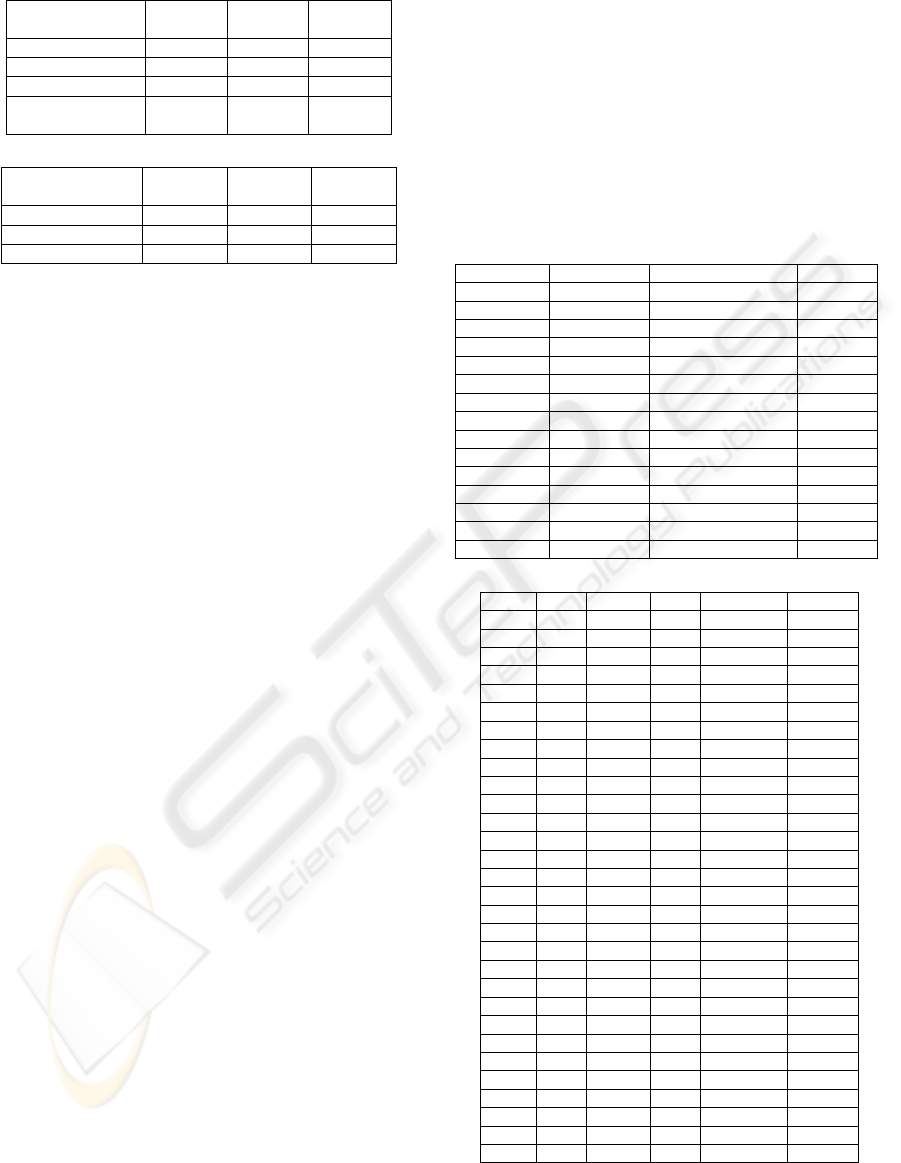
Table 4: Comparing training results.
None
technique
Statistical
analysis
k-means
clustering
Min. error (°C) 0.000035 0.000039 0.017174
Max. error (°C) 1.19 1.021237 0.92959
Error average (°C) 0.15 0.244534 0.33199
N° of iterations
spent in training
7700000 412800 80800
Table 5: Comparing validation results.
None
technique
Statistical
analysis
k-means
clustering
Min. error (°C) 0.02185 0.043265 0.030246
Max. error (°C) 0.70706 1.475292 1.359952
Error average (°C) 0.27365 0.625548 0.458544
ACKNOWLEDGEMENTS
This work has been financially supported by CEMIG
(Energy Company of Minas Gerais - Brazil).
REFERENCES
Morrison, G. L., & Ranatunga, D. B. J. 1980. ‘Transient
response of thermosiphon solar collectors’, Solar
Energy, vol. 24, p. 191.
Huang, B. J. 1984. ‘Similarity theory of solar water heater
with natural circulation’, Solar Energy, vol. 25, p. 105.
Kudish, A. I., Santaura, P., & Beaufort, P. 1985. ‘Direct
measurement and analysis of thermosiphon flow’,
Solar Energy, vol. 35, no. 2, pp. 167-173.
Kalogirou, S. A. 2000. ‘Thermosiphon solar domestic
water heating systems: long term performance
prediction using ANN’, Solar Energy, vol. 69, no. 2,
pp. 167-174.
Kalogirou, S. A., Panteliou S., & Dentsoras A. 1999.
‘Modeling solar domestic water heating systems using
ANN’, Solar Energy, vol. 68, no. 6, pp. 335-342.
Zárate, L. E., Pereira, E. M., Silva, J. P., Vimieiro R.,
Diniz, A. S., & Pires, S. 2003a. Representation of a
solar collector via artificial neural networks. In
Hamza, M. H. ed. International Conference On
Artificial Intelligence And Applications, Benalmádena,
Spain, 8-11 September 2003. IASTED: ACTA Press,
pp. 517-522.
Zárate, L. E., Pereira, E. M., Silva, J. P., Vimieiro, R., &
Diniz, A. S. 2003b. Neural representation of a solar
collector with optimization of training sets
(Unpublished).
Moreira, F., & Roisenberg, M. 2003. Evolutionary
optimization of neural network’s training set:
application in the lymphocytes’ nuclei classification.
In Hamza, M. H. ed. International Conference On
Artificial Intelligence And Applications, Benalmádena,
Spain, 8-11 September 2003. IASTED: ACTA Press,
pp. 358-362.
Duffie, J.A., & Beckman, W. A. 1999. Solar engineering
of thermal processes. 2nd ed. U.S.A.: John Wyley &
Sons, Inc.
Kovács, Z. L. 1996. Redes neurais artificiais, São Paulo,
Brasil: Edição acadêmica, pp. 75-76.
APPEND
Table I.1: Collected data sample.
Tamb Tin Solar Irradiance Tout
25.05 27.17 908.42 33.97
25.91 34.7 1005.68 41.61
23.51 43.42 967.31 49.43
26.26 39.98 761.83 44.73
22.61 25.31 905.41 32.02
23.12 32.82 922.13 39.23
23.75 57.89 958.19 62.21
24.71 38.32 833.93 43.76
25.66 31.65 958.24 38.58
24.49 22.65 872.67 29.46
24.22 23.01 933.09 30.4
23.53 22.83 958.29 30.41
23.96 20.76 768.96 27.28
23.36 39.89 962.33 45.79
25.99 38.11 794.92 43.15
Table I.2: Validation data sets.
Tamb Tin G Tout Tout (ANN) Error
23.83 20.66 755.1 27.17 27.630451 0.460451
24.43 20.97 819.75 27.74 28.14392 0.40392
24.61 21.47 850.02 28.07 28.63377 0.56377
24.44 22.5 860.06 29.27 29.388565 0.118565
24.87 23.72 869.47 30.55 30.365038 0.184962
24.81 25.96 912.59 32.85 32.46125 0.38875
25.31 30.81 932.79 37.52 37.219883 0.300117
25.66 31.65 958.24 38.58 38.304413 0.275587
25.82 33.75 993.54 40.78 40.810246 0.030246
25.85 33.81 996.78 40.86 40.902008 0.042008
25.96 34.65 993.69 41.6 41.772133 0.172133
26.03 34.79 1024.01 41.88 42.176178 0.296178
26.45 37.9 1022.66 44.63 45.445923 0.815923
26.66 38.04 1022.82 45.12 45.592113 0.472113
26.98 38.16 1041 45.27 45.85676 0.58676
26.02 38.18 794.81 43.3 43.93853 0.63853
26.08 39.96 765.05 44.65 45.60505 0.95505
23.77 22.96 924.79 30.32 30.066198 0.253802
24.1 22.98 924.84 30.28 30.099792 0.180208
24.04 23.05 931.81 30.42 30.191616 0.228384
22.76 23.03 907.4 29.93 29.971178 0.041178
23.33 39.98 966.37 46.05 47.142525 1.092525
23.27 40.09 967.81 46.09 47.26527 1.17527
23.74 53.4 983.64 58.6 57.840714 0.759286
25.1 27.13 911.55 33.95 33.501625 0.448375
25.09 27.12 910.06 33.95 33.481182 0.468818
24.65 38.42 849.35 43.85 44.61326 0.76326
24.69 47.01 809.17 51.31 52.669952 1.359952
24.86 23.72 785.5 30.12 29.872047 0.247953
24.96 23.73 770.1 29.83 29.797749 0.032251
ICEIS 2004 - ARTIFICIAL INTELLIGENCE AND DECISION SUPPORT SYSTEMS
152