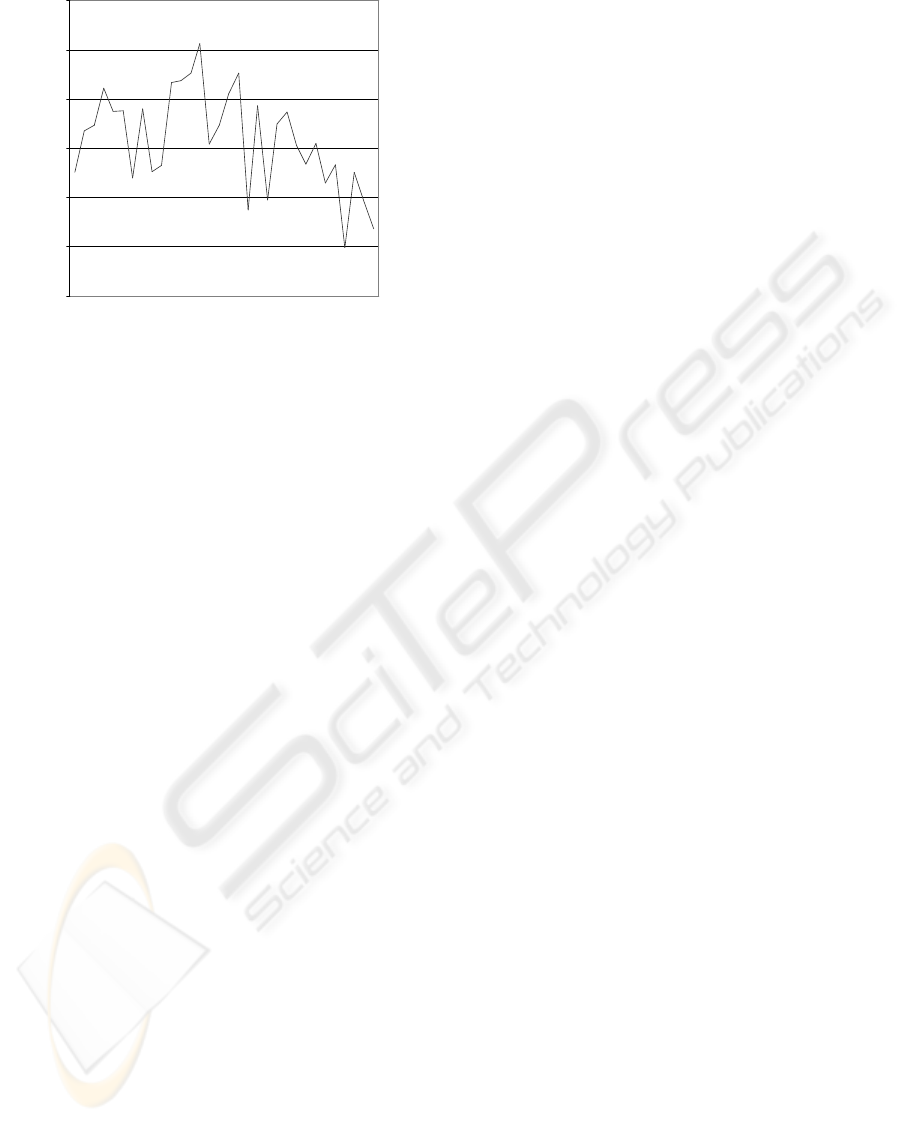
0
0.05
0.1
0.15
0.2
0.25
0.3
Students sorted according to average results
Standard deviation of module results per student
Figure 5: Standard deviation: results / module / student
by transactional systems. It is argued in this article
that the types of correlation and the trends found, de-
pend on the mindset of the data miner. To support this
argument, it is shown that within the student = ma-
terial paradigm, the learning processes can be mon-
itored by applying established SPC techniques. The
limited scope of this exercise does not allow gener-
alised conclusions, but sets the scene for future re-
search that may include the following:
• Identifying and managing other assignable sources
of variation.
• Identifying and defining control limits for the learn-
ing process, based on more data, given assignable
variation.
• Given data of more learning events (as extracted
from the LMS), identifying common variation in
student performance per student. Applying regres-
sion to find correlation between different courses
Assessment results per student are captured by
transactional systems (LMS and SIS), whether or not
these are used for quality control purposes. If these
data could be used for quality control purposes, time
and effort could be saved in gathering quality data
through surveys and by other means.
REFERENCES
Berson, A. (1999). Building Data Mining Applications
for CRM. McGraw-Hill, Osborne Media, isbn:
0071344446 edition.
Besterfield-Sacre, M. (1998). Implications of statistical pro-
cess: Monitoring for abet 2000 program evaluation:
An example using freshman engineering attitudes. In
American Society for Engineering Education.
Braha, D. (1998). Data Mining for Design and Manufac-
turing: Methods and Applications. Kluwer academic
publishers, International, 1-4020-0034-0 edition.
Chase, R. (2004). Operations Management for Competitive
Advantage. Irwin, Boston, 10th ed edition.
Educase (2001). Higher education data warehouse config-
urations page. In Decision Support/ Data Warehouse
Contituency group. Retrieved August 5, 2003, from
http://www.georgetown.edu/users/allanr/dwconfig/.
Ingham, J. (2002). Data warehousing: A tool for the out-
comes assessment process. In In IEEE Transactions
on Education. IEEE,Vol. 43, no. 2.
Keller, G. (1992). Increasing quality on campus: What
should colleges to about tqm mania? In Change. Vol
24.
Kimball, R. (2002). The data warehouse toolkit. John Wiley
and Sons, International Edition, 0-07-113478-6 edi-
tion.
Lee, S. (2001). A review of data mining techniques. In
Industrial Management and Data Systems. Vol 101,
Issue 1, 0263-5577.
Luan, J. (2001). Data mining as driven by knowledge man-
agement in the higher education - persistance cluster-
ing and prediction. In Proceedings of SPSS Public
Conference. UCSF.
Lumsdaine, E. . M. (1995). Creative Problem Solving.
McGraw-Hill, International Edition, 0-07-113478-6
edition.
Lundy, J. (2002). Gartner’s e-learning glossary. In Research
Note. Gartner research note, M-14-9025.
Murray, C. (1 May 2001). A high level look at pace univer-
sity student information system, pace university. In
submitted to ERP Project Office, Report no. Issue 1.
Pace University.
SPSS (2003). Spss om higher education. In
SPSS. Retrieved August 10, 2003, from
http://www.spss.com/applications.
TwoCrows (1999). Introduction to data mining and knowl-
edge discovery. 3rd edition.
Weber, R. (2002). The use and value of data warehousing
in higher education. In Mountain Plains Journal of
Business and Economics. Vol. 10.
Willis, T. (1999). Total quality management and higher ed-
ucation: the employers’ perspective. In Total Quality
Management. Vol. 10, No. 7.
Yanow, E. (1998). Data warehouse stores mit infor-
mation in a central place. In MIT News Of-
fice, TechTalk. Retrieved September 21, 2003, from
http://web.mit.edu/newsoffice/tt/1998/sep30/
warehous.html.
Zaiane, O. (1995). Resource and knowledge discovery in
global information systems: A scalable multiple lay-
ered database approach. In Proceedings Conference
on Advances in Digital Libraries, Washington DC.
WAREHOUSING AND MINING OF HIGHER EDUCATION DATA BY MEANS OF STATISTICAL PROCESS
CONTROL
115