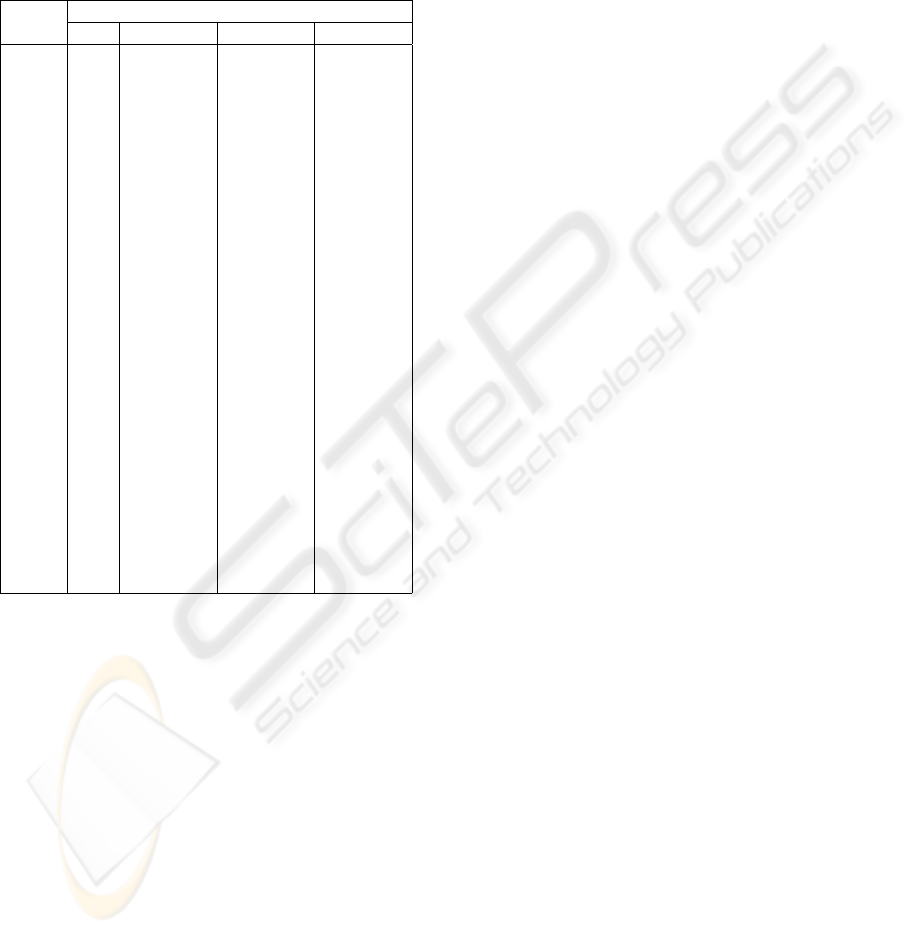
cipal Component Analysis explained 96.2% of the to-
tal variance of the data. A look at the correlation ma-
trix for the four main factors and the variables (Figure
8) shows all positive correlations in the column for
the first component, indicating a non-periodic com-
ponent, whereas for example the pattern of the corre-
lation coefficients for the second component shows a
periodic component.
Component
time t 1 2 3 4
0 .762 -.261 .452 -.276
-2 .777 -.437 .350 -.186
-4 .791 -.543 .160 -.120
-6 .804 -.551 -7.047E-02 -9.277E-02
-8 .815 -.455 -.284 -.113
-10 .825 -.278 -.426 -.174
-12 .833 -5.918E-02 -.462 -.253
-14 .840 .150 -.382 -.323
-16 .846 .303 -.205 -.354
-18 .851 .363 2.348E-02 -.332
-20 .854 .319 .247 -.256
-22 .856 .186 .410 -.139
-24 .857 8.896E-04 .470 4.746E-04
-26 .856 -.185 .411 .140
-28 .854 -.319 .248 .257
-30 .850 -.364 2.372E-02 .333
-32 .845 -.305 -.206 .354
-34 .840 -.153 -.383 .322
-36 .833 5.687E-02 -.464 .253
-38 .824 .276 -.429 .173
-40 .814 .455 -.287 .113
-42 .803 .551 -7.393E-02 9.279E-02
-44 .790 .545 .157 .120
-46 .776 .440 .348 .187
-48 .760 .265 .451 .277
Figure 8: Correlation matrix for the four main components
for Bob Hall Pier.
For the deep water station Flower Garden, five
main components explain 93.8% of the total variance
of the data. The first two components for Flower
Garden are periodic and the third component has all
positive correlation coefficients, indicating that it is a
non-periodic component. We found that at each sta-
tion tested, no more than 5 factors explain over 90%
of the variance for water levels. The periodic main
components could be called “astronomical”, the non-
periodic component that shows mainly in shallow wa-
ters could be called “weather”. For all stations in the
coastal shallow waters and estuaries we found the first
component to be not periodical; hereweather is an im-
portant input for predictions. The other components
are periodical. For the stations on off-shore deep wa-
ters the first two components are astronomical or pe-
riodical.
These conclusions assisted us in improving pre-
dictions in the shallow waters since the conclusion
suggested integrating a regression approach with har-
monic analysis. Namely, we use the idea that vari-
ations of water levels depend on two components -
a harmonic component (tides) and another compo-
nent which is affected by weather. The regression ap-
proach incorporates recent weather and the harmonic
analysis parts accounts for the astronomical compo-
nents. We separate out the weather part as follows:
Let us denote
x
n
= w
n
− h
n
where x
n
is the difference between the actual water
level w
n
and the harmonic forecast h
n
at the moment
n. Then we can apply a bootstrapping technique to
find the next value of x from the water levels of the
preceding n hours. That is, we can predict next hour
difference between water level and harmonic level
x
1
= a
0
x
0
+ a
−1
x
−1
+ . . . + a
−n
x
−n
and step by step
x
k
= a
0
x
k−1
+ a
1
x
k−2
+ . . . + a
−n
x
k−n
.
Now we find the prediction for water levels as by
adding the harmonic forecast h
t
to the forecasted dif-
ference x
t
w
t
= h
t
+ x
t
.
This symbiosis of regression and harmonic analysis
approach to the predictions of water levels proved to
be very effective.
5 MODEL PERFORMANCE
ASSESSMENT
Once water level predictions are developed,
they are evaluated based on a suite of Na-
tional Ocean Service Skill Assessment Statistics.
Error predicted value − observed value
SM series mean
RMSE root mean square error
SD standard deviation
CF(X) central frequency;
% of errors between −X and X
POF(2X) positive outlier frequency
NOF(2X) negative outlier frequency
MDPO(2X) max. duration of positive outlier
MDNO(2X) max. duration of negative outlier
A web-based tool (see Figure 9) was created that
combines harmonic analysis with multivariate sta-
tistical modeling to predict water levels along the
Texas Gulf Coast. Water level predictions, as well
as skill assessment statistics, are dynamically gener-
ated based on a set of user given criteria including
station identifier, dates, number of coefficients (that
REAL TIME WEB AVAILABILITY OF STATISTICAL MODELS FOR WATER LEVELS ALONG THE TEXAS
COASTLINE
221