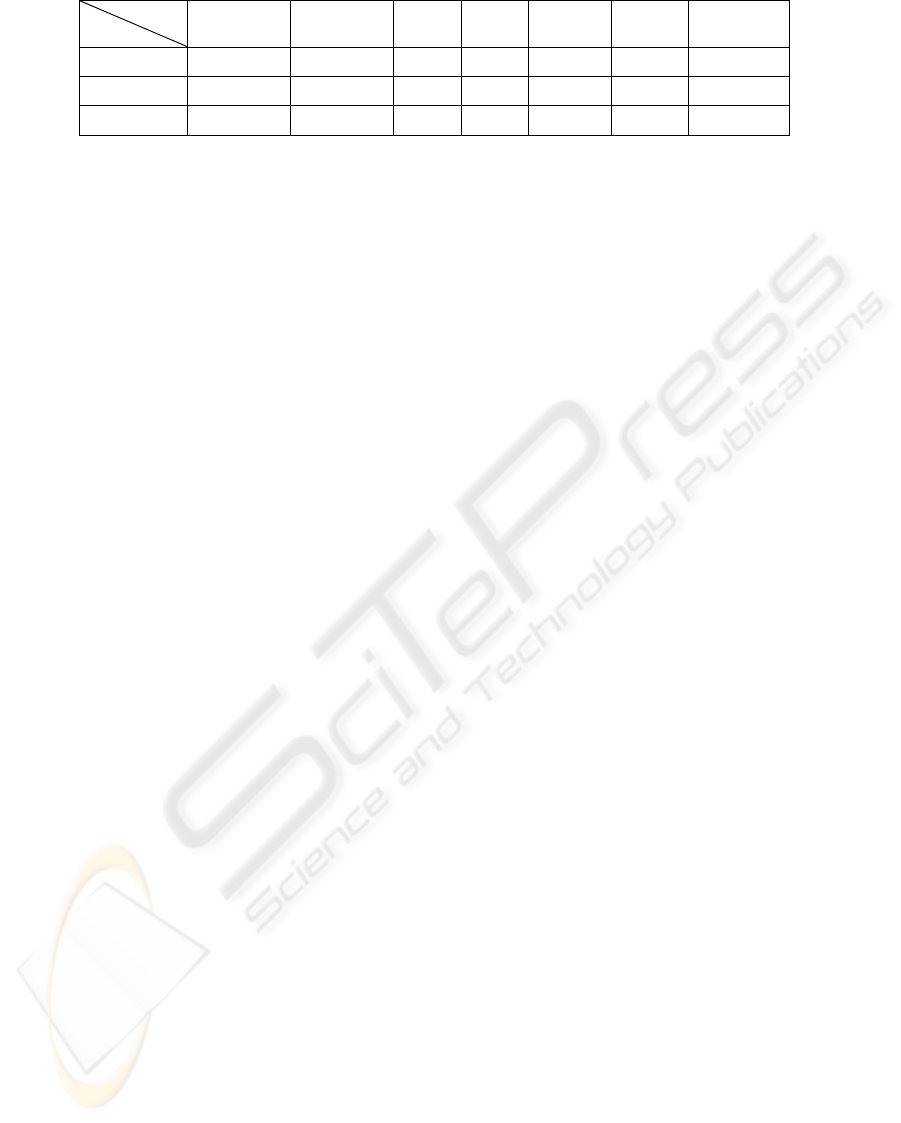
Table 4: Residual error of different pairs of medical images
Method
Group
RANSAC MLESAC MGA SEA SEA+T FMEA FMEA+T
MG1 6.537 1.664 1.584 1.702 1.887 1.637 1.621
MG2 8.025 2.124 1.621 2.116 2.075 1.936 1.907
MG3 29.06 3.032 2.135 2.626 2.651 2.452 2.276
5 CONCLUSION
In this paper, we described a novel competitive
evolutionary agent-based approach to trifocal tensor
estimation, which employs a new competitive
strategy to control the breeding number of new
agents and reduce the chance of reproducing unfit
ones. It focuses on the reproduction behavior to
reduce the computation time, and produces results
commensurate with, or superior to, that of SEA. The
experimental results indicate that the proposed
method attains a high level of performance in terms
of accuracy and computational efficiency. It can
obtain an optimal (or near optimal) result in the
solution space and is robust to outliers, even when a
large number of outliers are involved.
By accurately estimating the trifocal tensor, it
will now be possible to generate 3D views of the
sequence of 2D images. This brings the authors
closer to their ultimate goal, the real time generation
of 3D views during a laparoscopic procedure in
order to enhance features, in particular obscured
features, to the surgeon. This requires that the
geometric data are estimated as fast and accurately
as possible. The novel finite multiple evolutionary
agent-based approach presented here allows us to do
this.
REFERENCES
Faugeras O. and Papadopoulo T., 1998. “A nonlinear
method for estimating the projective geometry of 3
views”, in Proc. 6
th
International Conference on
Computer Vision, pp.477-484.
Hartley R. and Zisserman A., 2000. “Multiple view
geometry in computer vision,” Cambridge University
Press.
Hartley R. and Vidal R., 2004. “The multibody trifocal
tensor: motion segmentation from 3 perspective
views”, in Proc. Computer Vision and Pattern
Recognition, vol. 1, pp.769-775.
Hu M.X. and Yuan B.Z., 2002. “Robust Estimation of
Trifocal Tensor using Messy Genetic Algorithm”, in
Proc. 16
th
International Conference on Pattern
Recognition, vol. 4, pp.347-350.
Hu M.X. and Yuan B.Z., 2002. “Novel estimation of
trifocal tensor using GMM,” IEE Electronics Letters,
vol. 38, no. 19, pp.1094-1095
.
Hu M.X., Dodds G. and Yuan B.Z., 2004. “Evolutionary
agents for epipolar geometry estimation”, in Proc.
IEEE International Conference on Image Processing,
2004, vol. 2, 1843-1846.
Liu J.M, Tang Y.Y. and Cao Y.C., 1997. “An evolutionary
autonomous agents approach to image feature
extraction”, IEEE Trans. On Evolutionary
Computation, vol. 1, no. 2, pp. 141-158.
Matei B., Georgescu B., Meer P., 2001. “A versatile
method for trifocal tensor estimation,” in Proc. 8
th
International Conference on Computer Vision, vol. 2,
pp.578-585.
Quan L., 1994. “Invariants of 6 points from 3 uncalibrated
images”, in Proc. European Conference on Computer
Vision, vol. 2, pp.459-470.
Shashua A., 1995. “Algebraic functions for recognition,”
IEEE Transactions on Pattern Analysis and Machine
Intelligence, vol. 17, no. 8, pp. 779-789.
Torr P. H. S., 1995. “Outlier detection and motion
segmentation,” PhD thesis, University of Oxford
.
Torr P. H. S. and Zisserman A., 1997. “Robust
parameterization and computation of the trifocal
tensor,” Image and Vision Computing, vol. 15, pp.
591-605.
Veenman C.J., Reinders M.J.T. and Backer E., 2003. “a
cellular coevolutionary algorithm for image
segmentation”, IEEE Trans. Image Processing, vol.
12, no. 3, pp. 304-316.
MULTIPLE VIEW GEOMETRY ESTIMATION BASED ON FINITE-MULTIPLE EVOLUTIONARY AGENTS FOR
MEDICAL IMAGES
209