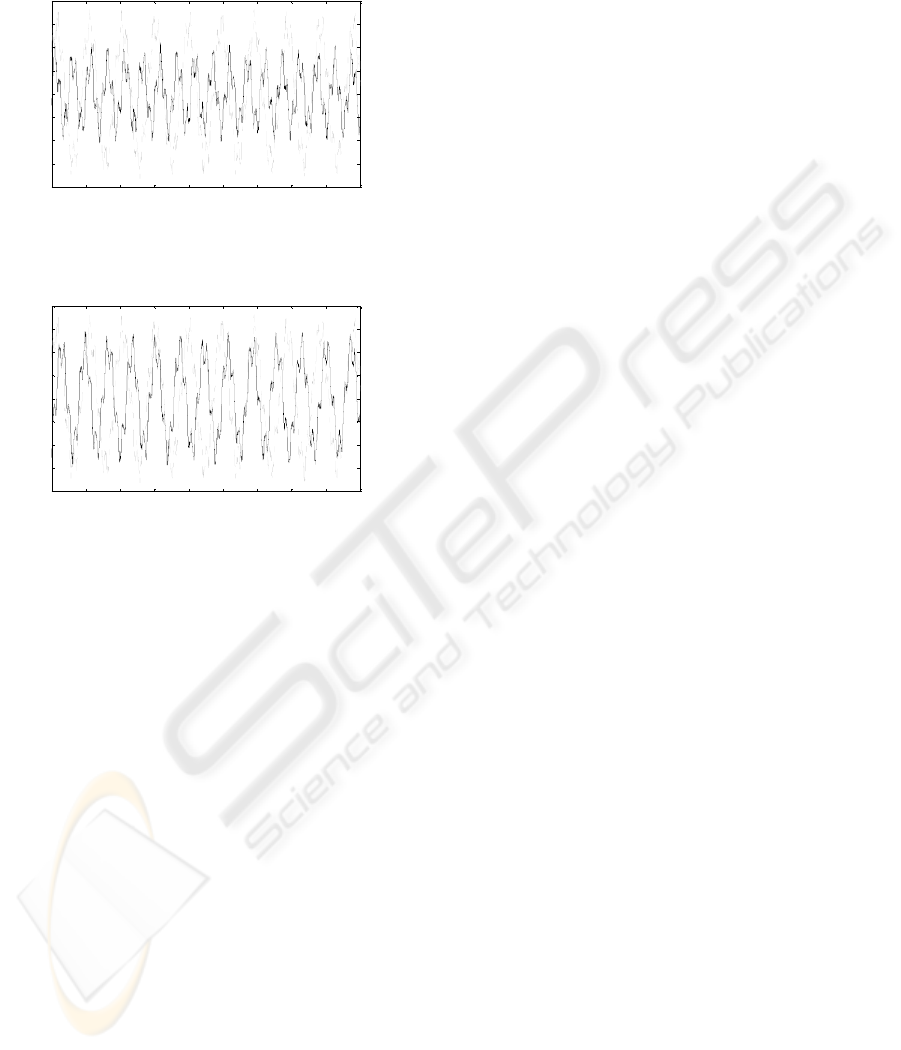
fluctuation after cancellation using ANFIS is 4, GA
is 1.8 and RLS is 1.2 times smaller as compared to
the fluctuation before cancellation.
0 0.5 1 1.5 2 2.5 3 3.5 4 4.5
-8
-6
-4
-2
0
2
4
6
8
x 10
-4
Tim e (s e c )
Defl ection (m)
Figure 5: Performance in implementing the AVC
algorithm using GA
0 0.5 1 1.5 2 2.5 3 3.5 4 4.5
-8
-6
-4
-2
0
2
4
6
8
x 10
-4
Tim e (s e c )
Defl ecti on (m)
Figure 6: Performance in implementing the AVC
algorithm using RLS
4 CONCLUDING REMARKS
This paper has presented the relative real-time
performance and error convergence issues in
implementing system identification and AVC system
of a flexible beam vibration using, ANFIS, GA and
RLS algorithm. A comparative performance of the
algorithms has been presented and discussed through
a set of experiments. For system identification, it is
noted that the execution time in implementing
ANFIS as compared to GA and RLS is significantly
higher. However, ANFIS shows slightly better error
convergence for the same number of iterations. On
the other hand, real-time computing performance of
GA varies based on the selection of the size of
population and binary representation. It is noted that
the GA with higher bit representation and larger
population size for the same error convergence
performs slower than ANFIS. It is also noted that the
execution time for each of the three algorithms is
less than the sampling time, in turn satisfying the
real-time requirement. However, in case of GA, this
is true only for population size 10 with 8 bit
representation.
REFERENCES
Albertos, P., Crespo, A. and Simo, J. (2001). Real-time
constraints in intelligent control,
http://www.delet.ufrgs.br/vsbai/vsbai/artigos/1156.pdf
Amato P., Farina, M., Palma, G. and Porto, D. (2001). An
alife-Inspired evolutionary algorithm for adaptive
control of time-varying system, Proc EUROGEN
2001, Athens, Greece, pp. 15-16
Baxter, M. J., Tokhi, M. O. and Fleming, P. J. (1994).
Parallelising algorithms to exploit heterogeneous
architectures for real-time control systems,
Proceedings of IEE Control-94 Conference, Coventry,
21-24 March 1994, 2, pp. 1266-1271.
Hossain, M. A. (1995). Digital signal processing and
parallel processing for real-time adaptive noise and
vibration control, Ph.D. thesis, Department of
Automatic Control and System Engineering, The
University of Sheffield, UK.
Hossain, M. A. and Tokhi, M. O. (1997). Evolutionary
adaptive active vibration control, Proc Inst.
Mechanical Eng., 211(1), pp. 183-193.
Jang, J. S. R. (1993). ANFIS: Adaptive-Network-based
Fuzzy Inference Systems, IEEE Transactions on
Systems, Man, and Cybernetics, 23(3), pp. 665-685,
1993.
Jones, D. I. (1989). Parallel architectures for real-time
control, Electronics and Communications Engineering
Journal, 1(5), 217-224.
Kourmoulis, P. K. (1990). "Parallel processing in the
simulation and control of flexible beam structure
systems", PhD thesis, Dept. of Automatic Control &
Systems Engineering, The University of Sheffield.
Madkour, A. A. M., Hossain, M. A., Dahal, K. P. and Yu,
H., (2004). Real-time System Identification using
Intelligent Algorithms, Proceedings of IEEE SMC
UK-RI Chapter Conference 2004 on Intelligent
Cybernetic Systems, pp. 236-241.
Tokhi, M. O. and Hossain, M. A. (1994). Self-tuning
active vibration control in flexible beam structures,
Proc. IMECE-I: J. Systems Control Eng. 208(14), pp.
263-277.
Tokhi, M. O., Hossain, M. A. and Shaheed, M. H. (2002).
Parallel Computing for Real-time Signal Processing
and Control, Springer, London.
Yamlidou, K. Moody, J., Lemmon, M. and Antsaklis, P.
(1996). Feedback control of Petri nets based on place
invariants, Automatica, 32(1), pp. 15-28.
COMPARATIVE PERFORMANCE OF INTELLIGENT IDENTIFICATION AND CONTROL ALGORITHMS FOR A
FLEXIBLE BEAM VIBRATION
367