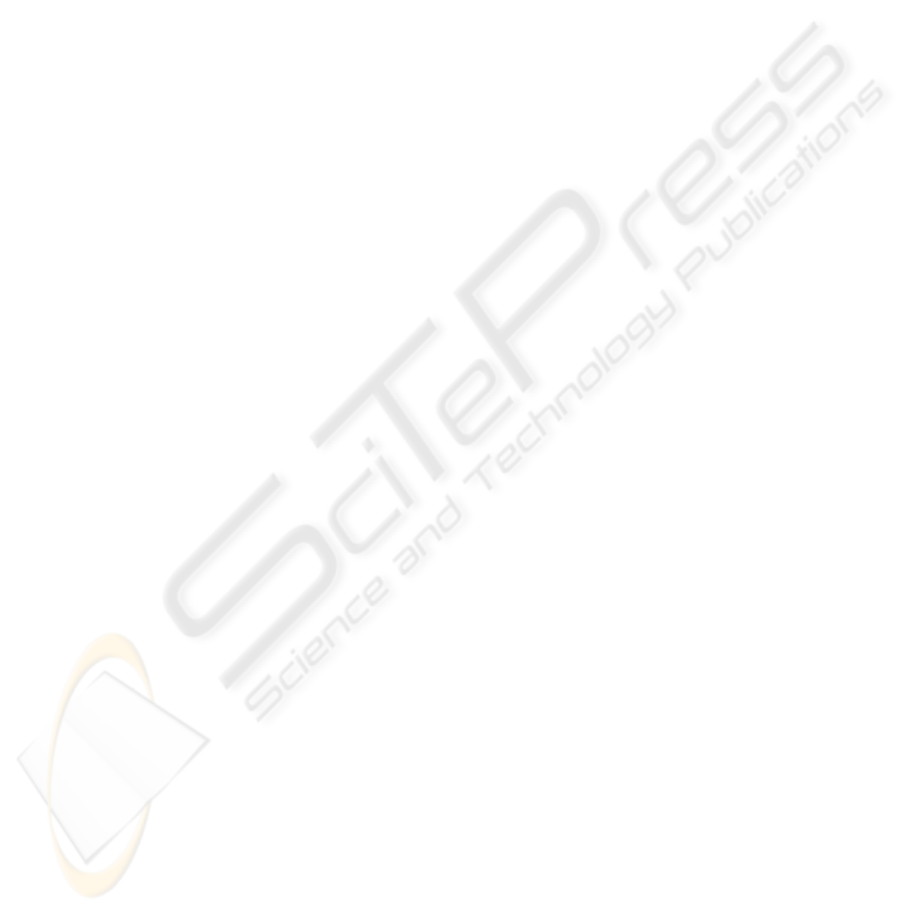
hand, calculation times with the state of art PC are
also reasonable.
Although the presented approach proceeds
systematically, some expertise is useful defining the
correct boundaries for calculating parameters, for
example, data window sizes. If limits are set too
wide, calculation times may increase exponentially.
In this phase, there is not any automated procedure
to select best model candidates from result table and
requires also expertise. This is mainly because the
selection of best model candidates requires also
visual inspection of the model behaviour and is
therefore difficult to automate. In the future, the
approach will be developed more into fully
automated way.
The presented approach can provide successful
results, even if data pre-processing or outlier
removal has failed. This is because data survey can
help to choose data segments containing only
relevant information. Thus, the need for data survey
is evident.
5 CONCLUSIONS
In this paper, a systematic approach for data survey
was presented and applied to wood loss data.
Model candidates using simple dynamic ARX –
models were constructed systematically with
different input combinations, window sizes and data
ranges. The target was to find out the best data sets
for further modelling. Model candidates working
best with validation data were stored and tested with
independent data.
Main process interactions and delays were easily
discovered from structures of the interpretable linear
model candidates. The analysis can thus provide
valuable information also for the model structure
selection. This shows the importance of proper data
survey. It is also one kind of data mining stage: with
the proper data survey, best inputs, correct
interactions between variables and optimal data
window sizes could be found even with linear
modelling methods. Data survey also provides
information about model degrees and delays. This
kind of knowledge discovery is an important step in
process control development.
REFERENCES
Abonyi, J., R. Babuška and B. Feil, 2003. Structure
selection for nonlinear input-output models based on
fuzzy cluster analysis. IEEE International Conference
on Fuzzy Systems, v 1, pp. 464-469
Anctil, F., C. Perrin and V. Andréassian, 2004. Impact of
the length of observed records on the performance of
ANN and conceptual parsimonious rainfall-runoff
forecasting models, Environmental Modelling &
Software, 19, pp. 357-368.
Isokangas A. and K. Leiviskä, 2005. Minimising wood
losses of drum debarking. Accepted to Paper and
Timber magazine.
Kocjančič R. and J. Zupan, 2000. Modelling of the river
flowrate: the influence of the training set selection,
Chemometrics and Intelligent Laboratory Systems, 54,
pp. 21-34.
Linkens, D.A. and M.-Y. Chen, 1999. Input selection and
partition validation for fuzzy modelling using neural
network. Fuzzy Sets and Systems, 107, pp. 299-308.
Ljung, L., 1999. System identification: theory for the use.
Prentice hall, Englewood cliffs, NJ.
Luo, W. and S.A. Billings, 1995. Adaptive model
selection and estimation for nonlinear systems using a
sliding data window, Signal Processing, 46, pp. 179-
202.
Mendes, E.M.A.M and S.A. Billings, 2001. An alternative
solution to the model structure selection problem.
IEEE Transactions on Systems, Man, and Cybernetics
Part A: Systems and Humans, 31, pp. 597-608.
Näsi, J., A. Isokangas and E. Juuso, 2001. Klusterointi
kuorimon puuhäviöiden mallintamisessa. ISBN 951-
42-5894-0.
Prudêncio, R.B.C., Ludermir T.B. and de Carvalho F.A.T.,
2004. A Modal Symbolic Classifier for selecting time
series models, Pattern Recognition Letters, 25, pp.
911-921.
Pyle, D., 1999. Data Preparation for Data Mining.
Morgan Kaufmann, San Francisco, California.
Simon, G., J Schoukens and Y. Rolain, 2000. Automatic
model selection for linear time invariant systems,
Proceedings of the12th IFAC Symposium on System
Identification, SYSID, Santa Barbara, CA, USA, 21-
23 June 2000, Vol. I., pp. 379-384.
Šindelář, R., 2004. Input selection for fuzzy modelling.
Proceedings of the 2nd IFAC Workshop on Advanced
Fuzzy/Neural Control, Oulu, Finland, pp. 13-18.
Sugeno, M. and G. Kang, 1988. Structure identification of
fuzzy model. Fuzzy Sets and Systems, 28, pp. 15-33.
Söderström, T. and P. Stoica, 1988. System identification,
Englewood cliffs, NJ. Prentice Hall.
SYSTEMATIC APPROACH TO MODEL-BASED DATA SURVEY
65