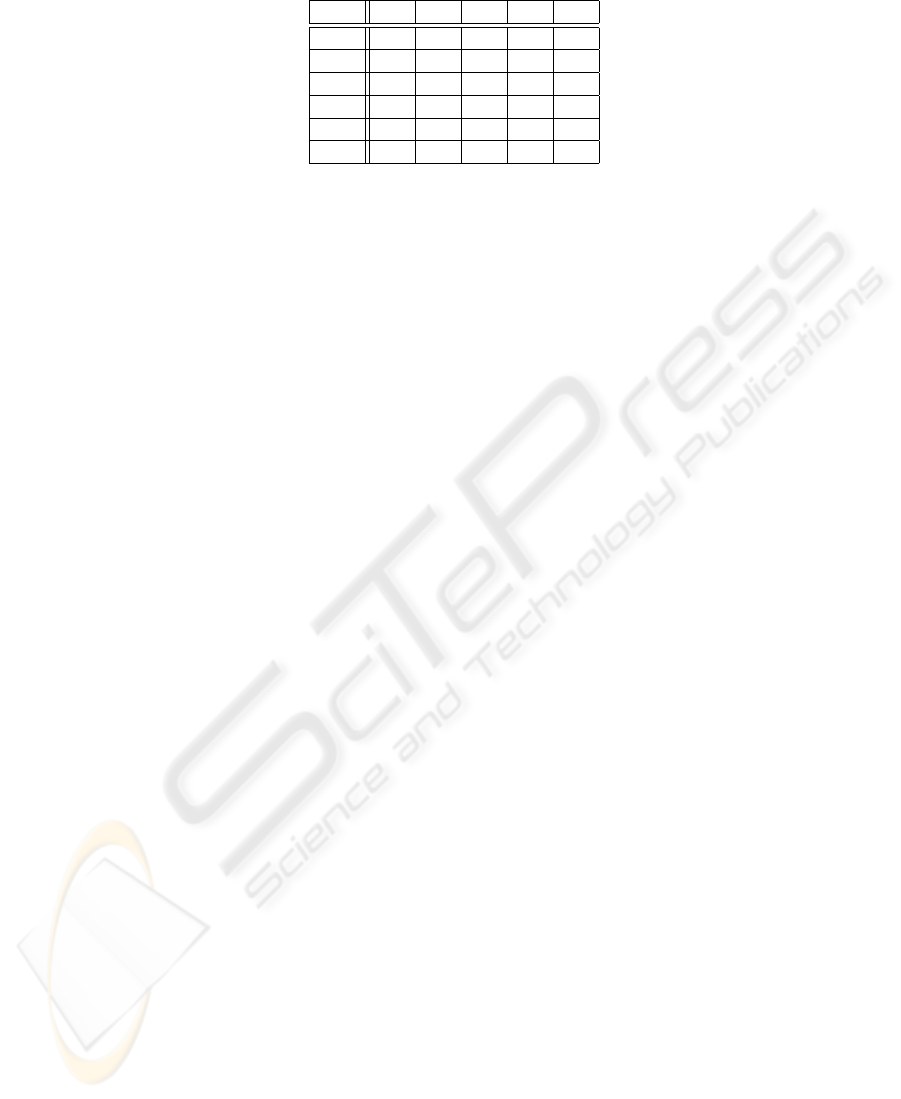
Set1 Set2 Set3 Set4 Set5
SA 10.95 10.35 10.35 10.00 10.05
W A 10.50 10.35 10.45 9.90 10.00
AHP
1
10.05 9.95 10.05 9.60 9.30
AHP
2
10.00 9.70 9.45 9.50 9.50
AHP
3
9.80 9.50 9.50 9.20 9.45
AHP
4
9.90 9.55 9.75 9.30 9.50
Table 5. Classification errors [%] on the Satimage database. (Error without combination: 12.05%)
5 Conclusion
In this paper we proposed a new linear combination method, and compared its per-
formance with those of other combiners. As shown in the experiments, AHP-based
combinations proved an effective generalization of the weighted averaging rule; they
outperformed the other averaging methods in almost every case.
Finally, we should mention that resampling techniques may further improve the
performance of linear combinations, hence it is worth investigating generalizations of
the bootstrapped learners Bagging [11] and Boosting [12] in future work.
References
1. Anil K. Jain, Robert P. W. Duin, and Jianchang Mao. Statistical pattern recognition: A review.
IEEE Transactions on Pattern Analysis and Machine Intelligence, 22(1):4–37, 2000.
2. V. N. Vapnik. Statistical Learning Theory. John Wiley and Son, 1998.
3. R. O. Duda, P. E. Hart, and D. G. Stork. Pattern Classification. John Wiley and Son, New
York, 2001.
4. L. Xu, A. Krzyzak, and C.Y. Suen. Method of combining multiple classifiers and their
application to handwritten numeral recognition. IEEE Trans. on SMC, 22(3):418–435, 1992.
5. K. Tumer and J. Ghosh. Analysis of decision boundaries in linearly combined neural classi-
fiers. Pattern Recognition, 29:341–348, 1996.
6. F. Roli and G. Fumera. Analysis of linear and order statistics combiners for fusion of imbal-
anced classifiers. In 3rd Int. Workshop on Multiple Classifier Systems (MCS 2002), Cagliari,
Italy, June 2002. Springer-Verlag, LNCS.
7. M. P. Perrone and L. N. Cooper. When networks disagree: Ensemble methods for hybrid neu-
ral networks. In R. J. Mammone, editor, Neural Networks for Speech and Image Processing,
pages 126–142. Chapman-Hall, 1993.
8. T. L. Saaty. The Analytic Hierarchy Process. McGraw-Hill, New York, 1980.
9. A. Kocsor and L. T
´
oth. Kernel-based feature extraction with a speech technology application.
In IEEE TRANS. ON SIGNAL PROCOCESSING, 2003.
10. G. Fumera and F. Roli. Linear combiners for classifier fusion: Some theoretical and experi-
mental results. In 4th Int. Workshop on Multiple Classifier Systems (MCS 2003), Guildford,
UK, January 2003. Springer-Verlag, LNCS.
11. Leo Breiman. Bagging predictors. Machine Learning, 24(2):123–140, 1996.
12. Freund. Boosting a weak learning algorithm by majority. In Proceedings of the Workshop
on Computational Learning Theory (COLT 1990). Morgan Kaufmann Publishers, 1990.
58