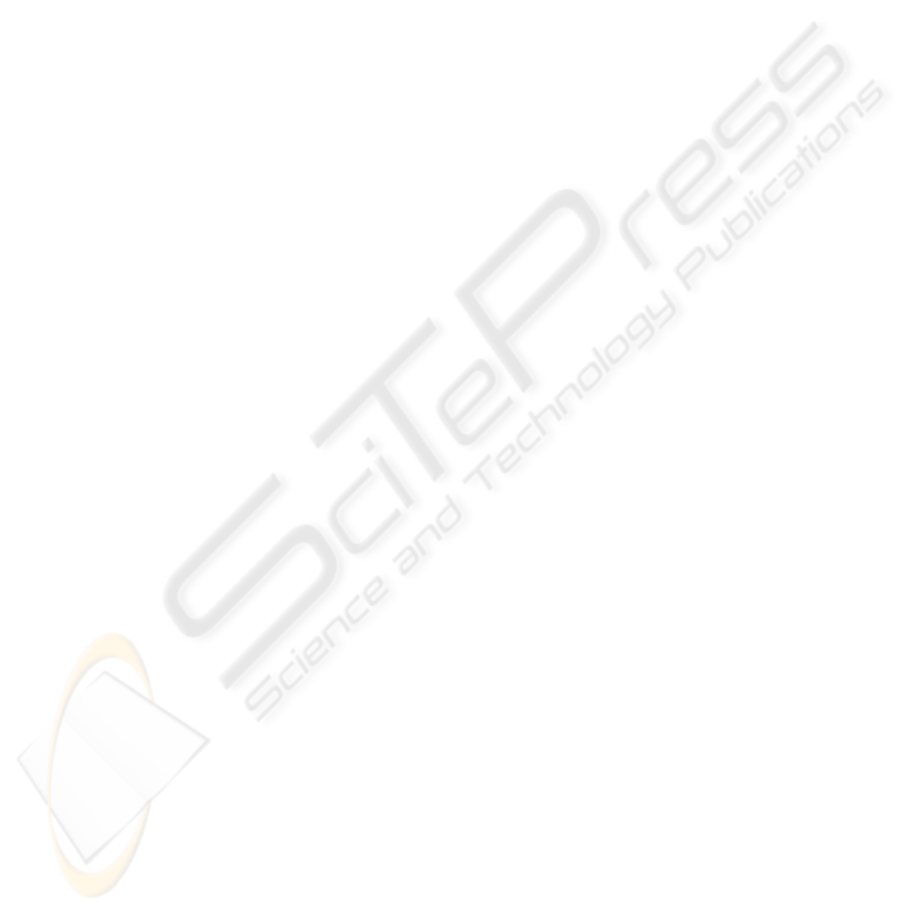
• Title of qualification awarded: Bachelor of
Science in Sociology and Psychology.
• Principal subjects/occupational skills covered:
Sociology of Risk, Sociology of Scientific
Knowledge / Information Society, E-learning and
Psychology, Research Methods.
The name and the type of organizations and the
title of qualification have to be imported from
external Information Systems or standardized using
coding schemes, in house. Subjects and occupational
skills have to be handled using two complementary
approaches: Subject Headings (or Thesaurus) and
Uncontrolled phrases. The Uncontrolled phrases
(index terms) must be given by the applicants while
the controllable terms are assigned by experienced
information officers.
Dissertation titles and the related short abstracts
form some kind of bibliographic data and can be
handled using standard procedures. However, this
approach (these handling procedures) makes sense
only in the case of a system oriented towards the
support of scientific CVs.
Skills and competence are also potential sources
of critical information but, in general, is difficult to
be handled using (even semi) automated methods.
A possible solution for the retrieval of CVs
seems to be classification based on training sets.
Hence, intuitively, we propose the use of a
document collection (collection of portions extracted
from specific CVs that is the “training” set) as a
basis. Each new document (portion of the CV) could
be seen as a query submitted for extracting “similar”
documents from the collection. The document
collection is also characterized by a number of Index
Terms (Uncontrolled Terms). For each document in
the collection the existing Index terms in the
document can have weight, frequency etc. This
approach has an advantage because such text
retrieval techniques are well known and have been
tested for many years.
Reviewing the process we can select new
documents classified and improve the document
collection (the “training” set).
Hence, for each new document we can extract
the key-phrases for possible future utilization in the
list and identify the UTs that exist in the text. Then
the vector for the document is constructed and the
similarity of the document with the documents of the
training set is calculated using the above measure.
Then a discretization table (with ranges) could
be used to propose possible Classification Codes
(CC) for the indexing of the document.
4 DISCUSSION
Main target of this paper is to discuss techniques that
could offer to the information officers the
opportunity to extract CVs and companies’ profiles
from various sources, store them in a Data Mart
system and automatically or semi-automatically
match (retrieve) the appropriate information in the
future. Hence, such cross lingual documents could
be classified by using codes contained in a Standard
Classification Scheme – SCS. The STEP list
(EKEPIS), a statistical classification list of jobs, was
used in our system. Eventually, the information
officer can focus on an information extraction
process, from various sources and documents, which
is based on phrases from a loosely controlled
vocabulary and codes from a specific SCS.
The proposed method is based on the following
process:
1) A set of documents is formed by extracting
(and storing) documents from various sources. These
documents play the role of a “training” set for the
whole Corpus of the documents in the future.
2) The traditional IR process is applied as a first
step to automatically or semi - automatically focus
on some information extraction; in other words to
extract potential terms describing documents.
3) The creation of an authority list follows. All
the extracted UTs are submitted to the information
officer(s) to select the appropriate ones to
characterize the documents.
4) A Study of the distribution of UTs in the
corpus of the documents can be conducted to form
the "training set".
5) A vector is created for each document of the
training set. Each item (of the vector) has two
possible values (true, false) representing the
existence or not of the corresponding UT in the
document. The last item of each vector has the
classification code (e.g. the STEP code) that
characterizes the document (the class of document).
Hence, there are some critical points for the
method:
• Extract key-phrases. As an example, we can
adapt a method (e.g. Naïve Bayes technique)
used in (Witten, 1977) for selecting key-phrases
from a new document.
• Using the extracted phrases from the text the
experts can form a list of Uncontrolled Terms.
• The STEP list could be used for the indexing of
documents.
• A Knowledge Discovery “mapping” between
UTs and classification codes must be supported.
INFORMATION SYSTEM FOR SUPPORTING THE INCLUSION OF JOB SEEKERS TO THE LABOUR MARKET.
621