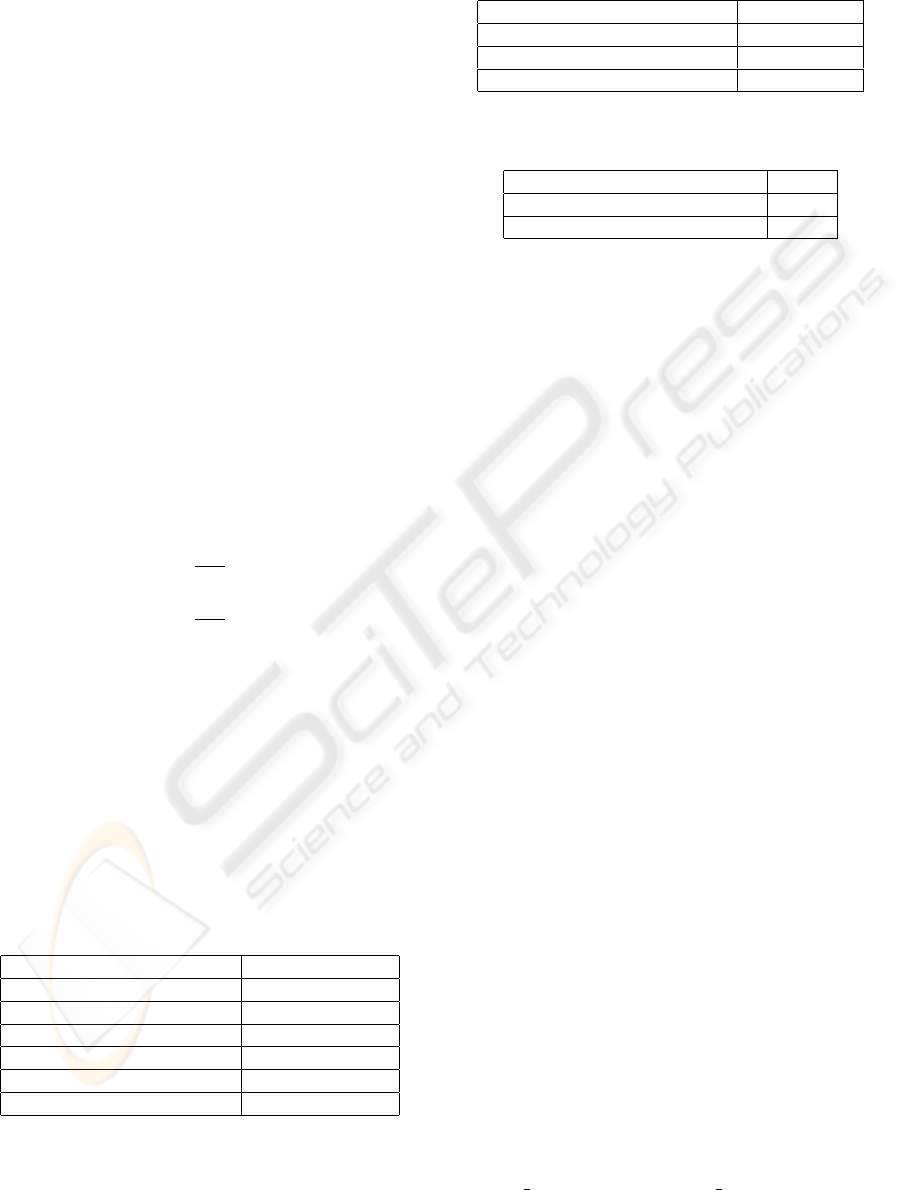
1986 to 2000 (Figure 1). The output variable (the
modelled variable) is the indebtedness for the Span-
ish autonomous region in the year t, and the input
variables are the region indebtedness of the previ-
ous year D/P IB(t − 1), the non financial revenues
IN F/P IB(t) and the type of interest T (t). Thus,
the searched function F is shown in Equation 10.
F (D/P IB(t − 1), INF/P IB(t), T (t)) (10)
The fuzzy model F
0
is developed using the Clas-
sification and Regression fuzzy tree (ADRI). A fuzzy
rule consist of two components, the first one is the an-
tecedent and the second one is the consequent. These
components show the cause-effect relation. The an-
tecedent is formed for aggregation of fuzzy proposi-
tions, such as X is A, where X is the input variable
and A is the fuzzy set defined over its domain. In this
work, the membership function of the fuzzy sets are
trapezoidal functions (see Equation 11). This mem-
bership function is adequate for our problem due to it
is one of the most simple membership function, and
it is easy to construct from the fuzzy sets obtains by
using ADRI (ADRI is defined over trapezoidal sets).
The change to other membership function not implies
substantial modifications.
A(x, a, b, c, d) =
0 x 6 a
x−a
b−a
a < x < b
1 b 6 x 6 c
d−x
d−c
c < x < d
0 x > d
(11)
Our aim is to define a system of linguistic rules,
since linguistic labels must be associated to each one
of the fuzzy sets in which is divided the domain of
each input variable (Tables 1, 2 and 3). Each row of
these tables define a linguistic value. The numbers
which appear in the first column of the tables are the
four values that define the trapezoidal function of the
linguistic value given in its associated second column.
Thereby, we get a set of linguistic variables (Zadeh,
1975).
Table 1: Linguistic Variable D/PIB
[8.00, 8.48, MAX, MAX] Extremely Big
[6.45, 7.20, 8.00, 8.48] Very Big
[3.53, 4.50, 6.45, 7.20] Big
[1.50, 2.10, 3.53, 4.50] Norm
[0.80, 1.10, 1.50, 2.10] Small
[0.40, 0.60, 0.80, 1.10] Very Small
[MIN, MIN, 0.40, 0.60] Extremely Small
The model acquired by ADRI may be observed in
Table 4. Each row is a rule with EB is Extremely Big,
V B is Very Big, B is Big, N is Norm, S is Small,
Table 2: Linguistic Variable INF/PIB
[14.08, 15.62, MAX, MAX] Big
[8.68, 10.84, MAX, MAX] Norm or Big
[8.96, 10.84, 14.02, 15.62] Norm
[MIN, MIN, 8.68, 10.84] Small
Table 3: Linguistic Variable T
[11.05, 12, 49, MAX, MAX] Big
[8.22, 9.65, 11.05, 12.49] Norm
[MIN, MIN, 5.87, 9.65] Small
V S is Very Small and ES is Extremely Small. These
rules are arranged in a decreasing way according to
the percentage of data that are correctly classified by
the rule. The first number indicates the index of the
rule, the following four are the values that the input
variable take, the sixth the rule error and the last per-
centage of instances that has been correctly classified.
Thus, the rule 10 covers the 7.4% of the data, it has a
error of 0.04%, and it could be read as:
IF D/P IB(t − 1) is Big AND IN F/P IB(t)
is Small AND T (t) is Small THEN
D/P IB(t) is 4.55
Now, let us look at how the model responds to a
situation. Table 5 shows how is carried out the infer-
ence from the data about Andalusia in 1993. Each
row of this table is a rule of the model (see Ta-
ble 4). The first number indicates the index of the
rule, the following three are the values that the in-
put variables (D/P IB(t − 1), INF/P (t) and T (t))
take in each rule (value 1), the fifth the minimum
membership grade for the input variables and the last
the value of the output variable. The real output is
D/P IB(t) = 6.6. The data about Andalusia, input
of the model, are 5.3, 18.96 and 10.17 for the input
variables D/P IB(t − 1), INF/P IB and T (t) re-
spectively. The output value obtained by the model is
D/P IB(t) = 6.23.
The cell (1, D/P IB(t − 1)) contains the mem-
bership grade of the value 5.3 to the fuzzy set
[0.80, 1.10, 1.50, 2.00] labelled as Small. The value
into the cell (9, min) represents the minimum value
of the membership of the input values set to the rule
9 (row 9). The cell value (9, D/P (T )) is the output
of the rule 9 multiplied by the cell value (9, min).
The output value is the weighted average of all out-
puts times the min column.
In Figures 2 to 16 we show the predictive perfor-
mance of the model. Each Figure presents the results
of a Spanish region, the real and obtained indebted-
ness (Y
axis) in each year (X axis). The real in-
debtedness is represented by a continuous line. The
A QUALITATIVE MODEL OF THE INDEBTEDNESS FOR THE SPANISH AUTONOMOUS REGIONS
277