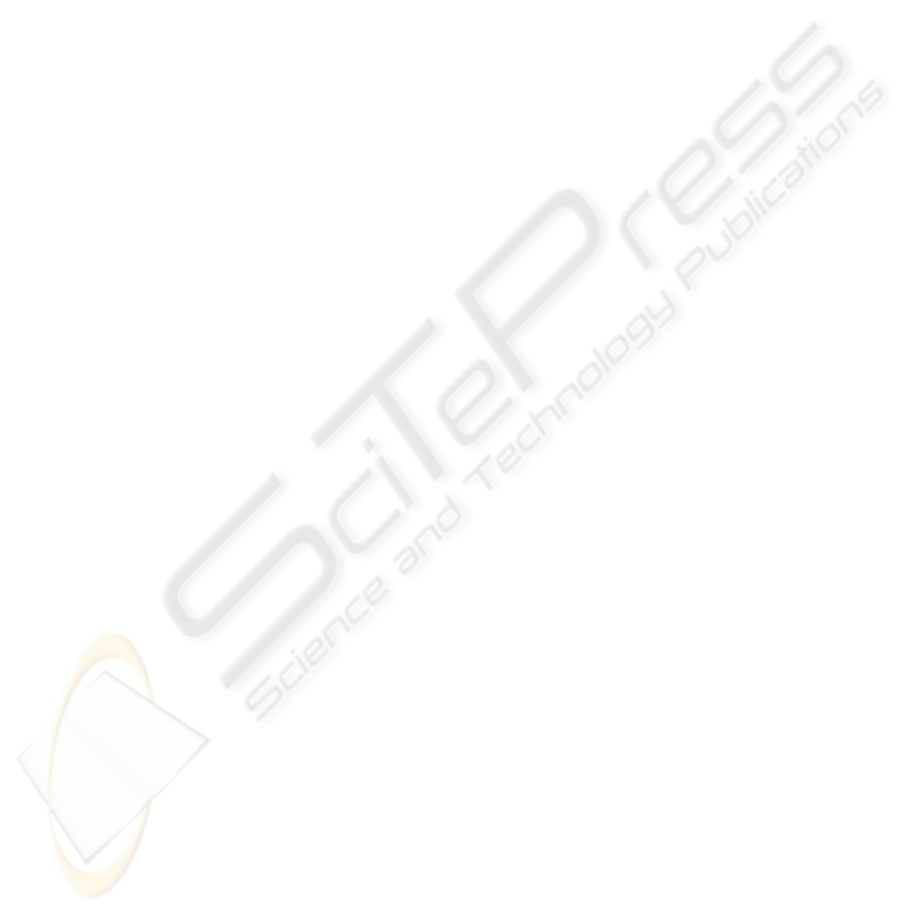
5 CONCLUSION AND
PERSPECTIVES
We have developed a newer approach called D
3
G
2
A.
This approach is a dynamic distributed double
guided genetic algorithm enhanced by three new
parameters called guidance probability P
guid
, the
local optima detector LOD and the weight ε,. The
latter is a weight used by Species agents to
determine their own genetic process parameters on
the basis of their chromosomes Fitness values.
Compared to the centralized guided genetic
algorithm and applied to LPCP, our new approaches
have been experimentally shown to be better in
terms
of fitness value and CPU time.
The improvement is due to both diversification
and guidance. The first increases the algorithm
convergence by escaping from local optima
attraction basin. The latter helps the algorithm to
attain optima. Consequently D
3
G
2
A gives more
chance to the optimization process to visit all the
search space. We have come to this conclusion
thanks to the proposed mutation sub-process. The
latter is sometimes random, aiming to diversify the
search process, and sometimes guided in order to
increase the best of the fitness fonction value. The
genetic sub-process of D
3
G
2
A Species agents will no
longer be the same depending on their fitness values.
This operation is based on the species typology. The
sub-population of a species agent can be considered
as strong or weak with reference to its fitness value.
For a strong species, it’s better to increase cross-over
probability and to decrease mutation probability.
However, when dealing with a weak species, cross-
over probability is decreased and mutation
probability is increased. The occurrence of these
measures not only diversifies the search but also
explore wholly its space.
No doubt further refinement of this approach
would allow its performance to be improved. Further
works could be focused on applying these
approaches to solve real hard CSOPs like the radio
link frequency allocation problem
REFERENCES
Bouamama S, Ghédira K., 2004. ED
3
G
2
A: an enhanced
Version of the dynamic Distributed double Guided
Genetic Algorithms for Max_CSPs. In SCI’048th
World Multiconference on Systemics, Cybernetics and
Informatics. IIIS press.
Bouamama S, Ghédira K.,2003. D
2
G
2
A: a Distributed
double Guided Genetic Algorithm for Max_CSPs. In
KES’03 the 7th International Conference on
Knowledge-Based Intelligent Information &
Engineering Systems, Oxford, UK.
Darwin C.1859, The Origin of Species, Sixth London
Editions, 1999.
DeJong K. A. , 1989. Spears W. M., Using Genetic
Algorithms to solve NP-Complete problems. George
Mason University, Fairfax, VA.
Freuder E.C, Wallace R.J,1992. Partial Constraint
Satisfaction, Artificial Intelligence, vol. 58, p 21-70,.
Ghédira K & Jlifi B, 2002. A Distributed Guided Genetic
Algorithm for Max_CSPs. Journal of sciences and
technologies of information (RSTI), journal of
artificial intelligence series (RIA), volume 16
N°3/2002
Goldberg D.E.,1989. Genetic algorithms in search,
Optimization, and Machine Learning, Reading, Mass,
Addison-Wesley, 1989.
Holland J., 1975. Adaptation in natural and artificial
systems, Ann Arbor: The University of Michigan
Press.
Lau, T. L. et Tsang, EPK., 1998. Solving the processor
configuration problem with the guided genetic
algorithm. In 10
ème
IEEE international conference on
Artificial Intelligence, Taiwan.
Michael B., Frank M., Yi P.,1999. Improved
Multiprocessor Task scheduling Using Genetic
Algorithms. In FLAIRS’ 99 twelfth International
Florida AI Research Society Conference, AAAI press,
p. 140-146.
Minton S. 1992. Minimizing conflicts a heuristic repair
method for constraint satisfaction and scheduling
problems. In Artificial Intelligence, volume 58 pages
161-205.
Schiex T., Fargier H. & Verfaillie G., 1995 Valued
constrained satisfaction problems: hard and easy
problems. In 14
th
IJCAI, Montreal, Canada august
1995.
Tsang EPK, 1993. Foundations of Constraints
Satisfaction. Academic Press Limited,
Tsang E.P.K, Wang C.J., Davenport A., Voudouris C.,
Lau T.L. 1999. A family of stochastic methods for
Constraint Satisfaction and Optimization. Technical
report University of Essex, Colchester, UK.
Tsujimura Y., Gen M., 1997. Genetic algorithms for
solving Multiprocessor Scheduling Problems. In the
1st Asia-Pacific Conference on Simulated Evolution
and Learning, LNAI, November 9-12, Springer, vol.
1285, p. 106-115.
D3G2A: A DYNAMIC DISTRIBUTED DOUBLE GUIDED GENETIC ALGORITHM FOR THE CASE OF THE
PROCESSORS CONFIGURATION PROBLEM
247