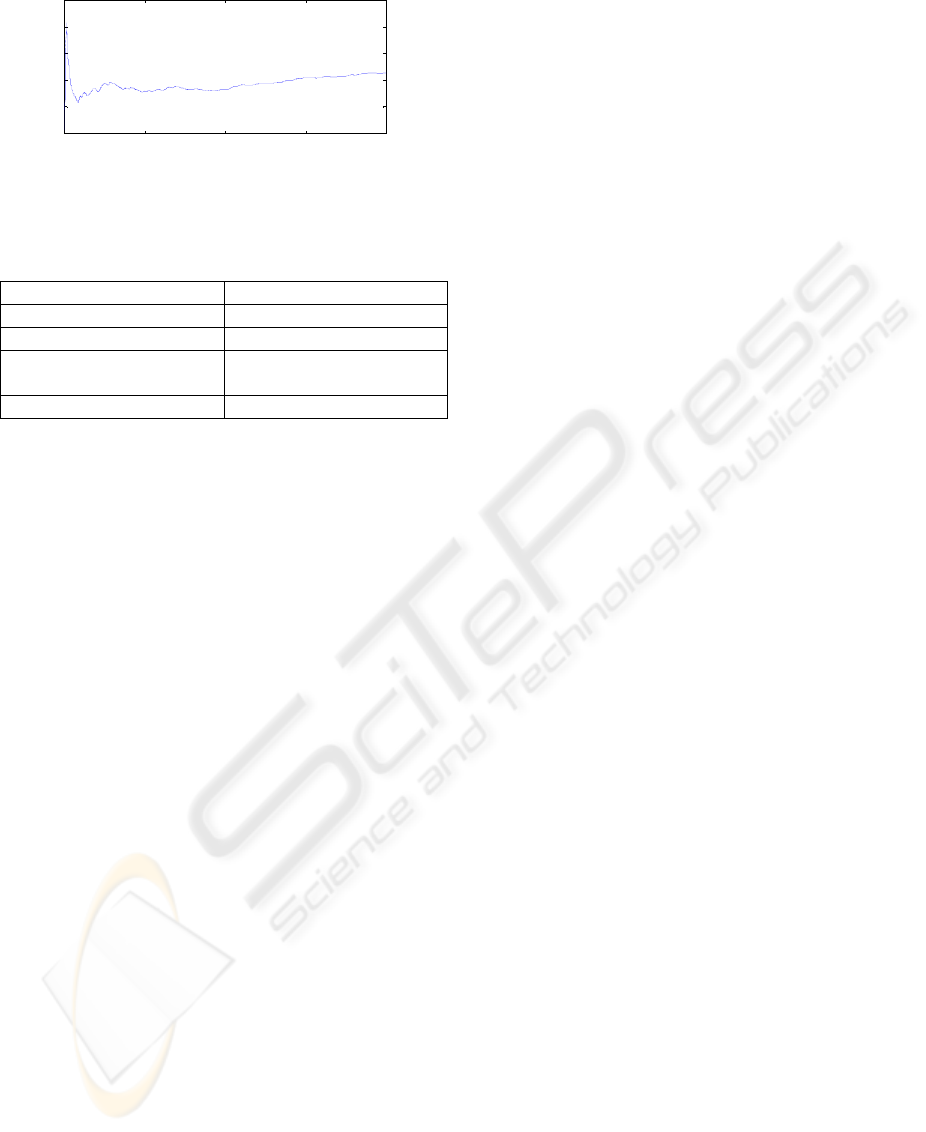
0 5 10 15 20
0
0.5
1
1.5
2
2.5
b) MSE of control.
Figure 8: Trajectory tracking control results obtained
using a fuzzy controller
Table 1: Mean Squared Error of identification and control
Name FNHMM vs. single RTNN
Systems identification: 0.08% vs. 0.27%
Feedforward control: 1.5% vs. 2.3%
Feedforward plus feedback
direct adaptive control:
0.41% vs. 2.7%
Fuzzy control: 5.8% (does not use NNs)
From Figures 6, 7, 8 and the MSE% data from Table
1, we could conclude that: the systems identification
using FNHMM gives better results than that using
only one RTNN; the control schemes which use
FNHMMC works better than that using one RTNN;
the FNHMM feedforward/feedback direct adaptive
control gives better results with respect to the
FNHMM feedforward control; the fuzzy control is
worse with respect to the neural control, especially
when the friction parameters changed.
6 CONCLUSIONS
A FNHMM for identification and control of
complex nonlinear plants is proposed. Two control
schemes of FNHMM has been experimented and
compared with a respective single-RTNN and fuzzy
control. The comparison of identification results for
a 1 DOF mechanical system with friction show that
the FNHMM identifier has a better performance
with respect to the identification using one RTNN.
The same is valid for the schemes of control. The
better control is the feedforward/feedback control
and the worse control is the fuzzy control.
REFERENCES
Babushka, R., and H. B. Verbruggen, 1997. Fuzzy
modeling: principles, methods and applications. In
Proc. of the Int. Workshop on Intelligent Control
INCON'97, Sofia, Bulgaria, Oct. 13-15, Bulgarian
Union for Automation and Informatics, pp. 1-23.
Baruch I., and E. Gortcheva, 1998. Fuzzy neural model for
nonlinear systems identification. In: Proc. of the
AARTC IFAC Workshop, Cancun, Mexico, April 15-
17, p.p. 283-288.
Baruch I., Flores J.M., Thomas F., and R. Garrido, 2001a.
Adaptive neural control of nonlinear systems. In Proc.
of the Int. Conf on NNs, ICANN 2001, Lecture Notes
in Computer Science, vol. 2130, Springer-Verlag,
Berlin, Heidelberg, N. Y., p.p. 930-936.
Baruch, I., Flores, J.M., and R. Garrido, 2001b. A fuzzy-
neural recurrent multi-model for systems identification
and control. In Proc. of the Europ. Contr. Conference,
ECC’01, Porto, Portugal, Sept. 4-7, p.p. 3540-3545.
Baruch I., Flores J.M., Nava F., Ramirez I.R., and B.
Nenkova, 2002. An advanced neural network topology
and learning, applied for identification and control of a
D.C. motor. In Proc. of the 1-st Int. IEEE Symp. on
Intelligent Syst., Varna, Bulgaria, Sept., pp. 289-295.
Eikens, B., and M.N. Karim, 1999. Process identification
with multiple neural network models. Internat.
Journal of Control, vol. 72, No 7/8, pp. 10-20.
Frasconi, P., Gori, M., and G. Soda, 1992. Local feedback
multilayered networks. Neural Computation, vol. 4,
pp. 120-130.
Gupta, M., Nikiforuk, P., and L. Jin, 1994. Adaptive
control of discrete time nonlinear systems using
recurrent neural networks. IEE Proc. Control Theory
and Applications, vol. 141, No 3, pp. 169-176.
Hunt, K.J., Sbarbaro, D., Zbikowski, R., and P. J.
Gawthrop, 1992. Neural network for control systems
(A survey). Automatica, vol. 28, pp. 1083-1112.
Hunt, K.J., Irwin, G.R., and K. Warwick, 1995. Neural
network engineering in dynamic control systems.
Springer Verlag, London.
Jin, L., and M. Gupta, 1999. Stable Dynamic
Backpropagation Learning in Recurrent Neural
Networks. IEEE Transactions on Neural Networks,
vol. 10, pp. 1321-1334.
Lee, S.W., and J.H. Kim, 1995. Robust adaptive stick-slip
friction compensation. IEEE Trans. on Ind. Elect., vol.
42 , No. 5, p.p. 474-479.
Mastorocostas P.A., and J.B. Theocharis, 2002.A recurrent
fuzzy-neural model for dynamic system identification.
IEEE Transactions on Systems, Man and Cybernetics,
Part B: Cybernetics, vol. 32, pp. 176-190.
Miller III, W.T., Sutton, R.S., and P.J. Werbos, 1992.
Neural networks for control. MIT Press, London.
Narendra K. S., and K.Parthasarathy, 1990. Identification
and control of dynamical systems using neural
networks. IEEE Transactions on Neural Networks,
vol. 1, No. 1, pp. 4-27.
Omatu, S., Khalil, M., and R. Yusof, 1995. Neuro-control
and its applications. Springer Verlag, London.
Takagi, T., and M. Sugeno, 1985. Fuzzy identification of
systems and its applications to modeling and control.
IEEE Trans. Systems, Man, and Cybernetics, vol. 15,
pp. 116-132.
A HIERARCHICAL FUZZY-NEURAL MULTI-MODEL: An application for a mechanical system with friccion
identification and control
235