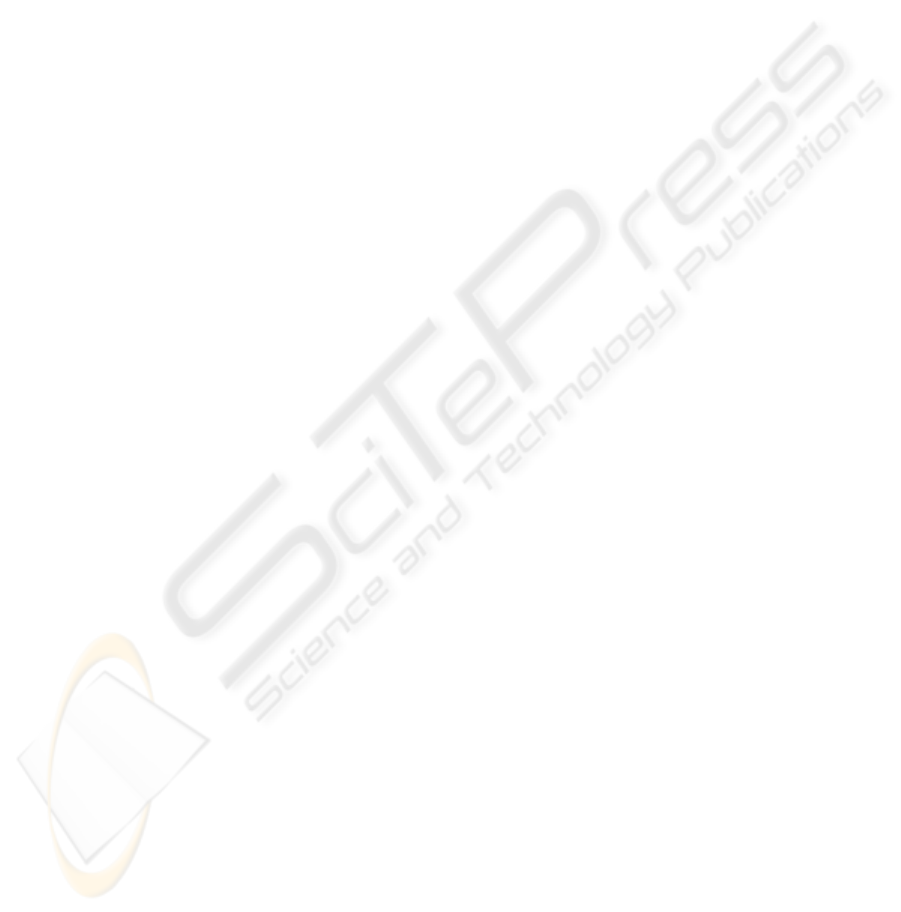
nm in real world coordinates. The accuracy of the
orientation estimation is 1°. The computation speed
depends strongly on the pattern size and the number
of cross-correlations per frame. In the presented case
the average number of cross-correlations per frame
is between 3 and 4. With a standard PC (P4, 2.6
GHz), the computation time is 0.02 s for one pose
estimation without orientation calculation and
approximately 0.07 s with orientation. With a SEM
frame rate of 10 fps real-time capability has been
demonstrated. Only for the first frame 0.8 s are
needed to determine the start ROI.
The acquisition time for one pixel is 250 ns.
Therefore the minimum image acquisition time for a
128x128 ROI is about 4.1 ms. The user can chose
between a high frame rate with low image quality
and a slower frame rate with better image quality. In
general, one should adopt the frame rate to the
processing time of the pose estimation algorithm.
Therefore an adaptive scan speed has been
implemented.
The disadvantage of the cross correlation
approach is that changes of the targets shape are
hard to recognize. For every magnification of the
SEM, the pattern has to be adapted, because the
scale of the target changes with the magnification. If
only few magnification steps are used this problem
can overcome by using additional patterns. A more
serious problem is shape variation by deformation,
e.g. while a gripping process.
6 CONCLUSION
The real-time pose estimation of micro- and
nanoobjects inside a SEM image stream requires
image processing algorithms with high robustness
against noise. Cross correlation is a powerful
method to overcome this problem. The disadvantage
that computation time is very high can be
compensated by using ROI’s. Here an advantage of
the image acquisition process of SEM’s can be used.
Because only a small region will be scanned, one
can choose between high image acquisition speed
and high image quality in comparison with a full
size scan. Overall this leads to real-time capability
for the sensor system. The presented approach
enables automatic positioning of mobile microrobots
with nanometer resolution, which is a further step
towards automatic nanohandling.
ACKNOWLEDGMENT
This paper is based on research work supported by
the European Union; project ROBOSEM (GRD1-
2001-41864).
REFERENCES
Yang, G., Gaines, J. A., Nelson, B.J., 2003. A supervisory
wafer-level 3D microassembly system for hybrid
MEMS fabrication, Journal of Intelligent and Robotic
Systems, nr. 37, Kluwer Academic Publishers, pp. 43-
68
Nakajima, M., Arai, F., Dong, L., Nagai, M., Fukuda, T.,
2004. Hybrid Nanorobotic Manipulation System
inside Scanning Electron Microscope and
Transmission Electron Microscope, Proc. of IEEE/RSJ
Int. Conference of Intelligent Robots and Systems, pp.
589-594
Garnica, S., Hülsen, H., and Fatikow, S., 2003.
Development of a Control System for a Microrobot-
Based Nanohandling Station, Proc. of 7th Int. IFAC
Symp. on Robot Control (SYROCO), Wroclaw,
Poland, pp. 631-636
Kortschack, A., Fatikow, S., 2004. Development of a
mobile nanohandling robot, Journal of
Micromechatronics, Volume 2, Nr. 3, pp. 249 – 269
Hülsen, H., 2004. Design of a fuzzy-logic-based
bidirectional mapping for kohonen networks. In Proc.
Int. Symposium on Intelligent Control (ISIC’04),
Taipei, Taiwan.
Fatikow, S. et al., 2004. Versatile Nanohandling Robot
Cell in a Scanning Electron Microscope, 12th
Mediterranean Conference on Control and
automation.
www.pointelectronic.de
Goudail, F., Réfrégier, P., 2003 Statistical Image
Processing Techniques for Noisy Images – An
Application-Oriented Approach, Kluver Academic /
Plenum Publisher.
Weisstein, E.W. "Cross-Correlation Theorem." From
MathWorld--A Wolfram Web Resource.
http://mathworld.wolfram.com/Cross-
CorrelationTheorem.html
Mølhave, K., Hansen, T. M., Madsen, D. M. and Bøggild,
P., 2004. Towards Pick-And-Place Manipulation of
Nanostructures, Journal of Nanoscience and
Nanotechnology, 4, 279-282
ICINCO 2005 - ROBOTICS AND AUTOMATION
198