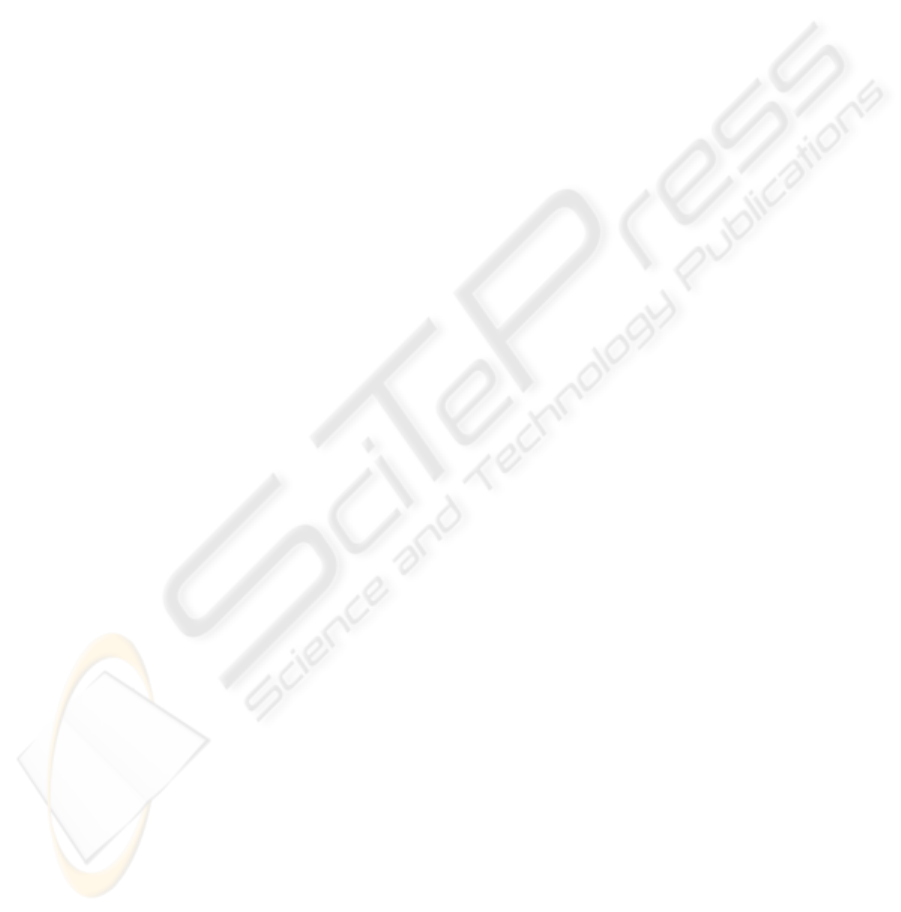
food processing, leather goods, textiles, construction,
tourism }} ⇒
{Area_total ~ 446550.0, Area_land ~ 446300.0,
Population ~ 31167783, Debt external ~ 19000.0}
(25%, 72%)
These two rules states that countries with few
types of industries, which include the 5 ones
mentioned in the rules, use to have a large area,
being it mainly land, a high population, a very
high external debt, as well as a low proportion of
cultivated area.
• {Natural resources ~ {gold, copper, silver, natural gas,
timber, oil, fisheries}} ⇒ {Area_total ~ 462840.0,
Area_land ~ 452860.0, Population ~ 5172033,
Population below poverty line ~ 37%} (23.3%, 41.83%)
This rule indicates that many countries with
considerable natural resources have a high percent
of people living below poverty line.
• {Map references ~ Central America and the Caribbean}
⇒ {Infant mortality rate ~ 24.2, Life expectancy at birth
female ~ 71.25} ( 23.3%, 93.02%)
The majority of the countries of the Central
America and the Caribbean have a medium infant
mortality rate, whereas the life expectancy of the
female population is relative high. It is worth
mentioning that the algorithm has not found any
association between these countries and the life
expectancy of the male population.
5 CONCLUSIONS
This paper presents a general framework for mining
complex objects that can include either single and
multi-valued attributes of any type. The mining
process is guided by the semantics associated to
each object attribute, which are stated by selecting
the appropriate representation model. Preliminary
experimental results show the usefulness of the
proposal.
In future works, we will analyze how to measure
the relevance of the co-occurrences and the
association rules by using background knowledge
that minimizes the number of associations presented
to the user.
Moreover, there are many subdescriptions that are
similar to each other according to the user-defined
similarity function. Consequently, they are presented
to the user as different cases. Hence, it is necessary
to group similar subdescriptions and to represent
each group with a representative. For this purpose,
traditional clustering algorithms could be applied.
Finally, another application under study is the
mining of XML documents, which can be seen as
complex objects with nested structures. Here, the
problem is to deal with the hierarchical nature of
objects. This has been recently treated by the authors
in the context of text mining (Danger et al, 2003).
REFERENCES
Agrawal, R.; Imielinski, T.; Swami, A., 1993. Mining
Association Rules between Sets of items in Large
DataBases. In Proceeding of ACM SIGMOD.
Agrawal, R.; Srikant, R, 1994. Fast algorithms for mining
association rules. In Proceedings of the 20th
International Conference on Very Large Databases,
pages 487-499.
Danger, R.; Berlanga, R., Ruíz-Shulcloper, J., 2003. Text
Mining using the hierarchical syntactical structure of
Documents. In Proceeding of the 10th CAEPIA, pages
139-148.
Gyenesei, A., 2000. Mining Weighted Association Rules
for Fuzzy Quantitative Items. In Proceedings of
PKDD Conference, pages 416-423.
Han, J.; Nishio, S.; Kawano, H.; Wang, W., 1998.
Generalization-based data minimg in object oriented
databases using an object Cube Model. Data and
knowledge engineering, pages 55-97.
Hipp, J: Myka A.; Wirth R.; Günttzer U., 1998. A new
Algorithm for faster mining of Generalized
Association Rules. In Principles of Data Mining and
Knowledge Discovery.
Miller, R.J. and Yang, Y., 1997. Association rules over
interval data. In Proceedings of ACM-SIGMOD,
pages 452-461.
Savasare, A.; Omiecinski, E.; Navathe, S., 1995: An
efficient Algorithm for Mining Association Rules in
Large Databases. Technical Report No. GIT-cc-95-04.
College of Computing. Georgia’ Institute of
Technology.
Srikant, R. Agrawal, R, 1995. Mining Generalized
Association Rules, In Proceedings of Very Large
Databases.
Srikant, R., Agrawal, R.:, 1996. Mining quantitative
association rules in large relational tables. In
Proceedings of ACM SIGMOD.
Zhang, Z., Lu, Y. Zhang, B., 1997:.An effective
Partitioning-Combining Algorithm for Discovering
Quantitative Association Rules. In Proceedings of the
First Pacific-Asia Conference on Knowledge
Discovery and Data Mining.
OBJECTMINER: A NEW APPROACH FOR MINING COMPLEX OBJECTS
47