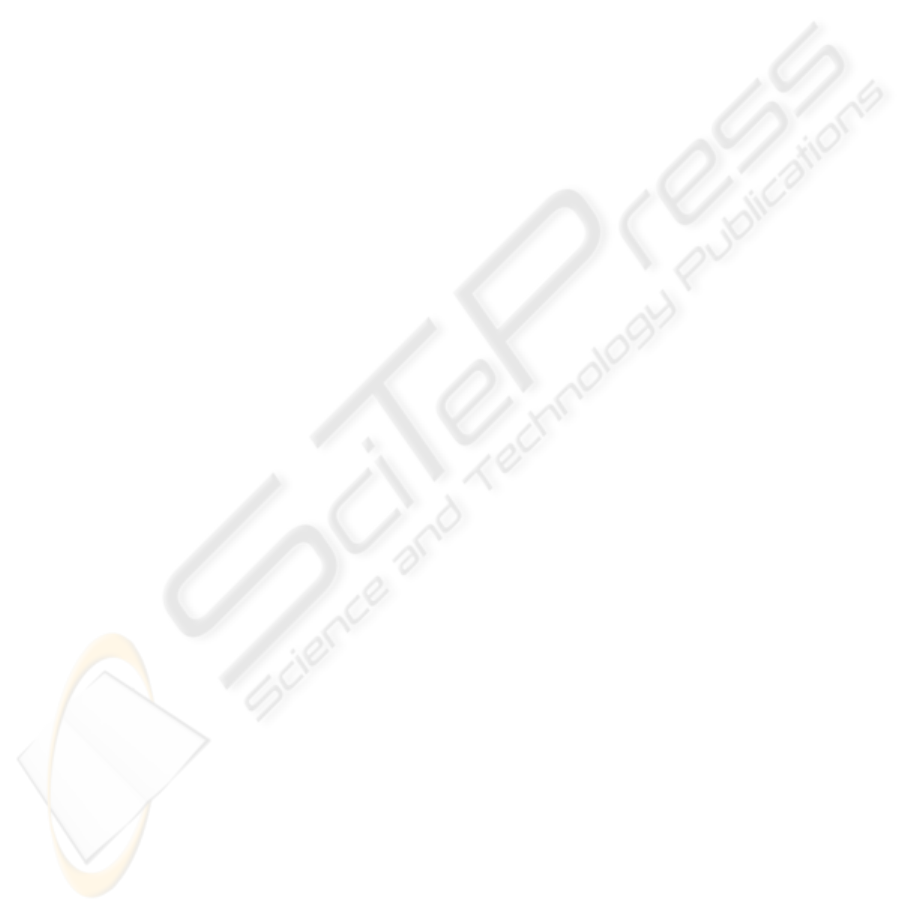
variety of types of data available—time series, spatial, multimedia, worldwide web
logs, etc. Using online learning algorithms on these different types of data is an im-
portant area of future work. Moreover, the used combination strategy is based on
voting method. In a future work, apart from voting, it might be worth to try other
combination rules to find the regularity between the combination strategy, individual
classifiers and the datasets.
References
1. Aha, D., Lazy Learning. Dordrecht: Kluwer Academic Publishers (1997).
2. Auer P. & Warmuth M., Tracking the Best Disjunction, Machine Learning 32 (1998) 127–
150, Kluwer Academic Publishers.
3. Bauer, E. & Kohavi, R., An empirical comparison of voting classification algorithms:
Bagging, boosting, and variants. Machine Learning 36 (1999) 105–139.
4. Blake, C.L. & Merz, C.J, UCI Repository of machine learning databases. Irvine, CA:
University of California, Department of Information and Computer Science (1998):
[http://www.ics.uci.edu/~mlearn/MLRepository.html]
5. Cohen W., Fast Effective Rule Induction. In Proc. of Int Conf. of ML-95 (1995). 115-123.
6. Domingos P. & Pazzani M., On the optimality of the simple Bayesian classifier under
zero-one loss. Machine Learning, 29 (1997) 103-130.
7. Fan W., Stolfo S., and Zhang J., The application of AdaBoost for distributed, scalable and
on-line learning, in Proceedings of the Fifth ACM SIGKDD International Conference on
Knowledge Discovery and Data Mining. New York, NY: ACM Press, 1999, pp. 362-366.
8. Fayyad U., and Irani K., Multi-interval discretization of continuous-valued attributes for
classification learning. In Proc. of the 13
th
Int. Joint Conference on AI (1993) 1022-1027.
9. Fern, A., & Givan, R., Online ensemble learning: An empirical study. In Proceedings of
the Seventeenth International Conference on ML (2000) 279–286. Morgan Kaufmann.
10. Freund Y., Schapire R., Large Margin Classification Using the Perceptron Algorithm,
Machine Learning 37 (1999) 277–296, Kluwer Academic Publishers.
11. Littlestone N. & Warmuth M., The weighted majority algorithm. Information and Compu-
tation 108 (1994) 212–261.
12. Mitchell, T., Machine Learning. McGraw Hill (1997).
13. Oza, N. C. and Russell, S., Online Bagging and Boosting." In Artificial Intelligence and
Statistics 2001, eds. T. Richardson and T. Jaakkola, 105-112.
14. Quinlan J.R., C4.5: Programs for machine learning. Morgan Kaufmann, San Francisco
(1993).
15. Saad, D., Online learning in neural networks, London, Cambridge University Press
(1998).
16. Salzberg, S., On Comparing Classifiers: Pitfalls to Avoid and a Recommended Approach,
Data Mining and Knowledge Discovery 1 (1997) 317–328.
17. Schaffer, C., Selecting a classification method by cross-validation. Machine Learning 13
(1993) 135-143.
18. Utgoff, P., Berkman, N., & Clouse, J., Decision tree induction based on efficient tree
restructuring. Machine Learning, 29 (1997) 5–44.
19. Widmer G. and Kubat M., Learning in the presence of concept drift and hidden contexts.
Machine Learning 23 (1996) 69–101.
20. Witten I. & Frank E., Data Mining: Practical Machine Learning Tools and Techniques
with Java Implementations, Morgan Kaufmann, San Mateo (2000).
68