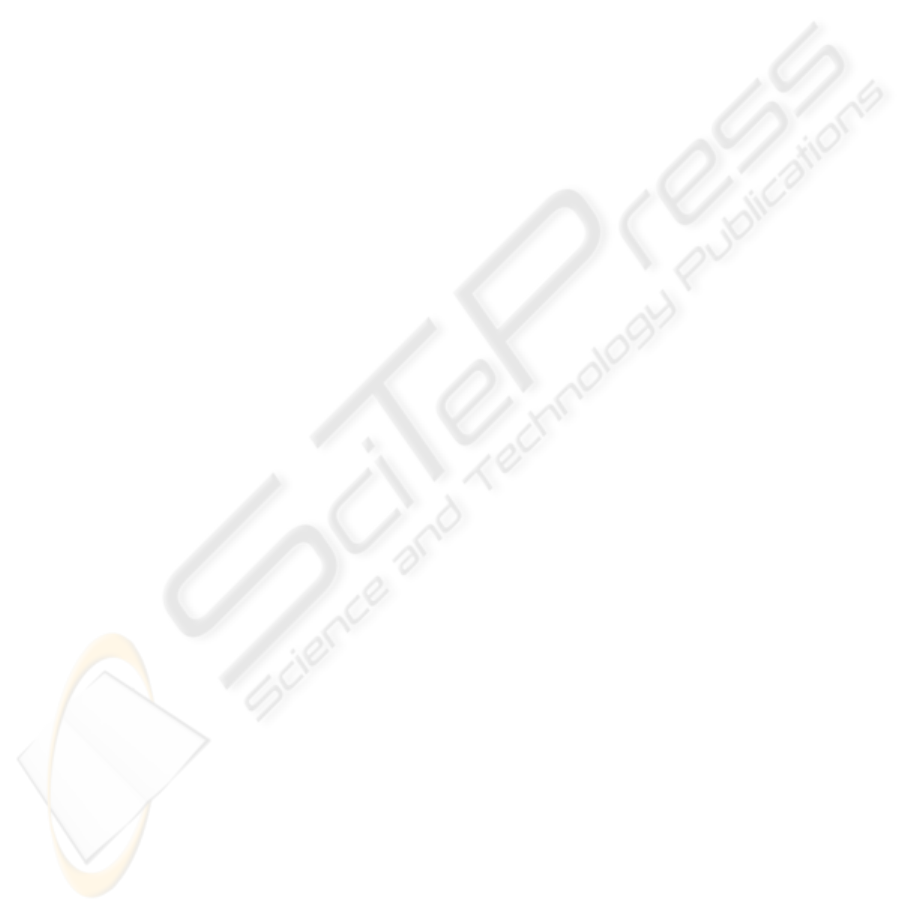
2 APPRAISAL OF STRUCTURAL
FUNDS PROJECT
In the procedure of appraisal referred to applications
for financial support of investment projects from
European funds, evaluation of documents (subjected
before to unification) and operation programmes
should be carried out in three implementation stages
as follows:
• operation programme is prepared and
supplemented (if required) together with
preliminary evaluation, prior to the beginning of
its implementation; so-called: ex-ante
evaluation,
• evaluation is carried out in the middle of
implementation period: so-called mid-term
evaluation,
• evaluation is carried out after the end of
implementation period: so-called ex-post
evaluation.
Prior appraisal of the project proposals can be
based on the analysis of strengths - weaknesses -
opportunities - threats (so-called SWOT).
Preliminary analysis of appraisal referred to
effectiveness of the project, should be done with
taking into consideration such elements as socio-
economical situation in general, situation on the
market, competitiveness and innovativeness. The
variables applied for appraisal of the project should
meet the following criteria:
• pertinence - the variables should be harmonized
with character of the project and with
anticipated effects of its implementation;
• measurability - the variables should be
expressed by means of numerical values;
• credibility - definition of any variable should
enable possibility of its verification;
• accessibility - the data should be easy to obtain.
The above mentioned properties of variables are
taken into account in the process of preparation and
implementation of appraisal models based on the
methods of artificial intelligence.
3 NEURAL MODELS
Principal properties of neural models are important
to determine their effectiveness. Application of
dependences appearing in neural networks, does not
involve formulation of assumptions which are very
difficult for checking. They are characteristic
because of their ability for approximation of optional
nonlinear dependences. They make possible
generalization of learning, from training (historical)
data towards the new data. Formation of neural
model is based on analysis of available historical
data. In result, the main dependences in phenomenon
being investigated, can be esteemed (with the use of
model). These models are useful in case, the
research worker does not know the rules
characteristic for arising of dependences analyzed.
They are particularly useful for description of
variable, complex socio-economical phenomena.
Application of neutral networks requires
adequate preparation related to significant number of
historical data, according to the character of
variables and type of network to be used. However,
this is connected with work and expenditure
necessary to estimate neural model. In this case, it is
most often assumed that number of samples for
learning should be minimum 10 to 20 times higher
than number of weights in the network. Direct and
substitutable dependence between number of
samples from the learning set and precision of
results was not stated empirically. It should be
noted, however, that in extreme cases, at small
number of data (i. e. comparable to the number of
weights), the network in unable to generate proper
results.
Effectiveness of neural networks in appraisal
procedure is expressed as description and analysis of
optional dependences and their generalization. Due
to the above, the neural network is able to give
correct answer to a formulated problem, on the basis
of already transformed input data. In this way
information about regular events from the past is
available. Functioning of the model is based on the
assumption that the information collected is
typically representative for any other data which
might appear. Highly desired property of neural
network is its ability for formulation of proper
answer, even for those input data which are not
included within the set of already collected
information. Applicability of one-way neural
networks for appraisal of investment projects, results
from the fact that socio-economical phenomena have
nonlinear character. These networks are able to
perform approximation of optional nonlinear
dependences and their generalization. They are
adaptable and can help for description of
dependences changing in time. Therefore, during the
input of new information, further network learning
process takes place. Sometimes, the learning process
is limited to small amendments only or to taking into
account transformations of the real system. One-way
neural networks can be particularly useful for
solving the problems of different types of markets
(e.g. capital market, labour market, markets of goods
and services). One-way neuron networks can serve
as well for analysis of problems related to
functioning of enterprise, also while applying for
financial assistance from European funds intended
ICEIS 2004 - ARTIFICIAL INTELLIGENCE AND DECISION SUPPORT SYSTEMS
502