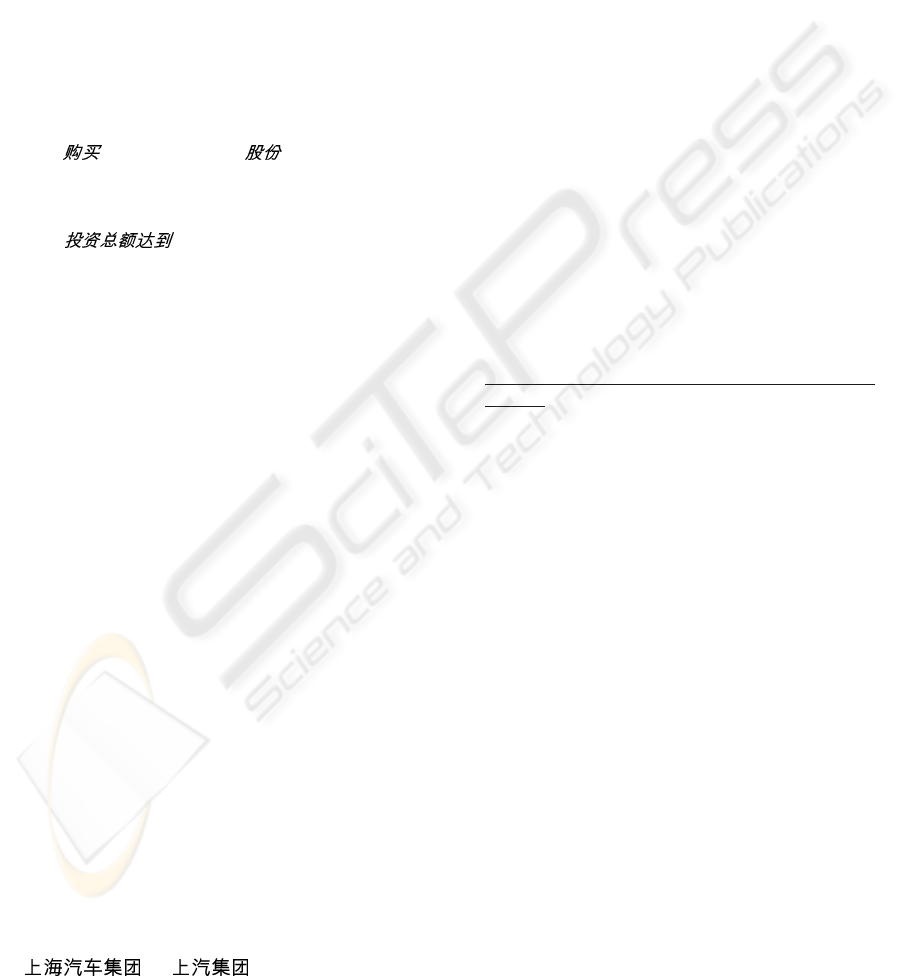
The recall is relatively low which is mainly
caused by the following reasons:
1. In the pattern acquisition, we use only the
positive examples, which is of only 11.89% in
the total training corpus. Although recall on the
training corpus can only reach 42.4%, the recall
on the positive examples will be much better.
2. Many events have few instances even one
instance; they are often of an individual or
infrequent structure. Obviously it is much
difficult to extract an event from few or even one
instance.
Following shows two patterns in investment
domain with its precision in pattern acquisition.
Pattern1 for invested-party with the precision of
88.9%:
Pattern1. +[stuff FIRM-NP]+
Translation: buy stocks of [invested-party FIRM-NP]
Pattern2 for investment amount with precision of
94.1%:
Pattern2. [invest-sum MONEY]
Translation: total investment reaches [invest-sum
MONEY]
6 CONCLUSION
In this paper, we describe a bootstrapping method to
acquire patterns in Information Extraction. Starting
with a tiny seed corpus and patterns, the
bootstrapping process collects new documents from
the Internet and extracts new domain patterns. This
approach overcomes the shortcoming in the scale of
training corpus of traditional method. In order to
improve the precision of acquisition, a classifier is
used to identify new positive and negative examples
for pattern acquisition and evaluation. A statistical
model is used in the classification in our prototype.
At last, we present a “key slots” idea in the
information management module in order to merge
multiple extracted records. Experiments show that
the precision of pattern acquisition of our method is
high.
However there are some points to be improved
in our future work:
1. In classifying the new corpus, the recall is less
than 50%. With the improvement on the recall,
many new patterns can be acquired from the
positive examples; also more negative examples
can be used to evaluate these new patterns.
2. Different forms of a NE can not been identified,
especially between the abbreviation of a
company’s name and its full name. For example,
“
”, “ ”, these tow phrases
are the same company name but in different
forms.
ACKNOWLEDGMENTS
This research work was supported by the grant No.
60083003 from National Natural Science
Foundation of China.
REFERENCES
S. Abiteboul, D. Quass, J. McHugh, J. Widom, and J.
Wiener, 1997. The Lorel query language for
semi-structured data. International Journal on Digital
Libraries, 1(1):68-88, April
S.Cluet, C.Delobel, J.Siemon and K. Smaga, 1998. Your
Mediators Need Data Conversion! in Processing of
ACM-SIGMOD International Conference on
Management of Data, 177-188.
S.Cluet, S. Jacqmin, and J.Siemon, 1999. The New
YTAL: Design and Specifications. Technical Report,
INRIA.
A. Deutsch, M. Fernandex, D. Florescu, A.Levy and D.
Suciu. A query language for XML. in International
World Wide Web Conference, 1999
M. Fernandez, J. Siemon, P. Wadler, 1999. XML Query
Languages: Experiences and Examples.
http://wwwdb.research.bell-labs.com/user/simeon/xqu
ery.html
E. Agichtein & L. Granvno, 2000. Snowball: Extracting
Relations from Large Plain-Text Collections. in
Proceedings of the 5th ACM International Conference
on Digital Libraries.
R. Grishman, S. Huttunen & R. Yangarber, 2002,
Real-Time Event Extraction for Infectious Disease
Outbreaks. in Proceedings of Human Language
Technology Conference (HLT)
S. Soderland, D. Fisher, J. Aseltine & Wendy Lhenert,
1995. CRYSTAL: Inducing a Conceptual Dictionary.
in proceedings of the 14th IJCAI’ 95.
Ellen Riloff & Janyee Wiebe, 2003. Learning Extraction
Patterns for Subjective Extractions. University of
Utah, The Association for Computational Linguistics
(ACL)
Roman Yangarber, 2003. Counter_Training in Discovery
of Semantic Patterns. New York University, the
Association for Computational Linguistics (ACL)
Ellen Riloff, 1995. Little Words Can Make a Big
Difference for Text Classification. University of Utah,
in proceedings of the 18th Annual International
Conference on Research and Development in
Information Retrieval (SIGIR’95)
ICEIS 2004 - ARTIFICIAL INTELLIGENCE AND DECISION SUPPORT SYSTEMS
308