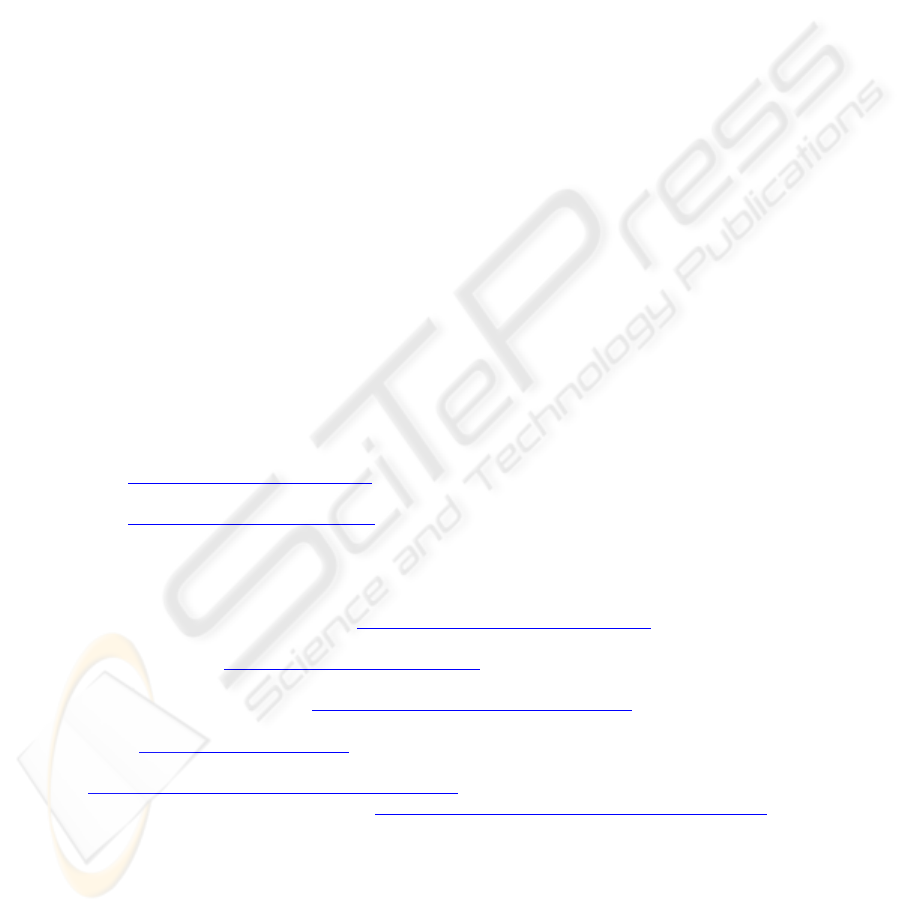
Conclusions
After the more reliable Test II, we confirm the conclusions of Test I, our approach has
better accuracy and speed than original 5x16 static matrix size crm114. We also
extract more conclusions, the most important is that the ZERO false positives are
within reach if we use relearning on false cases in a planned way, and we have
proposed a valid tactic in two phases for this aim. An other conclusion is we can give
more important to the market critical parameter "false positives", using bigger ham
corpus size than the spam one, to make the maps (.css); but this decrease the other
false cases, the negative, the spam and ham maps (and they train corpus) are an
antinomy with absolute dependencies one to each other for the final results. We also
observed that a ham subdivision in hard an easy may be good for planing the train and
relearning tactic, we have to considerer that hard ham is not very used, but are those
mails that could be easy mistaken with spam, even for the human eye, so depending
on corporative domain we should specially train the maps for them, or not. Finally we
have shown that not natural map-working domain, are not valid at all.
References
[1] Tom M. Mitchell. Machine Learning - McGraw-Hill, ISBN: 0-07-042807-7
[2] William S. Yerazunis. Sparse Binary Polynomial Hashing and the CRM114 Discriminator -
MER Labs. Cambridge, MA. 2003 and Cambridge Spam Conference Proceeding -
http://crm114.sourceforge.net/
[3] Paul Graham. A Plan for Spam. 2003 Cambridge Spam Conference Proceeding
http://paulgraham.com/spam.html
[4] Paul Graham. Better Bayesian Filtering. 2003 Cambridge Spam Conference Proceeding
http://paulgraham.com/better.html
[5] Jason D.M. Rennie, y Tommie Jaakkola. Automatic Featured Induction for Text
Classification. - MIT, AI Labs. Abstract Book. 2002 and 2003 Spam Conference-
http://www.ai.mit.edu/~jrennie/spamconference/
[6] Matt Sergeant. Internet Level Spam Detection and SpamAssassin 2.50.- 2003 Cambridge
Spam Conference Proceeding -
http://axkit.org/docs/presentations/spam/
[7] Teodor Zlatanov. Spam Analisys in Gnus with spam. - 2003 Cambridge Spam Conference
Proceeding - http://lifelogs.com/spam/spam.html
[8] Brian Burton. SpamProbe: Bayesian Spam Filtering Tweaks - 2003 Cambridge Spam
Conference Proceeding -
http://spamprobe.sourceforge.net/index.html
[9] John Graham The spammers compendium.- 2003 Cambridge Spam Conference Proceeding
-
http://popfile.sourceforge.net
[10] Kristian Eide. Winning the War on spam: Comparison of Bayesian spam filters. 2003.
http://home.dataparty.no/kristian/reviews/bayesian/
[11] Unam public spam set 2002-2003: http://www.seguridad.unam.mx/Servicios/spam/spam/
[12] From call for donations for this specific use, at the Universidad Carlos III de Madrid,
2003.
[13] Personal communication with Juan Carlos Martin, Security and Network Manager of
EspacioIT, which has over 3.000 mail users along different domains and mail servers.
October, 2003
[14] Carreras & Marquez. Boosting Trees for Antispam Email Filtering. 2001 TLAP Research
Center. LSI Department. Universitat Politecnica de Catalunya.
215