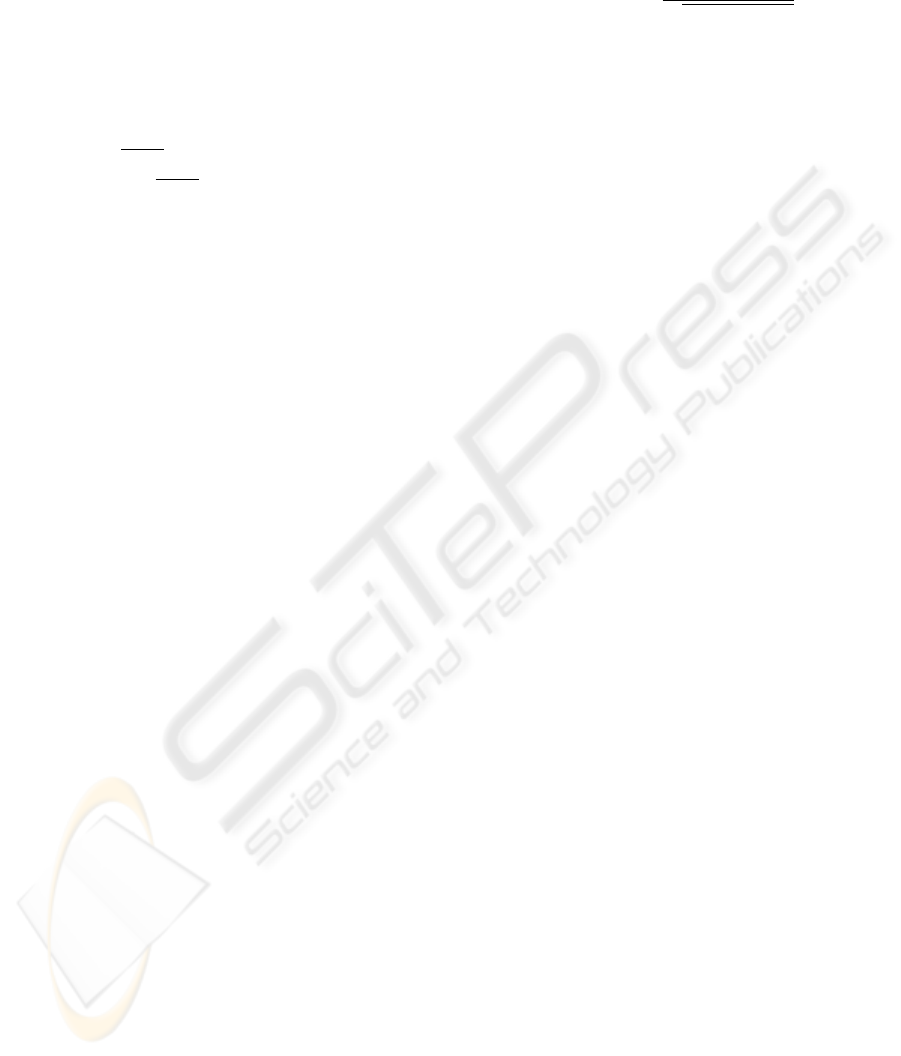
Ψ
ρ
j
,α
(k, p, q) =
ρ
j+1
X
ρ
j
α+θ
X
α
ρ
k
µ(F (ρ, θ))cos
p
θsin
q
θ
(10)
where k is order of the radial moments and (p + q) is
the order of the angular moments and
µ =
0 f ≤ a
2 × [
(f−a)
(c−a)
]
2
a ≤ f ≤ b
1 − 2 × [
(f−a)
(c−a)
]
2
b ≤ f ≤ c
1 x ≥ c
(11)
4 QUERY PROCESSING
The color image retrieval must be researched in the
certain color space. The YUV space is selected to
research the color image retrieval therefore we must
perform all the operations according to the Y,U,V
components.
The general algorithm for color image retrieval is as
follows:
1. Calculate the three component images of the color
image in the certain color space (i.e. YUV)
2. Calculate the color moments for each component
image
3. Calculate the Zernike moments for each compo-
nent image
4. Calculate the fuzzy moments for each component
image
5. Each component image corresponds to feature vec-
tors V
c
= [CM F V
c
, SM F V
c
, F M F V
c
] where
CM F V
c
is color moment feature vector (3 mo-
ments for each image components), and SM F V
and F M F V are respectively Zernike moments and
fuzzy moments for each image components c =
Y, U, V .
6. Feature vector for color image is
V
imag e
= [V
Y
, V
U
, V
V
]
7. Calculate the distance between each subfeature of
one image to the other images and the minimum is
selected as the feature distance between color im-
ages
8. Take the feature distance as the image similarity to
perform the color image retrieval.
Three similarity functions sim
Y
(Q, D),
sim
U
(Q, D) and sim
V
(Q, D), respectively account-
ing for YUV image components, are computed. Each
function simX(Q, D) between a database image
feature, defined by the tuple D = (d
0
, d
1
, . . . , d
n
),
and the query image feature, also defined by a tuple
Q = (q
0
, q
1
, . . . , q
n
) is computed using the cosine
similarity coefficient, defined as:
sim(Q, D) =
P
d
i
q
i
p
P
d
2
i
×
P
g
2
i
(12)
The resulting coefficients are merged to form the final
similarity function as:
sim(Q, D) = a × sim
Y
(Q, D) +
+b × sim
U
(Q, D) + c × sim
V
(Q, D) (13)
where a, b and c are weighting coefficient empirically
set.
5 CONCLUSION
Currently available large images repositories require
new and efficient methodologies for query and re-
trieval. Content based access appears to be a promis-
ing direction to increase the efficiency and accuracy
of unstructured data retrieval. We have introduced a
system for similarity evaluation based on the extrac-
tion of simple features such as color and fuzzy image
moments. We considered these features as a simple
set perceptually useful in the retrieval from thematic
databases, i.e. limited to a common semantic domain.
REFERENCES
A. Khotanzad (1990). Invariant image recognition by
zernike moments. IEEE Trans. on Patt.Anal.and
Mach. Intell., 12(5):489–497.
G. Taubin, D.B. Cooper (1991). Recognition and position-
ing of rigid objects using algebraic moment invariants.
In SPIE Conf. On Geometric Methods in Computer Vi-
sion, volume 1570, pages 175–186.
M.J. Swain, D.H. Ballard (1991). Color indexing. Interna-
tional Journal of Computer Vision, 7(1):11–32.
M.S. Lew (2001). Principles of Visual Information Re-
trieval. Springer-Varlag, London.
M. Stricker, M. Orengo (1995). Similarity of color images.
In SPIE Storage and Retrieval for Image and Video
Databases III, volume 2185, pages 381–392.
T. Gevers, A.W.M. Smeulders (1999). Colour based object
recognition. Pattern Recognition, 32:453–464.
W. Niblack et all. (1993). Querying images by content, us-
ing color, texture, and shape. SPIE Conference on
Storage and Retrieval for Image and Video Database,
1908:173–187.
ICINCO 2005 - ROBOTICS AND AUTOMATION
398