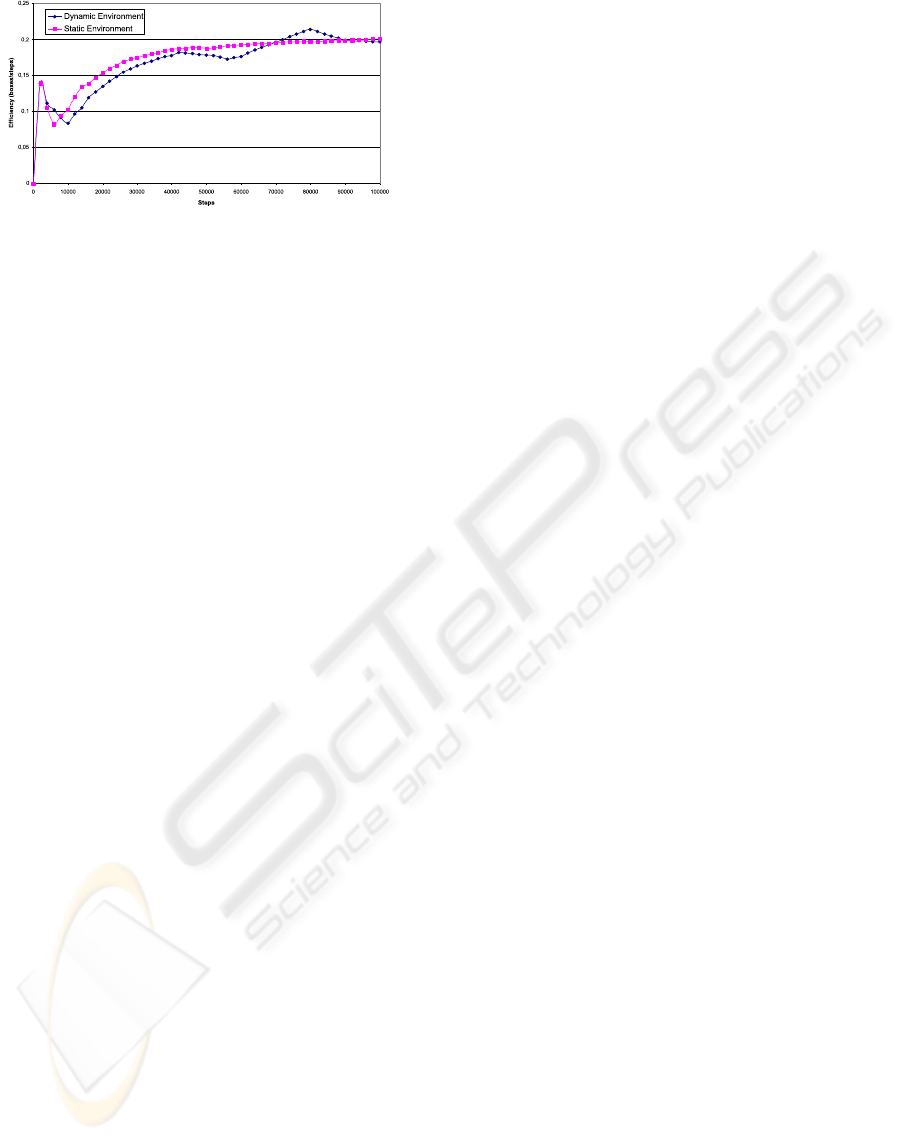
Figure 6: Compared efficiency (boxes/time) between a sim-
ulation in a static environment with two corridors and an-
other one in an dynamic environment with 4 corridors
which 2 corridors are randomly closed every 10,000 steps.
the effect of the environmental dynamic become neg-
ligible with a great number of steps. Figure 6 shows
the efficiency for two different environment (static or
dynamic). The efficiency of the system in the static
one is more stable but equivalent to the efficiency of
the system which evolves in the dynamic one.
Discussion. The AMAS cooperative agent ap-
proach is relevant for several reasons. Firstly, unlike
ant algorithms (Bonabeau et al., 1997), robots do not
physically mark their environment with pheromones,
but memorize personal markers. Secondly, contrary
to competition-based (Vaughan et al., 2000) or altruis-
tic (Lucidarme et al., 2002) approaches, robots do not
need direct communication to inform close robots or
exchange requests and intentions. Thirdly, coopera-
tive behavior encoding is insensitive to the number of
robots, to the topography and to the dimensions of the
environment. Fourthly, no global feedback is needed
to lead the system to functional adequacy, which pre-
vents the system to reach local extrema. Finally, ob-
tained collectives are robust and adaptive, even if per-
ception capabilities are very limited. Nevertheless,
ADELFE does not provide any guidance to instan-
tiate these values. For instance, the initial weights
for markers and the forgetting factor have been ad-
justed to the time robots spend to cross the entire
environment. This might be completely different in
a more complex environment with more or less cor-
ridors which can dynamically open or close. Some
simulations have been done with such environments,
and the affected values seem correct unless corridors
are too close to each other or frequency of closure is
too fast. These values also may be learnt at runtime,
which is one of our perspectives.
5 CONCLUSION
In this paper, we have shown the relevance of co-
operation as a local criterion for collectives to self-
organize to be more adapted to a specific task. Con-
sidering the ignorance of the global task and the
environment, the self-organizing collective reaches
an emergent coherent behavior, which is then more
robust to environmental risks. Our simulation ap-
plication tackles a simple problem in a simple sta-
tic environment in which the collective achieves its
global task. Simulations in difficult and dynamic en-
vironments confirm the relevance of cooperative self-
organizing collectives. Finally, this application has
been developed to confront the ADELFE cooperative
agent model to a task that requires adaptation and ro-
bustness. However, this application is very specific,
in the sense it only concerns quasi-reactive non com-
municative robots. In the case of agents able of com-
municative acts, other kinds of NCS are possible.
REFERENCES
Bernon, C., Camps, V., Gleizes, M.-P., and Picard, G.
(2003). Designing Agents’ Behaviors and Interac-
tions within the Framework of ADELFE Method-
ology. In 4
th
Engineering Societies in the Agents
World (ESAW’03), volume LNCS 3071, pages 311–
327. Springer-Verlag.
Bonabeau, E., Theraulaz, G., Deneubourg, J.-L., Aron, S.,
and Camazine, S. (1997). Self-organization in social
insects. Trends in Ecology and Evolution, 12:188–
193.
Capera, D., Georgé, J., Gleizes, M.-P., and Glize, P. (2003).
The AMAS theory for complex problem solving based
on self-organizing cooperative agents. In 1
st
Int.
TAPOCS Workshop at 12
th
IEEE WETICE, pages
383–388. IEEE.
Heylighen, F. (1999). The Science of Self-organization and
Adaptivity. EOLSS Publishers.
Lucidarme, P., Simonin, O., and Liégeois, A. (2002). Imple-
mentation and Evaluation of a Satisfaction/Altruism
Based Architecture for Multi-Robot Systems. In IEEE
Int. Conf. on Robotics and Automation (ICRA’02),
pages 1007–1012.
Maturana, H. and Varela, F. (1994). The Tree of Knowledge.
Addison-Wesley.
Picard, G. and Gleizes, M.-P. (2002). An Agent Architec-
ture to Design Self-Organizing Collectives: Principles
and Application. In AISB’02 Symposium on Adaptive
Multi-Agent Systems (AAMASII), volume LNAI 2636,
pages 141–158. Springer-Verlag.
Topin, X., Fourcassié, V., Gleizes, M.-P., Theraulaz, G.,
Régis, C., and Glize, P. (1999). Theories and experi-
ments on emergent behaviour : From natural to artifi-
cial systems and back. In European Conf. on Cogni-
tive Science, Siena, Italy.
Vaughan, R., Støy, K., Sukhatme, G., and Matari
´
c, M.
(2000). Go ahead make my day: Robot conflict reso-
lution by aggressive competition. In 6
th
Int. Conf. on
Simulation of Adaptive Behaviour.
COOPERATIVE SELF-ORGANIZATION TO DESIGN ROBUST AND ADAPTIVE COLLECTIVES
241