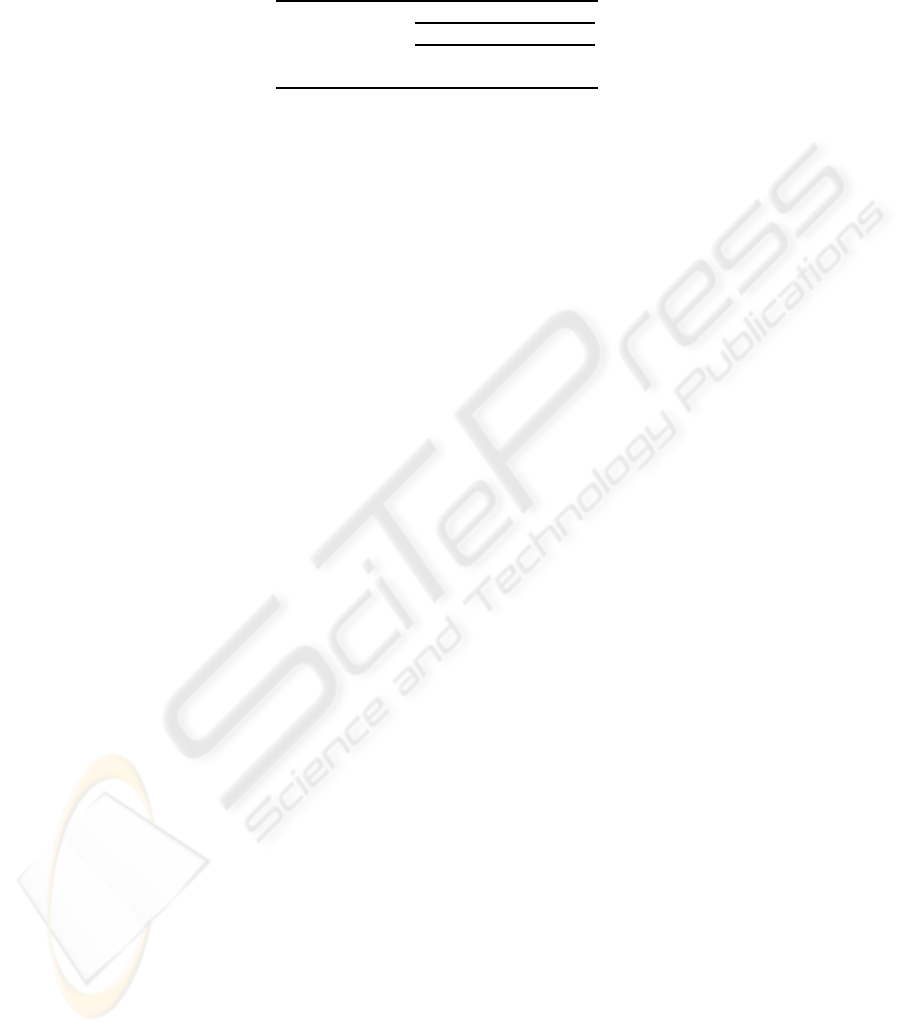
No. of retrieved images
Method
MDD Mixture
Gaussian Mixture
16 48 64 80 96
0.23 0.71 0.92 0.94 0.96
0.21 0.68 0.82 0.92 0.93
Table 5. Recall obtained for the texture database.
in a lot of other applications which involve discrete data or cooccurrence matrices such
as textmining, Webmining and E-mail segmentation.
Acknowledgment
The completion of this research was made possible thanks to Bell Canada’s support
through its Bell University Laboratories R&D program.
References
1. M. Tuceryan and A. K. Jain, Texture Analysis, The Handbook of Pattern Recognition and
Computer Vision, pp. 207-248, 1998.
2. R. M. Haralick, K. Shanmugan, and I. Dinstein Textural features for image classification,
IEEE Tr ansactions on Systems, Man and Cybernetics, vol. 8, pp. 610-621, 1973.
3. M. Unser, Sum and Difference Histograms for Texture Classification, IEEE Tr ansactions on
Pattern Recognition and Machine Intelligence, vol. 8, No. 1, pp. 118-125, 1986.
4. N. Bouguila, D. Ziou and J. Vaillancourt, Novel Mixtures Based on the Dirichlet Distribution:
Application to Data and Image Classification , IAPR International Conference on Machine
Learning and Data Mining, lNCS2734, pp. 172-181, 2003.
5. R. A. Redner and H. F. Walker, Mixture Densities, Maximum Likelihood and EM Algorithm
, SIAM Review, vol. 26, No. 2, pp. 195-239, 1984.
6. A. P. Dempster, N. M. Laird and D. B. Rubin, Maximum Likelihood from Incomplete Data
via the EM Algorithm , Journal of the Royal Statistical Society, B, vol. 39, pp. 1-38, 1977.
7. G. Schwarz, Estimating the Dimension of a Model , The Annals of Statistics, vol. 6, No. 2, pp.
461-464, 1978.
8. H. Akaike, A New Look at the Statistical Model Identification , IEEE Transactions on Auto-
matic Control, vol. AC-19, No. 6, pp. 716-723, 1974.
9. J. Rissanen, Modeling by Shortest Data Description , Biometrika, vol. 14, pp. 465-471, 1978.
10. S. Medasani and R. Krishnapuram, Categorization of Image Databases for Efficient Retrieval
Using Robust Mixture Decomposition, Computer Vision and Image Understanding, vol. 83,
pp. 216-235, 2001.
11. C. R. Rao, Advanced Statistical Methods in Biomedical Research, New York: John Wiley
and Sons, 1952
12. N. Bouguila, D. Ziou and J. Vaillancourt, Probabilistic Multimedia Summarizing Based on
the Generalized Dirichlet Mixture, Supplementary Proceedings of ICANN/ICONIP 2003,pp
150-154, 2003.
13. J. C. Bezdek, Pattern Recognition with Fuzzy Objective Function Algorithms, Plenum Press,
New York, 1981.
14. A. E. Raftery and J. D. Banfield, Model-Based Gaussian and Non-Gaussian Clustering Bio-
metrics, vol. 49, pp. 803-821, 1993.
127