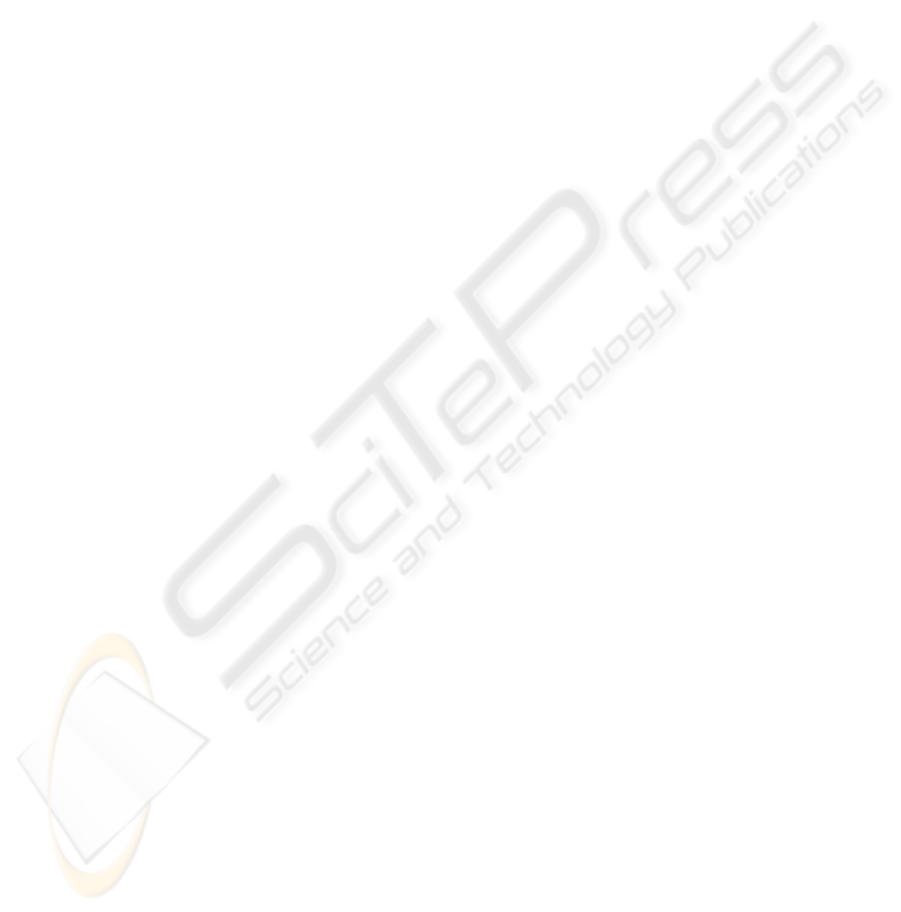
can be interpreted as the number of occurrences of
the data vector Ψ. Choosing the prior pdf f(Θ) in
the Dirichlet form ∝
Q
Ψ∈Ψ
∗
Θ
¯
V
y|ψ
−1
y|ψ
, the externally
supplied information increases it to the initial value
V
0
=
¯
V + νM . The posterior pdf is also Dirichlet
one given by the occurrence table V
t
. It evolves start-
ing from the initial value V
0
. The updating by the
observed data V
t
= V
t−1
+ B(Ψ
t
) adds the number
of occurrences of the values Ψ
τ
= Ψ, τ ≤ t, to the
Ψth entry of the table V
0
.
Again, importance of the prior knowledge can be
hardly over-stressed: the estimation of controlled
Markov chains is formally extremely simple but the
dimension of the occurrence table V grows exponen-
tially with the cardinality of the set Ψ
∗
. Consequently,
there is a lack of data in majority practical cases and,
moreover, their information content is as a rule insuf-
ficient.
6 CONCLUSIONS
The simple presented result is a quite powerful and
practical tool. Considering the parameterized model
m(Θ, Ψ) from the exponential family and a conju-
gate prior pdf, the posterior pdf f (Θ|M, ν) remains
in the conjugate form as it is in “proper” Bayesian es-
timation. Evaluation of
R
M(Ψ) ln(m(Ψ, Θ)) dΨ of-
ten reduces into evaluation of moments of Ψ. More-
over, a simulation model of a quite different nature
than the estimated one can be used for estimating
R
M(Ψ) ln(m(Ψ, Θ)) dΨ. In this case, the use of
M(Ψ) is often reduced into evaluation of sample mo-
ments of Ψ.
More complex models – probabilistic mixtures –
can be estimated by the proposed method using, e.g.,
a slightly modified quasi-Bayes algorithm (K
´
arn
´
y
et al., 2005).
Physically motivated models, black box models of
much higher order than the estimated one, relation-
ships described by production rules stimulated by real
data in past etc. may serve as data-vectors sources.
In this way, model simplification and translation be-
tween various knowledge domains are addressed in a
justified, purposeful and simple way.
The choice of the weight ν is an algorithmically
open problem. Its solution is, however, predictable:
Bayesian hypothesis testing and real data observed by
the participant should provide flexible universal solu-
tion.
Similarly, the case when information about some
entries of Ψ
t
is only offered is unsolved. It is expected
that assignment the extension of M to the set Ψ
∗
by a
very flat marginal on “non-reported” entries of Ψ will
solve this problem.
Even with this open problems pending, the pro-
posed “technology” is straightforward to pass un-
certain knowledge from one participant to another
one and thus to combine very different knowledge
sources.
ACKNOWLEDGEMENTS
This research has been partially supported by
GA
ˇ
CR grant 102/03/0049, AV
ˇ
CR project BADDYR
1ET100750401, M
ˇ
SMT grant 1M6798555601 DAR,
and AV
ˇ
CR project 1ET100750404.
REFERENCES
Barndorff-Nielsen, O. (1978). Information and exponential
families in statistical theory. Wiley, New York.
Berger, J. (1985). Statistical Decision Theory and Bayesian
Analysis. Springer-Verlag, New York.
Jirou
ˇ
sek, R. (2003). On experimental system for multidi-
mensional model development MUDIN. Neural Net-
work World, (5):513–520.
K
´
arn
´
y, M., B
¨
ohm, J., Guy, T., Jirsa, L., Nagy, I., Nedoma,
P., and Tesa
ˇ
r, L. (2005). Optimized Bayesian Dynamic
Advising: Theory and Algorithms. Springer, London.
to appear.
K
´
arn
´
y, M., Khailova, N., Nedoma, P., and B
¨
ohm, J.
(2001). Quantification of prior information revised.
International Journal of Adaptive Control and Signal
Processing, 15(1):65–84.
Meneguzzo, D. and Vecchiato, W. (2004). Copula sensitiv-
ity in collateralized debt obligations and basket default
swaps. Journal of Futures Markets, 24(1):37–70.
Peterka, V. (1981). Bayesian system identification. In
Eykhoff, P., editor, Trends and Progress in System
Identification, pages 239–304. Pergamon Press, Ox-
ford.
Savage, L. (1954). Foundations of Statistics. Wiley, New
York.
ICINCO 2005 - SIGNAL PROCESSING, SYSTEMS MODELING AND CONTROL
232