1988).
The dimensions of data quality are in the
ontological approach deducted to data being
incomplete, ambiguous, and meaningless while the
empirical findings isolated the groups of intrinsic,
contextual, representational, and accessible.
The data warehouse is a collection of data for
use in many applications and by many users. The
fact that most of these applications and users are
unknown when the system is designed – as well as
when data are extracted-transformed-loaded into the
data warehouse - accentuates that the development
of the data warehouse must assure extreme
flexibility to accommodate changes. The quality of
data is embedded not in the data itself, and not in the
system, but in the users use of data: "what may be
considered good data in one case (for a specific
application or user) may not be sufficient in another
case" (Wand & Wang 1996).
3.1 Incrementing quality by use
On the other hand the proposition in this paper is
that data quality is balanced. It is neither objective
nor solely a subjective undertaking. Enhancements
INCREMENTAL DATA QUALITY IN THE DATA WAREHOUSE
635