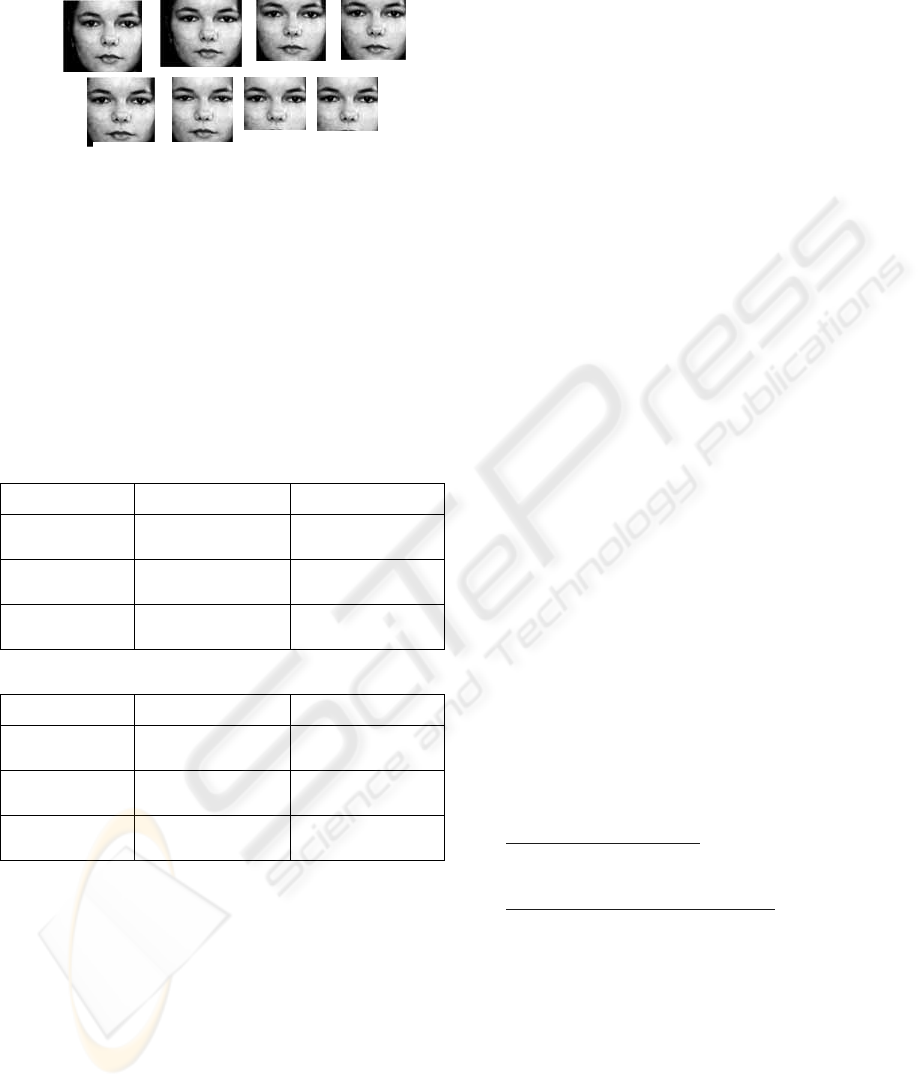
region with the greatest weight, as shown in Figure
5.
The figure above shows all regions obtained
without the intersection verification function. By
using that function, the result will be only one
figure: the one placed in the first line and the third
column.
Confirmation of the efficacy was done through
the use of 100 images – 50 male and 50 female –
from (PICS, 2003). Each image was used as an input
to the developed systems at two different threshold
values. The results are shown in Tables 2 and 3.
Table 2: Weighting Mask Results with 2 threshold values
T 0.22 0.38
Processing
Mean Time
15.20 seconds 31.62 seconds
Correct
detection
81% 48%
False
detection
25% 21%
Table 2: ANN Results with 2 threshold values
T 0.22 0.38
Processing
Mean Time
12.11 seconds 19.15 seconds
Correct
detection
88% 67%
False
detection
6% 12%
False detection is characterized by segmentation
and high punctuation for a non-face region. In some
cases, the correct face was detected and there was
false detection to another cut area to the same figure.
The best result for T=0.22 is explained by the
low brightness and consequently low contrast of the
images in the set. All the images used are at an 8 bit
gray scale and 540 x 640 pixels. All tests were
performed in an IBM –compatible PC, Athlon
700MHz, 128Mb RAM memory, Windows2000
platform and MATLAB 6 software.
5 CONCLUSION
The system presented in this study is robust and
effective, but its efficacy is dependent on optimum
threshold value, which depends on illumination
levels. That suggests that the system is ideal for use
in places where illumination can be controlled, such
as shopping malls and airports, because in such
places a unique threshold value can be more easily
reached for the pictures captured. ANN is faster and
reduces significantly false detection..
ACKNOWLEDGMENT
PIBIC/CNPq and Fapesp process 97/13.709-5
financed this work.
REFERENCES
Campos, T. E, Técnicas de Seleção de Atributos e
Classificação para Reconhecimento de Faces, Msc
dissertation, IME- USP, São Paulo, 200.
Cheng,Y.D, O’Toole,A. J, Abdi,H, Classifying adults’ and
children faces by sex: computational investigations of
subcategorical feature encoding, Cognitive Science,
Elsevier, 2001.
Filho, O. M. and Neto, H. V. Processamento Digital de
Imagens. Brasport, Rio de Janeiro, 1999.
Kirby,K. and Sirovich, L, Application of the Karhunen-
Loève Procedure for the Characterization of Human
Faces, IEEE Transactions on Pattern
AnalysisandMAchine Intelligence,1990.
Lin, C. e Fan, K. Triangle-based approach to detecion of
human face. Pattern Recogniton Society, Elsevier
Science Ltd, 2001.
Psychological Image Collection at Stirling, University of
Stirling, Psycology Department,
http://pics.psych.stir.ac.uk/
, at 02/2003.
Romdham, S, Face Recognition Using Principal
Components Analysis,
http://www.robots.ox.ac.uk/~teo/pca/
, at 05/2003
Rowley, H. A.,Baluja,S. and Kanade,T., Neural Network-
Based Face Detection, IEEE, 1996.
Rowley, H. A.,Baluja,S. and Kanade,T., Rotation
Invariant Neural Network-Based Face Detection,
IEEE, 1998.
Sung, K, and Poggio, T, Exampled-Based Learning for
View-Based Human Face Detection, IEEE
Transactions on Pattern AnalysisandMAchine
Intelligence,1998.
Young, I. T., Gerbrands, J. J. e Van Vliet, L. J.
Fundamentals of Image Processing. Delft University
of Tecnology, Netherlands, 1998.
Figure 5: Result without the use of
intersection verification
ICEIS 2004 - ARTIFICIAL INTELLIGENCE AND DECISION SUPPORT SYSTEMS
468