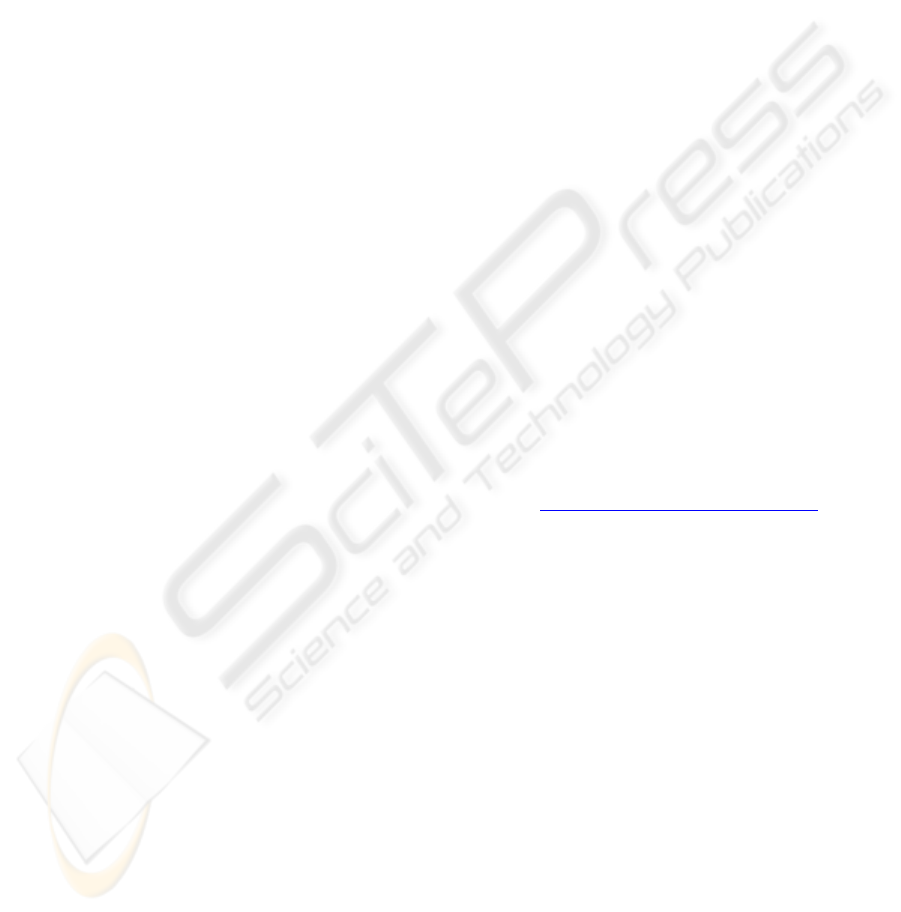
topic, and an is-neighbor topic. Selected topics are
those topics whose documents are judged as relevant
information by users in the previous search round.
Is-child and is-neighbor topics are those topics that
are connected by an is-child relation and an is-
neighbor relation respectively with selected topics.
Again, a document is said to be a relevant document
if users award it a 4 or a 5. Figure 12 shows that the
proportion of is-child topic drops gradually. This
probably means that once users have reached
suitable topics through a few rounds, any further
specific child topics are less important to users. A
continuous increase in the proportion of target topics
reflects the fact that relevant feedback improves
search quality. Moreover, there are some relevant
documents belong to is-neighbor topics in each
result round. That means is-neighbor topics have
some contributions for finding relevant information.
6 CONCLUSIONS
In this paper, we discuss the pattern of cross-topic
reference in Yahoo. The findings show that topic
relationships are not limited to a parent-child
relation. Instead, there are some significant and
useful relationships among relative topics or topics
from different branches. Is-child and is-neighbor
relations have been defined to represent those
relationships among the topics, and ST Nets are
formed based on such relations. Experiments show
that users using the incremental relevant feedback
and ST Nets are able to get the most relevant result
with fewer result rounds. In future, ST Nets will be
further investigated. Now we only consider those
topics that are all connected by is-child relations, or
are linked by an is-neighbor relation directly.
However, there are more types of combinations of
is-child and is-neighbor relations that should bring
some meaningful information for different
situations. In addition, we are trying to apply ST
Nets to different information retrieval systems in the
fact that topic relationships should have a wide
range of usage for information retrieval.
ACKNOWLEDGEMENTS
The research reported in this paper was partially supported
by the Hong Kong Polytechnic University Research Grant
A-PE35.
REFERENCES
Aalbersberg, I. J., 1992. Incremental Relevance
Feedback,15
th
Ann Int’I SIGIR ’92, Denmark, pp. 11-
22.
Allan, J., 1996, Incremental Relevance Feedback for
Information Filtering, SIGIR’96, Zurich, pp. 270-278.
Chau, M., Zeng, D., Chen, H., 2001. Personalized Spiders
for Web Search and Analysis, JCDL ’01, Virginia,
USA, 24-28 Jun, pp. 79-87
Ingwersen, P., 1992. Information Retrieval Interaction,
Taylor-Graham.
Iwayama, M., 2000. Relevance Feedback with a Small
Number of Relevance Judgements: Incremental
Feedback vs. Document Clustering, SIGIR’00,
Athens, Greece, July, pp. 10-16.
Jansen, B. J., Spink, A., Saracevic, T., 2000. A study and
analysis of users queries on the Web, Information
Processing & Management 36. 2., pp. 207-227.
Jeh, G., Widom, J., 2003. Scaling Personalization Web
Search, WWW2003, Hungary, 20-24 May, pp. 271-
279.
Jensen F. V., 1999. The book, Bayesian Networks and
Decision Graph, Springer.
Kelly, D., Cool, C., 2002. The Effects of Topic Familiarity
on Information Search Behavior, JCDL ’02, Portland,
Oregon, USA, 13-17 Jul, pp. 74-75.
Liu, B., Chin, C. W., Ng, H. T., 2003. Mining Topic-
Specific Concepts and Definitions on the Web, WWW
2003, Budapest, Hungary, 20-24 May, pp. 251-260.
Liu, F., Yu, C., Meng, W., 2002. Personalized Web Search
by Mapping User Queries to Categories, CIKM ’02,
Virginia, USA, 4-9 Nov, pp. 558-565.
Page, L., Brin, S., Motwani, R., Winograd, T., 1998. The
PageRank citation ranking: Bringing order to the Web.
Technical report, Standford University Database
Group,
http://citeseer.nj.nec.com/368196.html.
Pearl J., 1998. Probabilistic Reasoning in Intelligent
Systems: networks of plausible inference, Morgan
Kaufmann Publishers, San Mateo, CA.
Pitkow, J., Shcutze, H., Cass, T., Cooley, R., 2002.
Personalized Search, Communications of the ACM,
Vol. 45, No. 9, pp.50-55.
Popescul, A., Ungar, L. H, Pennock, D. M., Lawrence, S.,
2001. Probabilistic Models for Unified Collaborative
and Content-Based Recommendation in Sparse-Date
Environment, UAI, Seattle, USA, 2-5 Aug 2-5,
pp.437-444.
Rocchio J. J., 1971. Relevance Feedback in Information
Retrieval, Prentice-Hall Incorporation.
Salton, G., and Buckley, C., 1990. Improving Retrieval
Performance by Relevance Feedback, Journal of the
America Society for Information Science, 41(4), pp.
288-297.
White, R. W., Ruthven, I., Jose, J. M., 2002. The use of
implicit evidence for relevance feedback in web
retrieval, European Conference on Information
Retrieval Research, UK, March, pp. 93-109.
APPLYING CROSS-TOPIC RELATIONSHIPS TO INCREMENTAL RELEVANCE FEEDBACK
363