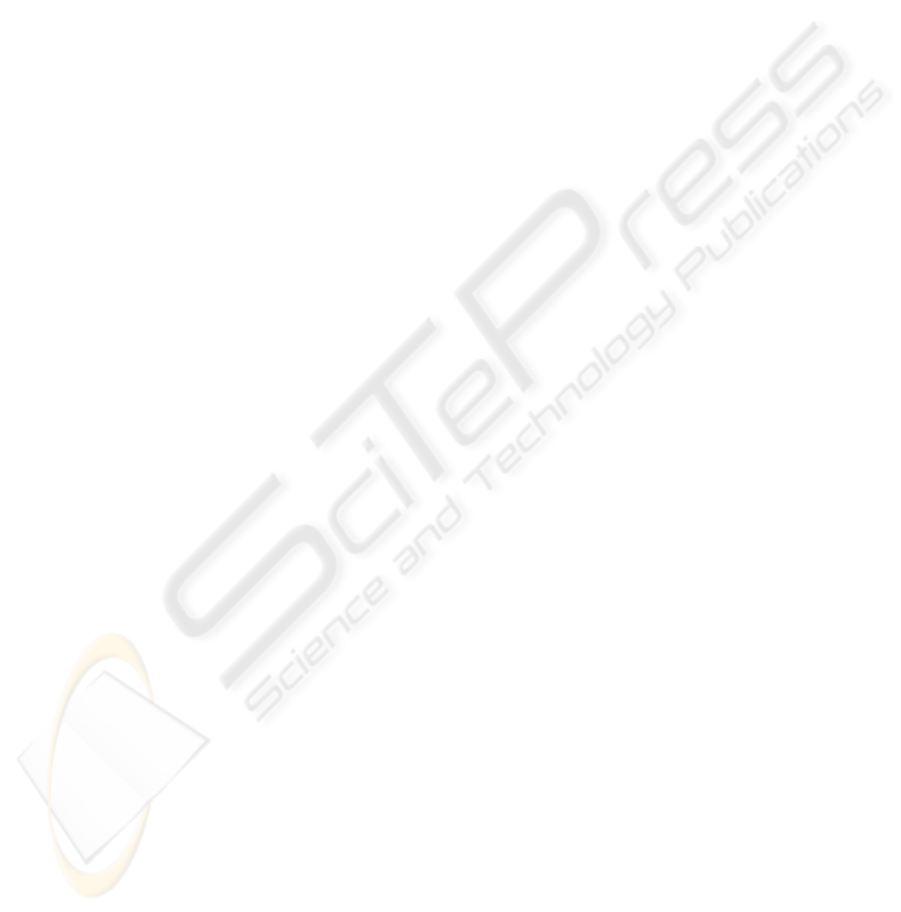
One can easily propose tens of different specific
realizations of each of the three functionals which
satisfy the required conditions. Hence, without much
effort, we get thousands of new features which are
invariant or sensitive to image motion. This only
proves the value of the considered theory for the
problems of pattern recognition with multiple
structure of the classes, like the problem of the
recognition of hieroglyphs or textures. In the
following, some functionals are presented which we
use to solve different problems and which are
implemented on a PC.
a) Invariant functionals:
1. Maximum value of the function.
2. The number of extrema of the function.
3. Total variation of the function.
4. Standard Euclidean norm of the function.
5. Any functional determined by the distribution
of the values of the function.
b) Sensitive functionals:
1. Standard center of gravity of the masses
calculated by formula (3).
2. The phase of the second Fourier harmonics of
the function.
3. Absolute value of the Fourier coefficient of
the third harmonics.
4. Probability-theoretic median.
6 CONCLUSION
Trace-transform has been considered, the latter
applying all-direction scanning.
The most valuable property of the Trace-
transform is that it establishes the new class of
recognition features with a triple structure (triple
features).
It is possible to form great many features (in fact,
thousands of them), as it needs to recognize patterns
with a great number of classes. A vast number of
features helps to understand image better and to
increase recognition flexibility and fidelity.
Besides, it has been established that the theory
based on triple features yields a stable result when
analyzing biological micro and nano objects, and, in
particular, when recognizing images in the field of
nanotechnology. Results have been obtained in
successful recognition of leucocytes and
erythrocytes, which саn be found in (Fedotov et al.,
2000). Moreover, the theory of triple features stated
above has been tested on and proved suitability for
technical diagnostics and flaw detection problems.
Applicability of the given ideas has been
experimentally proved in (Fedotov et al., 2002).
The theory created allows us to obtain features
independent of object motions or linear
deformations. Additionally, we can use the theory to
obtain features which depend on the transformations
mentioned above in a simple way giving us a
possibility to compute the parameters of motions and
motions or linear deformations transformed objects
(it can be helpful in machine vision system for exact
positioning of robot tools).
A composition of three functionals is applicable
at the same time to construct recognition features
and to perform non-linear filtration, to reduce noise
within the image and to segment the objects, to
smooth the image etc. It should be noted that one
could enhance non-linear filtration applying the
filtering capabilities of both the Trace-functional and
another functional of triple structure. Having the
opportunity to construct features and to perform
image pre-processing simultaneously and by the
same technique, one can make recognition system
faster, pre-processing and feature construction being
realized at the same stage of the scanning.
REFERENCES
Fedotov N.G., 1990. Metody Stokhasticheskoi Geometrii
v Raspoznavanii Obrazov (Methods of Stochastic
Geometry in Patters Recognition), Moscow, Radio i
Svjaz.
Fedotov N.G., Kadyrov A.A., 1995. Image scanning in
machine vision leads to new understanding of image.
In Proc. of 5th International Workshop on Digital In
Processing and Computer Graphics, Proc. International
Society for Optical Engineering (SPIE), Vol. 2363,
pp. 256-261.
Helgason S., 1980. The Radon Transform, Birkhauser,
Basel and Boston, Massachusetts.
Fedotov N.G., Shulga L.A., 2000. New Theory of Pattern
Recognition on the Basis of Stochastic Geometry. In
WSCG'2000 Conference Proceedings, Vol. 1, р. 373-
380.
Fedotov N.G., Shulga L.A., 2004. Feature Generation and
Stochastic Geometry. In Proc. of the 4th International
Workshop on Pattern Recognition in Information
Systems, PRIS’2004, Porto, Portugal, p. 169-175.
Fedotov N.G., Shul’ga L.A., Roy A.V., 2005. Visual
Mining for Biometrical System Based on Stochastic
Geometry Pattern Recognition and Image Analysis,
Vol. 15, No. 2, 2005, pp. 389–392.
Fedotov N.G., Nikiforova T.V., 2002. Technical
defectoscopy based on new pattern recognition theory,
Izmeritel’naya tekhnika (Measurement technics), 2002,
No. 12, pp. 27-31.
ICINCO 2005 - ROBOTICS AND AUTOMATION
192