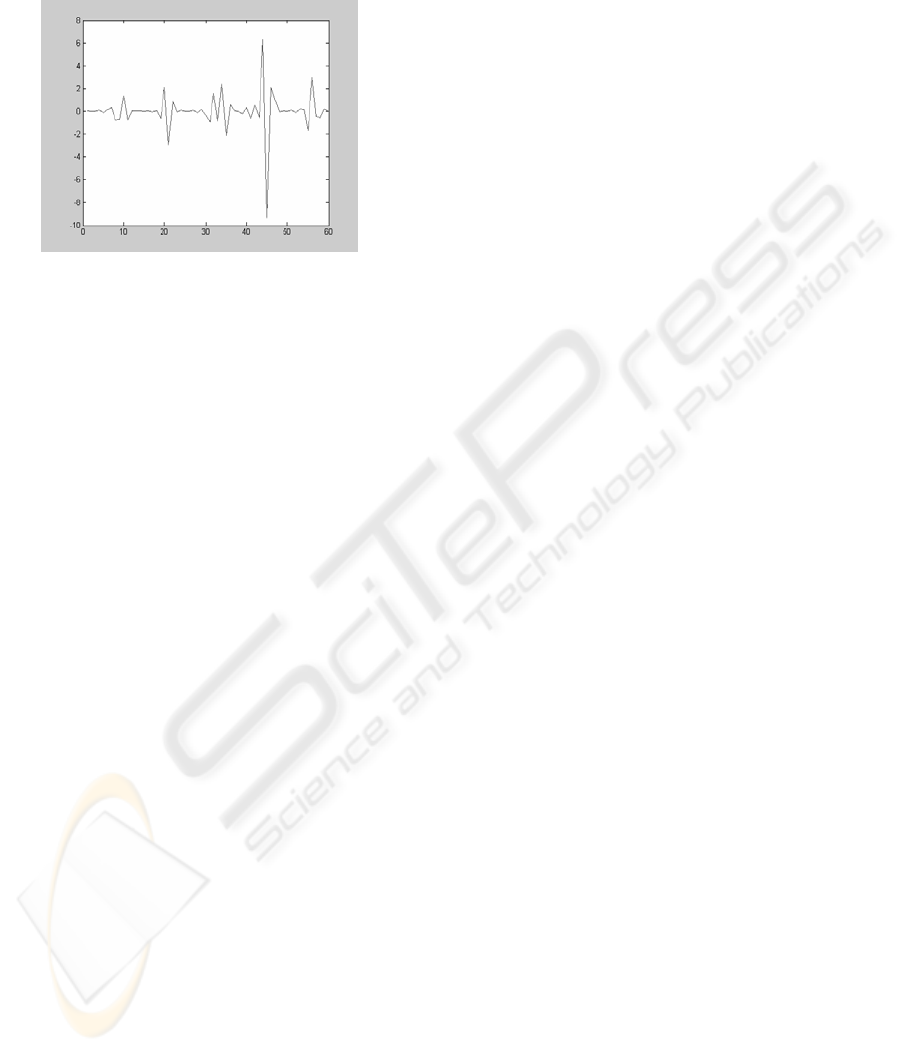
Figure 8 shows the prediction error curve where
it can be seen that the average error is not greater
than 0.2 %.
Figure 8: Percentage Error Curve
5 CONCLUSIONS
The results achieved by the use of the proposed
Elman ANNs for river flow prediction indicate that
they are quite adequate for the flow estimation task.
In the investigated application, the average
prediction error of about 0.2% is much less than that
obtained by traditional ANNs using data Windows
(Haykin, 1999) typically in the order of 5%.
Statistical methods used for flow prediction such as
Box & Jenkins and its variations (Box and Jenkins,
1976) yield an average error larger than 10 %.
For future work, suggestions include the use of
fully recurrent ANNs and ocean temperature data
added to the neural network input. Ocean
temperature is known to have a significant influence
on the river flow values so that sort of information
will be certainly useful for the neural network in
consideration.
REFERENCES
Chatfield E., 1991. The Analysis of Time Series, New
York, USA, Chapman and Hall, fourth edition.
Moraes, J. M., Pellegrino, G., Ballester, M. V., Martinelli,
L. A., Victoria, R. L., 1995. Hydrological Parameters
of a Southern Brazilian Watershed and its Relation to
Human Induced Changes. In Annales of 20th General
Assembly of the European Geophyscial Society, v13:
506-507.
Moraes, J. M., Genovez, A.M., Mortatti, J.,
Pellegrino,G.,Ballester, M.V., Martinelli, L. A, 1996.
Analyses and Modelling of a Flow Time Series under
the Influence of Man Made and Natural Actions. In
Anais do XVII Congresso Latino Americano de
Hidraúlica. (in Portuguese)
Tucci, C. E. M., Robin T. C., Dias P. L. da S., Collischonn
W., 2002. Medium Run Prediction of Reservoirs Flows
based on Weather Forecast. In Final Project
Report:BRA/00/029, Instituto de Astronomia, Geofísica
e Ciências Atmosféricas Universidade de São Paulo
and Instituto de Pesquisas Hidráulicas Universidade
Federal do Rio Grande do Sul. (in Portuguese)
Box, G. E. P., and Jenkins, G. M., 1976. Time Series
Analysis: Forecasting and Control. California, USA.
San Francisco: Holden Day, 2nd. ed.
Hoff C. J., 1983. A Practical Guide to Box-Jenkins
Forecasting, Belmont, CA., USA, Lifetime Learning
Publications.
Fog, T.L. et al, 1995. Training and Evaluation of Neural
Networks for Multi-Variate Time Series Processing. In
Proceedings of IEEE International Conference on
Neural Networks. IEEE Press.
Lachtermacher, G. and Fuller, J.D., 1995.
Backpropagation in Time series Forecasting. In
Journal of Forecasting. Vol 14, 381-393.
Sarle, W.S., 1995. Stopped Training and other remedies
for Overfitting. In Proceedings of the 27th Symposium
on the Interface.
Haykin, S. Neural Networks : A Comprehensive
Foundation, Prentice-Hall, New Jersey, 1999.
Evans, R. M. and S. Alvin, J., 1991. Relating Numbers of
Processing Elements in a Sparse Distributed Memory
Model to Learning Rate and Generalization, In ACM
APL Quote Quad, v21(4), 166-173.
Siqueira, T. G., Soares Filho, Secundino, 2002.
Application of Neural Networks with Radial Basis
Activation Function to the prediction of Non-
stationary Time Series, In XIV Congresso Brasileiro de
Automação. (in Portuguese)
Elman J. L., Finding Structure in Time, 1990. In
Cognitive Science, vol. 14, pp. 179-211.
Jacek M. Zurada and Tomasz J. Cholewo, 1997.
Sequential Network Construction for Time Series
Prediction. In Proceedings of the IEEE International
Joint Conference on Neural Networks, pp 2034–2039.
Ronaldo R. B. de Aquino, Manoel Afonso de Carvalho Jr.,
Benemar Alencar de Souza, 1999. Artificial Neural
Networks: An Application to the Operation Planning of
Hydrothermal Generation Systems. In Proceedings of
the IV Brazilian Conference on Neural Networks - IV
Congresso Brasileiro de Redes Neurais, pp. 164-169.
(in portuguese)
Armando Zeferino Milioni e Acioli Antonio de Olivo,
2001. Econometric Models for the Forecast of River
Floods . In Simpósio Brasileiro de Pesquisa
Operacional. (in portuguese)
Tucci, C. E. M., Brun, Gerti, 2001. Real Time Forecast of
the Volume Flowing to the Reservoir of Ernestina. In
Revista Brasileira de Recursos Hídricos. v.6, n.2, p.73
– 79. (in Portuguese)
Cichocki, A., Unbehauen, R., 1996. Neural Networks for
Optimisation and Signal Processing, New York, USA,
John Wiley & Sons, Inc.
month
error %
ICEIS 2004 - ARTIFICIAL INTELLIGENCE AND DECISION SUPPORT SYSTEMS
158