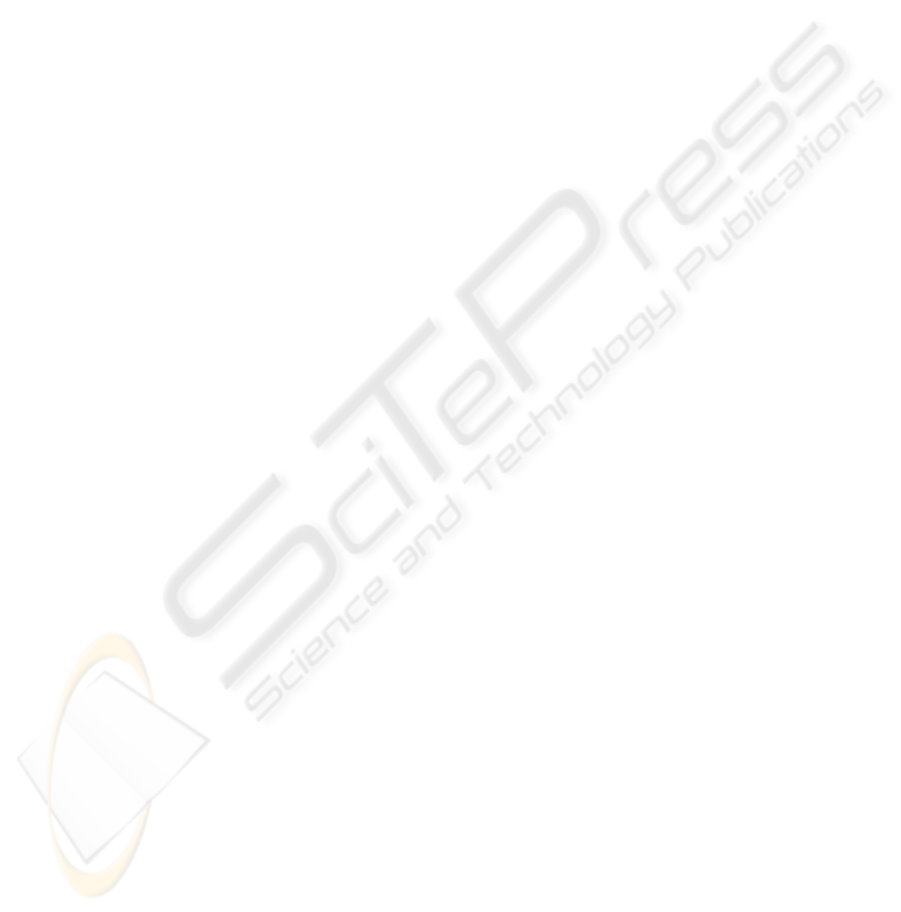
overall results. As for the latter, we could contemplate just simpler extensions,
helping, for instance, to determine the correct word sense. These could substitute the
word attributes used here.
Alternatively, we could try to categorize the given words (or groups of words) into
semantic categories. We could continue these extensions by trying to establish
relationships between different categories (e.g. relate an object of an action with some
agent and the action itself). This could give rise to new attributes (or predicates) that
could be exploited in learning.
From a practical standpoint it would be useful to exploit, in the first place,
semantic networks like WordNet for the Portuguese language. It would also be useful
to make use of existing ontologies developed by others. These could be exploited to
obtain generalizations not only for the focus element, but also in the right and left
contexts.
Despite the fact that many improvements could be done, our work shows that even
a relatively simple system could already be useful to carry out rather complex
extraction tasks.
Acknowledgements
The authors wish to acknowledge the support provided by FCT (Fundação para a
Ciência e Tecnologia) under so called Pluriannual Programme attributed to LIACC,
and the funding received from POSI/POCTI.
References
1. Shadbolt N., Caught up in the web, Invited talk at the 13th Int. Conf. on Knowledge
Engineering and Knowledge Manangement (EKWA02) (2002)
2. Kushmerick N., Wrapper induction: efficiency and expressiveness, Elsevier (2000), 15-68
3. Sitter A., W. Daelemans, Information Extraction via Double Classification, in Proceedings
of the Int. Workshop on Adaptive Text Extraction and Mining (C.Ciravegna and
N.Kushmerick, eds.), associated with ECML/PKDD-2003 Conf., Dubrovnik, Croatia,
(2003)
4. Mladenic D., M. Grobelnik, Feature selection for unbalanced class distribution and Naive
Bayes, in Machine Learning: Proceedings of the Sixtheenth International Conference
(ICML'99), Morgan Kaufmann (1999)
5. Mitchell T. M., Machine Learning, McGraw-Hill (1997)
6. Witten Ian, E. Frank, Data Mining: Practical Machine Learning Tools and Techniques with
Java Implementations, Morgan Kaufmann (2000)
7. Craven M., D. DiPasquo, D. Freitag, A. McCallum, T. Mitchell, K. Nigam and S. Slatery,
Learning to construct knowledge bases from the World Wide Web, Elsevier (2000), 69-113
8. Winston P. H., Artificial Intelligence, Addison-Wesley (1992)
9. Quinlan J. R., C5.0 Data Mining Tool,
www.rulequest.com (1997)
10. Meadow T. Charles, B. R. Boyce, D.H. Kraft, Text Information Retrieval Systems, 2nd ed.,
Academic Press (2000)
138