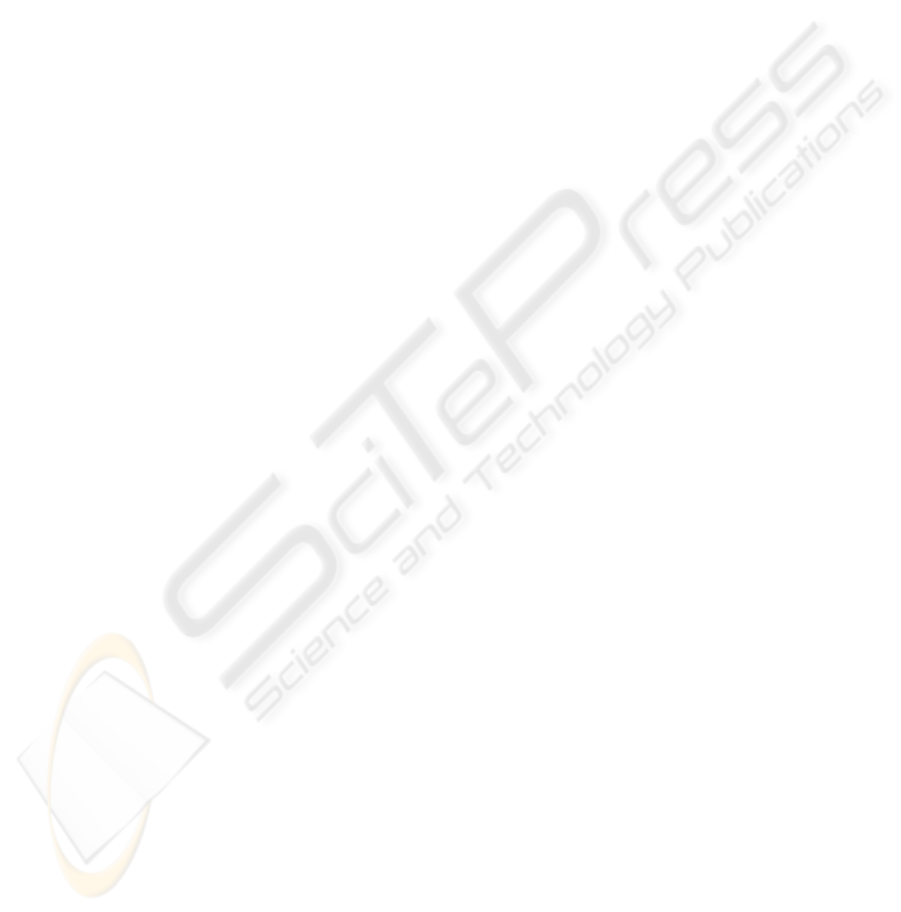
4.2.2 Fuzzy case
Observing FADs from ASDB, a CF of 0.31 is found
(Table 2). Despite of this low CF, the dependence
degree shown between HorizonT ype and OC con-
tent was more informative than in the crisp case. It
reflected better the expert knowledge. Even though,
initially, the soil scientist expected a higher degree, it
can be explained due to the influence of soils placed
at Southeast Mesoenvironment in ASDB. Indeed, due
to the arid nature of this climate, it could be expected
that one of the main soil forming factors, OC content
incorporated to soil from vegetation, were low and
homogenous. The latter conclusion can be checked
regarding Table 4. Moreover, fuzzy data mining let
us obtain a higher number of rules than in crisp case.
This supposes, quantitatively, a higher volume of dis-
covered knowledge.
A good example of correspondence or ”fusion”
between databases and expert knowledge could be
obtained comparing ARs from Sierra of G
´
ador with
Southeast ones. The former had rules with ”moder-
ate” and ”high” OC content in consequent, whereas
the latter had a ”low” value in consequent. Sierra
of G
´
ador has a higher mean altitude and mean an-
nual rainfall, and, consequently, more vegetation in
soil and horizons (especially in Ah type). Look-
ing at this, the fuzzy model reflects more accurately
soil forming processes as melanization and accumu-
lation. We can also examine others IFSDB in addi-
tion to Mesoenvironment. I.e., Protocol constitute an
important source of variability in ASDB. Comparing
”Perez” and ”Alias” categories, the former has more
ARs (Table 6) and relates more categories, reflecting
a more detailed and precise knowledge than ”Alias”.
”Perez” protocols (including field description, anal-
ysis and other techniques) seem to be more reliable
than ”Alias” ones.
5 CONCLUSIONS
We have seen how large databases can be divided into
homogeneous subsets defining one or more discrim-
inant attributes. This division, followed by a knowl-
edge discovery process, can allow us to discover pre-
viously unnoticed relations in data.
We conclude that, for this particular case, knowl-
edge extracted by means of fuzzy data mining was
more suitable to ”fusion” or comparison with ex-
pert knowledge that crisp. Moreover, fuzzy data
mining was sensitive to low support categories as
[%OrganicCarbon = Low] or [HorizonT ype =
Bk or Btk], discarded in crisp data mining.
We could confirm that fuzzy data mining is highly
sensitive to latent knowledge in ASDBs. That fact is
very important for a soil scientist, since lets us apply
it with the assurance that imprecision and uncertainty
factors (IFASDB) will not distort or alter the knowl-
edge discovery process.
As a future task, we propose to solve this same
problem in a general case. With a domain expert aid,
we must define the set of criteria for database decom-
position but also discern when fuzzy techniques get
better results than crisp ones.
REFERENCES
Agrawal, R., Imielinski, T., and Swami, A. (1993). Min-
ing association rules between sets of items in large
databases. In Proc. Of the 1993 ACM SIGMOD Con-
ference, pages 207–216.
Alias, J. (1986). Mapa de suelos de Mula. Mapa 1:100000
y memoria. LUCDEME; MAPA-ICONA-University
of Murcia.
Alias, J. (1987). Mapa de suelos de Cehegin. Mapa
1:100000 y memoria. LUCDEME; MAPA-ICONA-
University of Murcia.
Berzal, F., Blanco, I., S
´
anchez, D., Serrano, J., , and Vila,
M. (2003). A definition for fuzzy approximate depen-
dencies. Fuzzy Sets and Systems. Submitted.
Berzal, F., Blanco, I., S
´
anchez, D., and Vila, M. (2001). A
new framework to assess association rules. In Hoff-
mann, F., editor, Advances in Intelligent Data Anal-
ysis. Fourth International Symposium, IDA’01. Lec-
ture Notes in Computer Science 2189, pages 95–104.
Springer-Verlag.
Berzal, F., Blanco, I., S
´
anchez, D., and Vila, M. (2002).
Measuring the accuracy and interest of association
rules: A new framework. Intelligent Data Analysis.
An extension of (Berzal et al., 2001), submitted.
Blanco, I., Mart
´
ın-Bautista, M., S
´
anchez, D., and Vila, M.
(2000). On the support of dependencies in relational
databases: strong approximate dependencies. Data
Mining and Knowledge Discovery. Submitted.
Bosc, P. and Lietard, L. (1997). Functional dependencies
revisited under graduality and imprecision. In Annual
Meeting of NAFIPS, pages 57–62.
Bui, E. and Moran, C. (2003). A strategy to fill gaps
in soil over large spatial extents: An example from
the murray-darlin basin of australia. Geoderma, 111,
pages 21–44.
Cazemier, D., Lagacherie, P., and R., M.-C. (2001). A pos-
sibility theory approach from estimating available wa-
ter capacity from imprecise information contained in
soil databases. Geoderma, 103, pages 113–132.
Delgado, G., Delgado, R., Gamiz, E., P
´
arraga, J.,
S
´
anchez Mara
˜
non, M., Medina, J., and Mart
´
ın-Garc
´
ıa,
J. (1991). Mapa de Suelos de Vera. LUCDEME,
ICONA-Universidad de Granada.
AN EXPERIENCE IN MANAGEMENT OF IMPRECISE SOIL DATABASES BY MEANS OF FUZZY ASSOCIATION
RULES AND FUZZY APPROXIMATE DEPENDENCIES
143