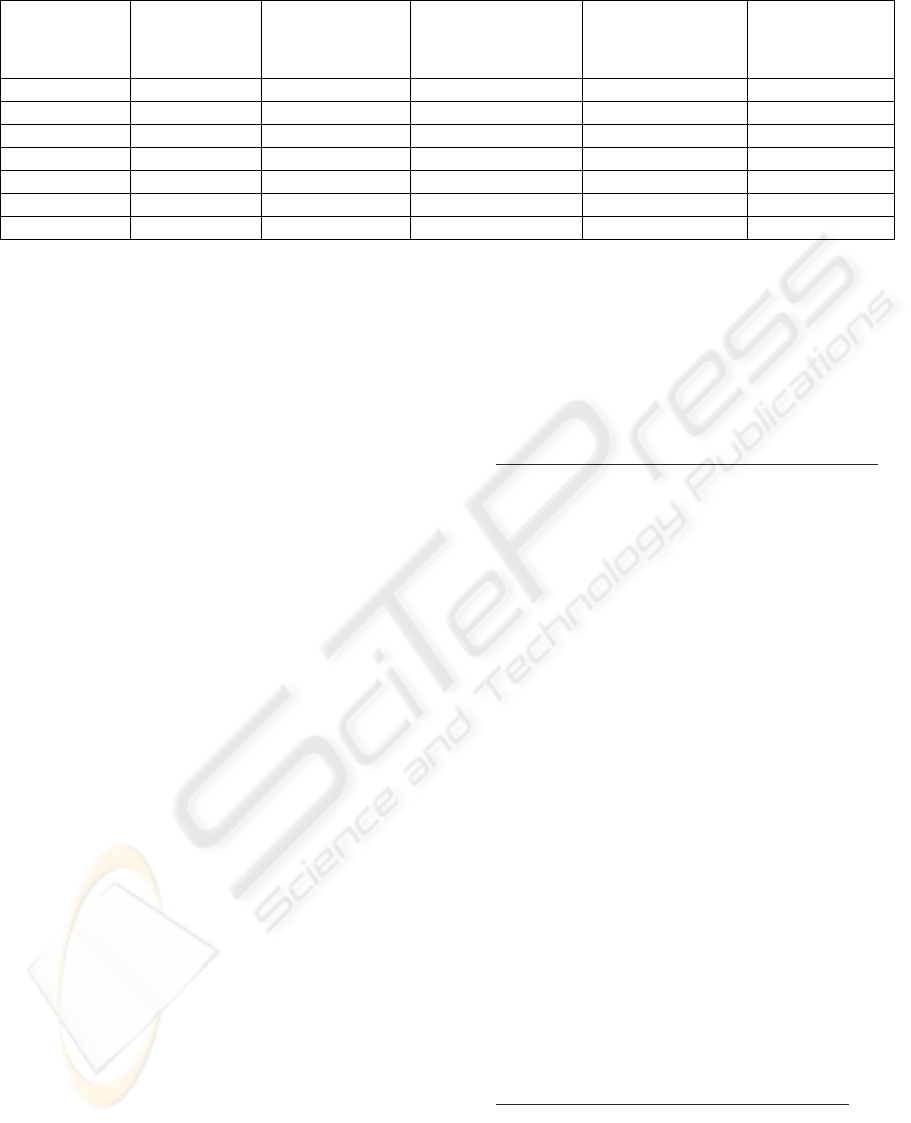
Table 2: Summary of results with 3 angular categories
Dataset Length Number of
frequent
trends
Frequent trends
spanning at ≥ 4
time periods
Average freq. of
trends spanning
≥ 4 periods
Num. of trend
associations
Balloon 2001 1200 1161 6 101
Darwin 1400 743 705 9 330
Foetal_ecg 2500 1510 1471 6 159
Greatlakes 984 510 471 8 221
Industrial 564 346 316 7 114
Soiltemp 2304 1404 1365 7 176
Sunspot 2899 1783 1744 7 159
REFERENCES
Agrawal, R., Psaila, G., Wimmers, E.L., and Zait, M.,
1995. Querying Shapes of Histories, Proceedings of
the 21
st
VLDB Conference, Zurich, Switzerland.
Allen, J.F., 1983. Maintaining Knowledge about Temporal
Intervals, Comm. ACM, 26(11):832-843.
Bieganski, P., Riedl, J., Carlis, J.V., and Retzel, E.R.,
1994. Generalized Suffix Trees for Biological
Sequence Data: Applications and Implementation, In
Proceedings of the 27
th
Hawaii Int’l Conference on
Systems Science, IEEE Computer Society Press, pages
35-44.
Das, G., Lin, K-I., Mannila, H., Ranganathan, G., and
Smyth, P., 1998. Rule Discovery from Time Series,
Proceedings of the 4
th
International Conference on
Knowledge Discovery and Data Mining, [KDD98],
New York, NY, pages 16-22.
Faloutsos, C., Ranganathan, M., and Manolopoulos, Y.,
1994. Fast Subsequence Matching in Time-Series
Databases, in Proceedings of the 1994 ACM SIGMOD
International Conference on Management of Data,
pages 419-429.
Gusfield, D., 1997. Algorithms on Strings, Trees, and
Sequences: Computer Science and Computational
Biology, Cambridge University Press.
Han, J., Gong, W., and Yin, Y., 1998. Mining Segment-
Wise Periodic Patterns in Time Series Databases,
KDD, pages 214-218.
Harms, S.K., Deogun, J., Saquer, J., and Tadesse, T.,
2001. Discovering Representative Episodal
Association Rules from Event Sequences Using
Frequent Closed Episode Sets and Event Constraints,
Proceedings of the IEEE International Conference on
Data Mining, Silicon Valley, CA, pages 603-606.
Hoppner, F., 2001. Discovery of Temporal Patterns,
Learning Rules about the Qualitative Behaviour of
Time Series, in De Raedt, L., Siebes, A., (Eds.),
PKDD 2001, LNAI 2168, Springer-Verlag, Berlin,
pages 192-203.
Indyk, P., Koudas, N., and Muthukrishnan, S., 2000.
Identifying Representative Trends in Massive Time
Series Data Sets Using Sketches, In Proceedings of the
26
th
Int’l Conference on Very Large Data Bases,
Cairo, Egypt, pages 363-372.
Keogh, E., 2003. The UCR Time Series Data Mining
Archive,
http://www.cs.ucr.edu/~eamonn/TSDMA/index.html
,
University of California – Computer Science and
Engineering Department, Riverside, CA.
Keogh, E.J., Chakrabarti, K., Pazzani, M.J., and Mehrotra,
S., 2000. Dimensionality Reduction for Fast Similarity
Search in Large Time Series Databases, Journal of
Knowledge and Information Systems, vol 3, number 3,
pages 263-286.
Mannila, H., Toivonen, H., and Verkamo, A.I., 1997.
Discovery of Frequent Episodes in Event Sequences,
Report C-1997-15, Department of Computer Science,
University of Helsinki, Finland.
Patel, P., Keogh, E., Lin, J., and Lonardi, S., 2002. Mining
Motifs in Massive Time Series Databases, Proceedings
of the IEEE Int’l Conference on Data Mining,
Maebashi City, Japan.
Perng, C-S., Wang, H., Zhang, S.R., and Parker, D.S.,
2000. Landmarks: A New Model for Similarity-,
Based Pattern Querying in Time Series Databases,
Proceedings of the 16
th
IEEE International Conference
on Data Engineering.
Qu, Y., Wang, C., Wang, X.S., 1998. Supporting Fast
Search in Time Series for Movement Patterns in
Multiple Scales, Proceedings of the ACM 7
th
International Conference on Information Management,
pages 251-258.
West, M., 2003. Some Time Series Data Sets, retrieved
June 18, 2003, from
http://www.stat.duke.edu/~mw/ts_data_sets.html
,
Duke University.
Yi, B-K, Faloutsos, C., 2000. Fast Time Sequence
Indexing for Arbitrary L
p
norms, in The VLDB
Journal, pages 385-394.
AN EFFICIENT FRAMEWORK FOR ITERATIVE TIME-SERIES TREND MINING
137