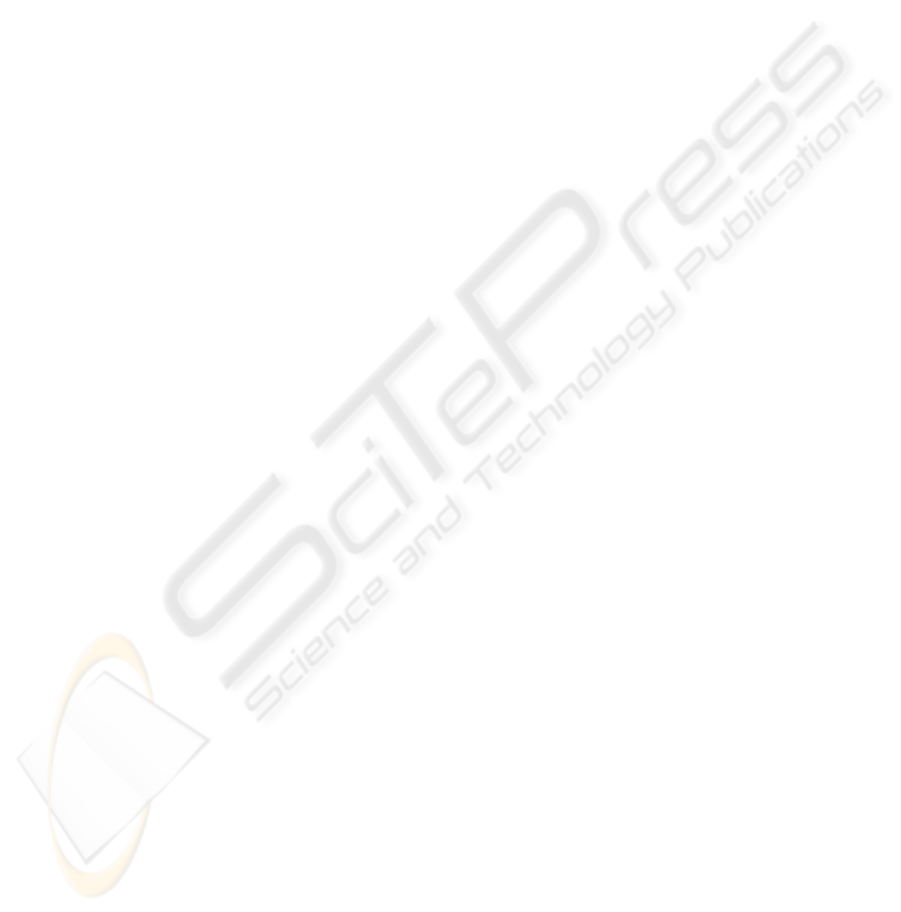
determining the objective variables we subdivided the
track section into 100 parts of 1 m each; thus for any
possible moving direction an evaluation vector com-
prising 100 elements was recorded for each crossing.
For an objective we used the maximum of deviations
from the middle position of the car body in the respec-
tive part; it was measured by the existing position-
measuring sensors. One run over the track section in
view takes 10s at an assumed speed v = 10m/s. Af-
ter each crossing the evaluation vectors are transmit-
ted to the learning algorithm which defines the new
trajectories of the disturbance compensation for the
next crossing. These trajectories are parameterised
over 100 supporting points corresponding to the sec-
tions. As shown in fig. 4 the signal f is interpo-
lated between the supporting points. The algorithm
required to determine an optimal disturbance com-
pensation was implemented on a dSPACE real- time
hardware, in addition to the testbed control described.
Here the focus was on testing the learning algorithm.
Difficulties resulting from the necessary communica-
tion between vehicle and processing of information
on the track were ignored here. At the time interval
where the crossing is finished the detected evaluation
variables are transmitted to the optimising procedure
which then computes the new trajectories of the dis-
turbance compensation at exactly the same time in-
terval and makes them available for the next crossing
that begins at the next time interval.
6 RESULTS
This section describes the results of the optimisation
on the basis of measurements at the testbed. The re-
curring excitation over the track section in question is
displayed in Fig. 6 to 8 at the top. In translatory di-
rection the chassis was at first excited by a sinusoidal
signal and subsequently by three steps in lateral direc-
tion resp. inversely in vertical direction. Additionally
a superposed sinusoidal rotation around the longitu-
dinal axis of the chassis took effect. The middle dia-
grams display the disturbance trajectory acquired by
repeated runs in the course of optimisation. The plot
shows the characteristics as follows: at the outset of
the optimisation as a dotted line, after five crossings as
a broken one, and as an unbroken line after 50 cross-
ings. The lower part displays the corresponding plots
of the evaluation functions.
All in all the optimisation method has proved its
ability to compensate the car body movements almost
in full for the periodically recurring excitations. After
only five crossings the amplitudes of the body motion
fell below 10%. After 50 crossings the carriage body
is nearly in a position of rest in spite of the excitation.
Another aspect is made clear in a comparison of
the excitation behaviors and the corresponding dis-
turbance compensation. With a vertical motion these
variables converge while with torsion and lateral mo-
tion there remain significant differences even after 50
repetitions. This is due to the coupling of motions.
A lateral excitation will always bring about a torsion
in the car body; vice versa, a rotation of the chas-
sis around the longitudinal axis will always affect the
lateral motion of the car body. This is why the dis-
turbance compensation has to take into account these
couplings, the result being the behavior shown. On
the other hand, the car body motion in vertical direc-
tion is decoupled from the other degrees of freedom;
thus the disturbance compensation will only have to
deal with the excitation portion in this direction.
7 CONCLUSION
It was shown that using existing data processing in-
frastructure for the exchange of collected data can be
effective for the compensation of recurring stationary
disturbances. The realization at the testbed confirmed
the advantages of this approach. However it may not
be ignored that the trajectories of the compensation
do not represent the disturbances themselves. Rather
they are optimized with respect to the particularly re-
garded vehicle and its velocity. At the testbed this
causes no problem, because the dynamics and the as-
sumed velocity of the testbed does not change during
a test. Of course in reality different vehicles must be
considered. In this case the compensation adapted to
a particular vehicle cannot be used. In order to be able
to use the presented method nevertheless, vehicle and
velocity independent information must be stored. For
this purpose the actual track characteristics are ideal
which can be determined by observation from the re-
spective system response of an individual vehicle. In
this way the approach introduced here can be gener-
alized on different types of vehicles.
Thus the method represents a good way to improve
the dynamic behavior for repetitive motions. It can
also be transferred to other applications, which show
similar characteristics.
To prove the convergence of the learning algorithm
a simplified convergence analysis was performed by
using methods derived from digital control theory.
An enlargement of the convergence model in view of
a concurrent analysis of several supporting points is
possible and will be object of future research.
REFERENCES
Donahue, M. D. (2001). Implementation of an active sus-
pension, preview controller for improved ride com-
ICINCO 2005 - INTELLIGENT CONTROL SYSTEMS AND OPTIMIZATION
36