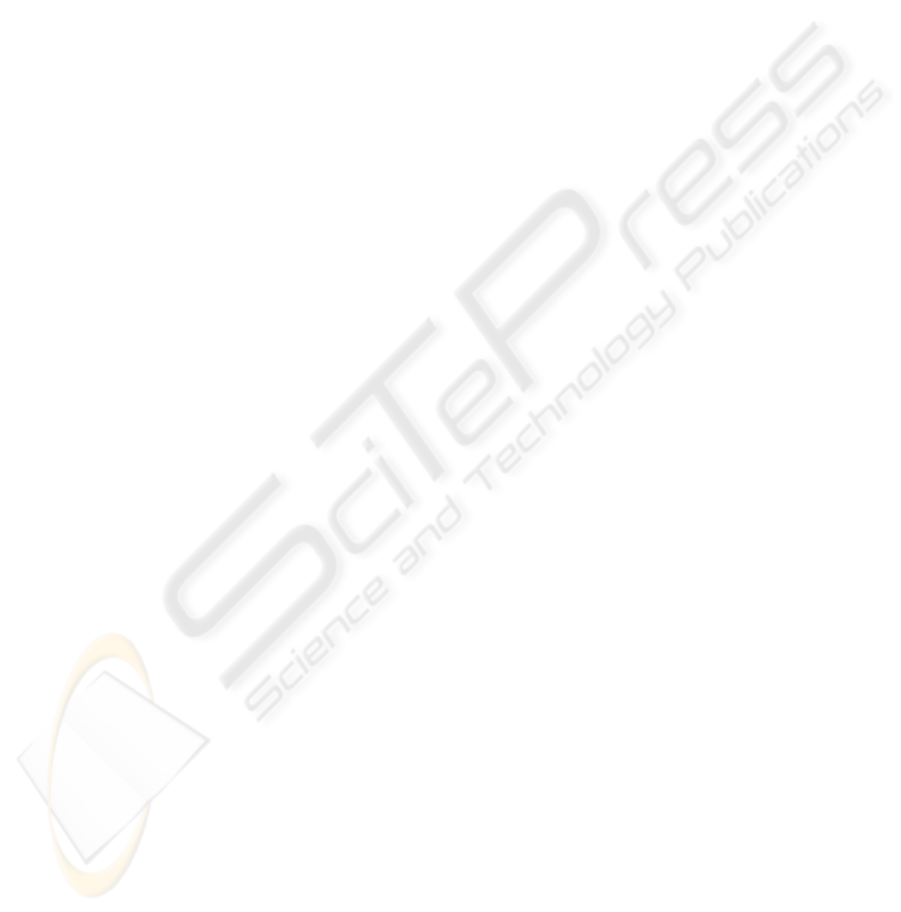
Interpretation of
o
q and inversion of Riccati matrix
Q is non-standard: they represent stationary covari-
ance matrices of the optimal inputs and states, respec-
tively.
4 DISCUSSION
Design of the optimal strategy reduces to the solution
of the stationary version of the integral filtering equa-
tion (5) and of the solution of the integral equation (8).
For the normal state-space model and the normal ideal
pdf, it reduces to the stationary version of Kalman fil-
ter and design minimising quadratic criterion (Med-
itch, 1969). Even in this case, the FPD interpretation
brings practical advantages, as it interprets the penal-
isation matrices as inversions of the ideal covariance
matrices and thus guides on their choice. Moreover,
when they are recursively (approximately) estimated,
the weights adapts to the varying noise level, which
generally spares the input effort as the control of un-
controllable innovations is given up.
Generally, the closed-form solutions of discussed
equations exist rarely but the explicit form of con-
trol laws simplifies numerical approximations sub-
stantially. The stationary form of the solution pre-
pares such approximations even better as ””only” the
stationary functions f (x
t
|V
t
) and ω(ψ
t
, V
t−1
) have to
be approximated (not sequences of such functions).
Non-linear character of the filtering and the de-
sign equations together with a generic high dimen-
sionality of their domain restrict supply of available
approximation techniques. Essentially, a global ap-
proximation suitable for higher dimensions has to be
used. The neural networks (Haykin, 1994), ideally in-
terpreted as finite probabilistic mixtures (Titterington
et al., 1985) and general ANOVA-like approximations
(Rabitz and Alis, 1999) seem to be prime candidates.
Especially, the mixture versions look promising as
there are approximate techniques for FPD with them
(Murray-Smith and Johansen, 1997; K
´
arn
´
y et al.,
2003).
ACKNOWLEDGEMENTS
This research has been partially supported by the
grants GA
ˇ
CR 102/03/0049, GA
ˇ
CR 102/03/P010 and
by the grant 1M6798555601 of the Czech Ministry of
Education, Youth and Sports.
REFERENCES
Berger, J. (1985). Statistical Decision Theory and Bayesian
Analysis. Springer-Verlag, New York.
Haykin, S. (1994). Neural networks: A comprehensive
foundation. Macmillan College Publishing Company,
New York.
K
´
arn
´
y, M. (1996). Towards fully probabilistic control de-
sign. Automatica, 32(12):1719–1722.
K
´
arn
´
y, M., B
¨
ohm, J., Guy, T., Jirsa, L., Nagy, I., Nedoma,
P., and Tesa
ˇ
r, L. (2005). Optimized Bayesian Dynamic
Advising: Theory and Algorithms. Springer, London.
to appear.
K
´
arn
´
y, M., B
¨
ohm, J., Guy, T. V., and Nedoma, P. (2003).
Mixture-based adaptive probabilistic control. In-
ternational Journal of Adaptive Control and Signal
Processing, 17(2):119–132.
K
´
arn
´
y, M. and Guy, T. (2004). Fully probabilistic control
design. Systems & Control Letters. submitted.
Kullback, S. and Leibler, R. (1951). On information and
sufficiency. Annals of Mathematical Statistics, 22:79–
87.
Kushner, H. (1971). Introduction to stochastic control.
Holt, Rinehart and Winston, New York, San Fran-
cisco, London.
Lee, J.M. and Lee, J.H. (2004). Approximate dynamic
procgramming strategies and their applica bility for
process control: A review and future directions. Inter-
national Journal of Control, 2(3):263–278.
Meditch, J. (1969). Stochastic Optimal Linear Estimation
and Control. Mc. Graw Hill.
Murray-Smith, R. and Johansen, T. (1997). Multiple Model
Approaches to Modelling and Control. Taylor & Fran-
cis, London.
Peterka, V. (1981). Bayesian system identification. In
Eykhoff, P., editor, Trends and Progress in System
Identification, pages 239–304. Pergamon Press, Ox-
ford.
Rabitz, H. and Alis, O.(1999). General foundations of high-
dimensional model representations. Journal of Math-
ematical Chemistry, 25:197–233.
Titterington, D., Smith, A., and Makov, U. (1985). Statis-
tical Analysis of Finite Mixtures. John Wiley & Sons,
Chichester, New York, Brisbane, Toronto, Singapore.
ISBN 0 471 90763 4.
ICINCO 2005 - INTELLIGENT CONTROL SYSTEMS AND OPTIMIZATION
112