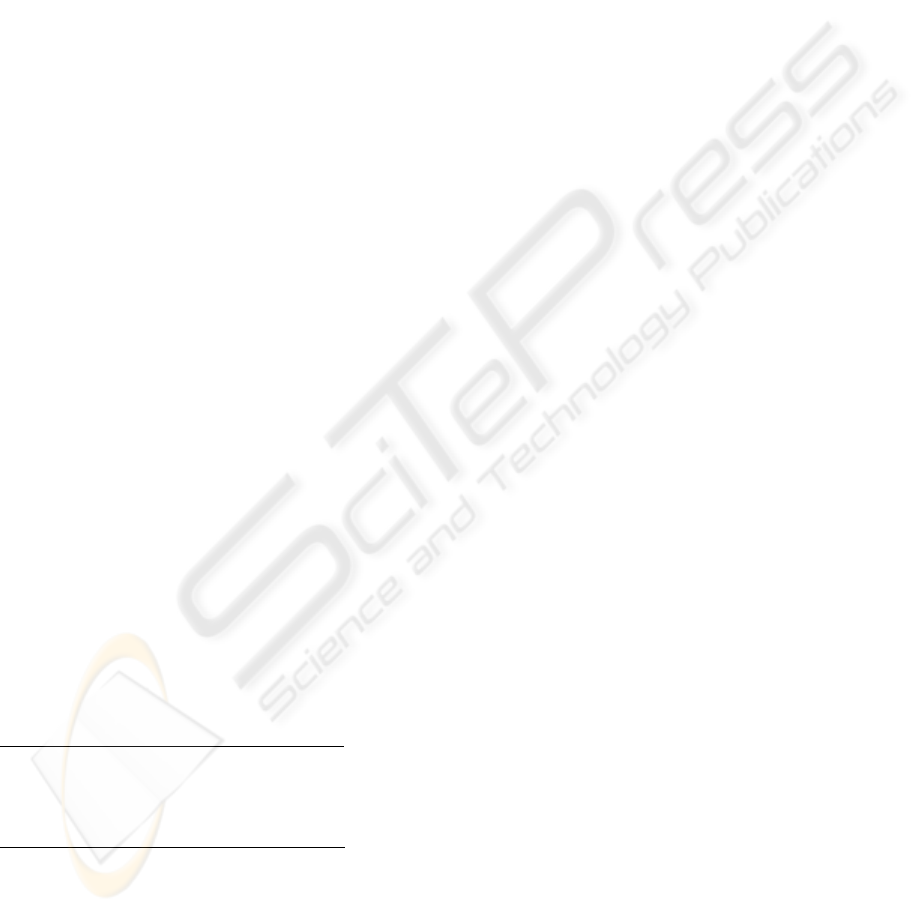
And also the amount of the catalyst at the previous
time point is higher than the amount of the catalyst
at the current time point. The amount of the products
at the post time point is higher than the amount of
the products at the current time point. We look at the
reactant and the catalyst at the previous time point as
the predisposing factor and look at the product as the
co-incident factor. The fact about the catalyst is it
will not be transformed to be the product, so after
the reaction finish, we will get the catalyst back. We
will see the amount of the catalyst at the post time
point is higher than the amount of the catalyst at the
current time point. So we look at the catalyst at the
post time point as the co-incident factor as well.
4 EXPERIMENTS
We apply our method with one OSS data set which
consists of 17 attributes (Project name, Month-Year,
Rank0, Rank1, Page-views, Download, Bugs0,
Bugs1, Support0, Support1, Patches0, Patches1,
Tracker0, Tracker1, Tasks0, Tasks1, CVS. This data
set consists of 41,540 projects, 1,097,341 records
4.1 Results
We set the rate of the data change threshold of the
Download attribute and the rest of all of the other
attributes as 1.5.
4.1.1 In case without considering the slope
rate direction of the Download
attribute
Predisposing Factor(s): Tasks0, Tasks1, CVS
Co-incident Factor(s): Support0, Support1,
Patches0, Patches1
4.1.2 In case considering the slope rate
direction of the Download attribute
The acceleration of the Download attribute
Predisposing Factor(s): none
Co-incident Factor(s): Bugs0, Bugs1, Support0,
Support1, Patches0, Patches1, Tracker0, Tracker1
The deceleration of the Download attribute
Predisposing Factor(s): Bugs0, Bugs1, Support0,
Support1, Patches0, Tracker0, Tasks0, Tasks1, CVS
Co-incident Factor(s): Support1
5 CONCLUSION
The combination of the existing methods and the
new idea from the fact seen in the chemical reaction
to be our new algorithms can be used to mine the
predisposing factor and co-incident factor of the
reference event of interest very well. As seen in our
experiments, our propose algorithms can be applied
with both the synthetic data set and the real life data
set. The performance of our algorithms is also good.
They consume execution time just in linear time
scale and also tolerate to the noise data.
REFERENCES
Freemantle, M., 1995. Chemistry in Action. Great Britain,
MACMILLAN PRESS.
Harrison, R. M., Mora S., et al., 1991. Introductory
chemistry for the environmental sciences. Cambridge,
Cambridge University Press.
Last, M., Klein Y., et al., 2001. Knowledge Discovery in
Time Series Databases. In IEEE Transactions on
Systems, Man, and Cybernetics 31(1): 160-169.
Liska, K. and Pryde L., 1984. Introductory Chemistry for
Health Professionals. USA, Macmillan Publishing
Company.
Robinson, W. R., Odom J., et al., 1997. Essentials of
General Chemistry. USA, Houghton Mifflin
Company.
Roddick, J. F. and Spiliopoulou M., 2002. A Survey of
Temporal Knowledge Discovery Paradigms and
Methods. In IEEE Transactions on Knowledge and
Data Mining 14(4): 750-767.
Snyder, C. H. 1998. The Extraordinary Chemistry of
Ordinary Things. USA, John Wiley & Sons, Inc.
ICEIS 2004 - ARTIFICIAL INTELLIGENCE AND DECISION SUPPORT SYSTEMS
534