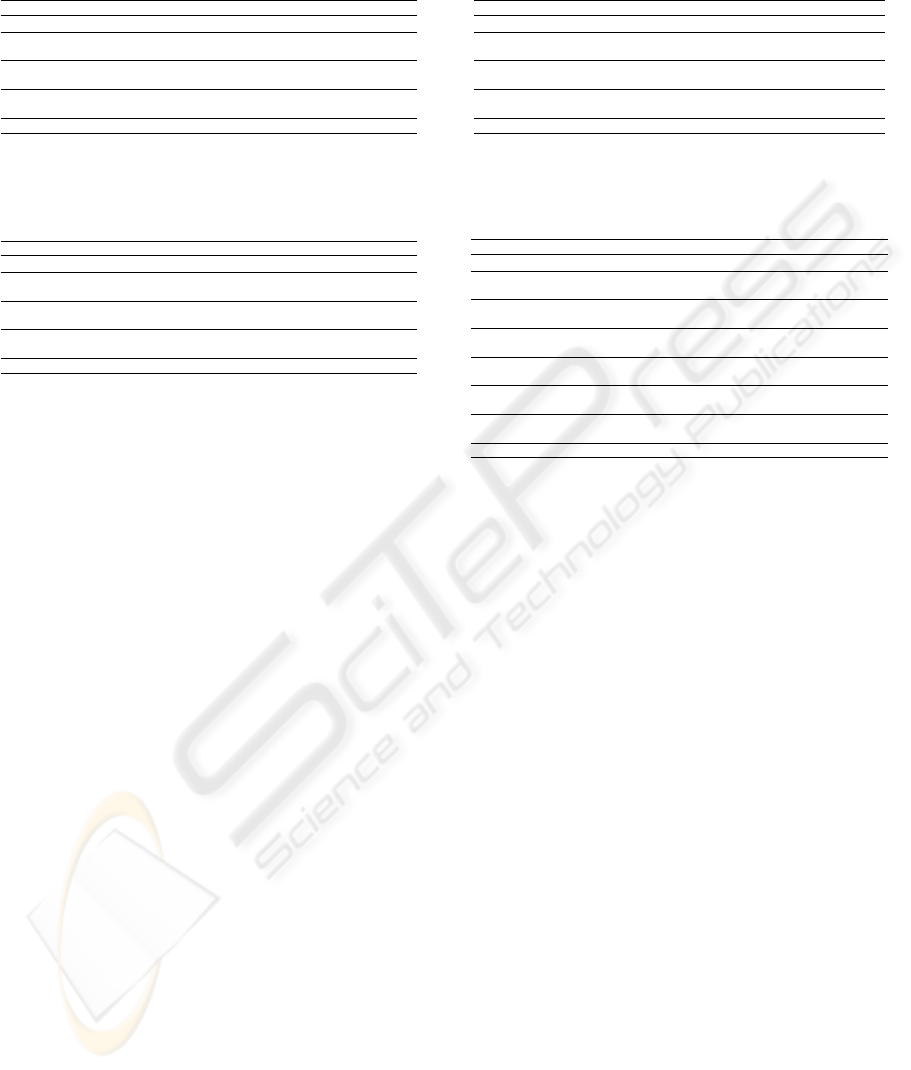
Table 1: System identification using signal model MA(2),
Mean, Standard deviation, NMSE, and ESR . SNR=20dB.
True Values LS GDA GNA
λ = 8. 10
−5
µ = 0.5
h(1) -2.3333 mean -2.1704 -2.2676 -2.2676
σ 0.0585 0.0149 0.0149
h(2) 0.6667 mean 0.5101 0.6541 0.6541
σ 0.0211 0.0060 0.0060
NMSE mean 0.0093 0.0008 0.0008
σ 0.0043 0.0003 0.0003
ESR -20.3041 -30.9500 -30.9500
Table 2: System identification using signal model MA(2),
Mean, Standard Deviation, NMSE, and ESR. SNR=10dB.
True Values LS GDA GNA
λ = 8. 10
−5
µ = 0.5
h(1) -2.3333 mean -2.1013 -2.1158 -2.2624
σ 0.1835 0.4664 0.0511
h(2) 0.6667 mean 0.4987 0.6166 0.6557
σ 0.0632 0.1275 0.0192
NMSE mean 0.0203 0.0478 0.0014
σ 0.0181 0.1490 0.0015
ESR -16.9250 -13.2057 -28.5387
The zeros of the system transfer function H(z) are lo-
cated at −2, 0.7±j0.7 and 0.25±j0.433. This model
has also been used in (Alshebeili, 1993), (Stogioglou,
1996), (Tugnait, 1990), and (Tugnait, 1991).
Additive colored noise is generated as the output of
the following MA(3) model (Abderrahim, 2001) and
(Na, 1995) :
v(k) = e(k)+0.5e(k−1)−0.25e(k−2)+0.5e(k−3)
where the input sequence e(k) is an i.i.d Gaussian se-
quence. In this case N = 10240. The simulation re-
sults are given in tables 4, 5, and 6.
In these simulations, we initialize the nonlinear op-
timization algorithms with the LS solution. The ad-
vantage of this is to avoid the convergence to local
minimum. The good value of the step-size allows also
to avoid this problem of the local minima.
Table 1 shows that the parameters estimation via
the proposed method is much more powerful than LS
solution in term of mean value, standard deviation, or
NMSE, and ESR . The Gradient Descent and Gauss-
Newton algorithms converge to the same values, but
the convergence speed is different. The figures 2 and
3 illustrate this.
Tables 2 and 5 show that the Gradient Descent al-
gorithm is sensitive to the additive noise. However,
the Gauss-Newton algorithm is more robust to mea-
surement noise.
We can note that for a low SNR (SNR = 0dB),
the estimate of the parameters is poor what is due
to the bad estimate of third and fourth order cumu-
lants. These estimation results could be improved by
processing more data, which allows to get better esti-
mates of the cumulants.
Table 3: System identification using signal model MA(2),
Mean, Standard Deviation, NMSE, and ESR. SNR=0dB.
True Values LS GDA GNA
λ = 8. 10
−5
µ = 0.5
h(1) -2.3333 mean -1.3781 -0.8631 -1.0952
σ 0.6033 0.6223 1.0662
h(2) 0.6667 mean 0.3217 0.3006 0.2882
σ 0.1794 0.2092 0.3499
NMSE mean 0.2418 0.4623 0.4964
σ 0.2464 0.2762 0.4890
ESR -6.1661 -3.3511 -3.0421
Table 4: System identification using signal model MA(5),
Mean, Standard Deviation, NMSE, and ESR. SNR=20dB.
True Values LS GDA GNA
λ = 6. 10
−9
µ = 0.5
h(1) 0.1 mean 0.0474 0.0779 0.0887
σ 0.0359 0.0384 0.0392
h(2) -1.87 mean -1.4212 -1.2822 -1.7798
σ 0.0845 0.0665 0.1010
h(3) 3.02 mean 2.2489 1.6874 2.8902
σ 0.1258 0.0667 0.1494
h(4) -1.435 mean -1.0779 -1.1768 -1.4206
σ 0.0671 0.0754 0.0876
h(5) 0.49 mean 0.3640 0.3927 0.4536
σ 0.0275 0.0301 0.0320
NMSE mean 0.0651 0.1484 0.0046
σ 0.0221 0.0184 0.0075
ESR -11.8660 -8.2867 -23.3527
By increasing the order q of the model, we use the
third and fourth order cumulants with large lags that
are poorly estimated for a low SNR. The consequence
of this is the bad parameters estimated (table 6) much
poorer than in the case of a model of order 2 (table 3).
These two algorithms are numerically expensive
compared to the Least-Squares algorithm.
5 CONCLUSION
In this paper, a blind identification of the MA mo-
dels using Higher-Order Statistics (HOS) is exposed.
The linear algebra solution is compared with the non-
linear optimization algorithms solution. Computer si-
mulation results prove that it is interesting to use this
algorithms in spite of their expensive calculative cost.
REFERENCES
Abderrahim, K., Ben Abdennour, R., Favier, G., Ksouri, M.,
and Msahli, F. (2001). New Results on FIR Identifica-
tion using Cumulants. Journal Européen des Systèmes
Automatisés-JESA, Vol. 35, No. 5, pp. 601-622.
Alshebeili, S. A., Venetsanopoulos, A. N., and Cetin, A.
E. (1993, April). Cumulant Based Identification Ap-
proaches for Nonminimum Phase FIR Systems. IEEE
Transactions on Signal Processing, Vol. 41, No. 4, pp.
1576-1588.
ICINCO 2005 - SIGNAL PROCESSING, SYSTEMS MODELING AND CONTROL
14