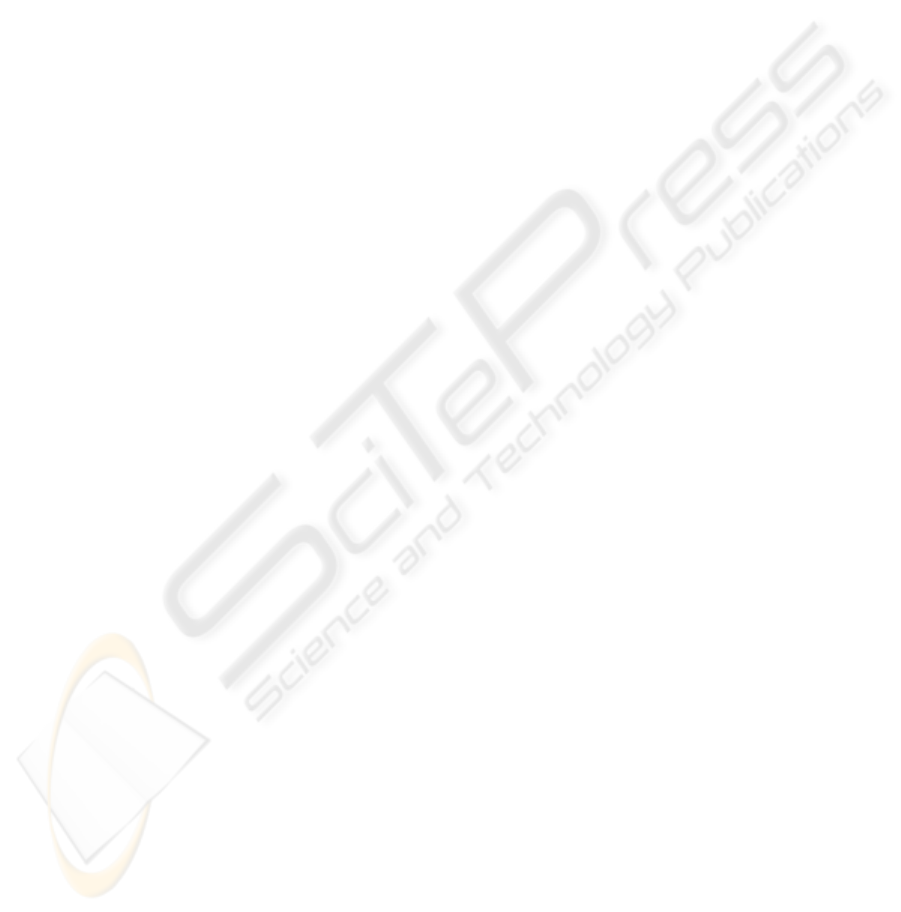
ROBUST STABILITY ANALYSIS OF SINGULARLY
PERTURBED MAGNETIC SUSPENSION SYSTEMS
§
Nan-Chyuan Tsai, Chien-Ting Chen
Department of Mechanical Engineering, National Cheng Kung University,
Tainan City, 701,Taiwan
Keywords: Singular perturbation, Two-time-scale systems, Kharitonov Theorem.
Abstract: For a singularly perturbed magnetic suspension system, two kinds of state feedback controllers are
synthesized to account for the inherent instability of the open-loop plant with two-time-scale properties.
Kharitonov polynomials, extremal vertex and uncertain Nyquist plot are employed to examine the maximum
tolerance against system parameters uncertainties such that the stability of the closed-loop system is still
retained. Experimental simulations are reported to illustrate the robustness of designed controllers both in
stability and performance. At last, Interlacing Theorem is introduced to analyze the stability of uncertain
suspension systems via the characteristic interval polynomials. It is found that identical results are obtained,
in comparison with extremal vertex approach.
1 INTRODUCTION
In general, two-time-scale systems are not
uncommon in practice, such as electro-mechanical
systems, power engineering and so on. The major
feature of this kind of singularly perturbed systems
is the eigenvalues of the plant are located distinctly
apart into two sectors: one near the origin on
complex frequency plane and the other far away. In
other words, two-time-scale system can be analyzed
as a coupled system composed by a slow subsystem
and a fast subsystem.
The singular perturbation technique is employed
to decouple the slow subsystem and the fast
subsystem by choosing an appropriate coordinate
transformation such that the controller for the two-
time-scale system can be designed individually by
two uncoupled models at first and then composed
together. Numerous researchers had applied this
concept to synthesize the singularly perturbed
industrial control systems. Vournas et al. reported
to design the generator voltage regulator by singular
perturbation method (Vournas, 1995). Flexible
robot links have been discussed and presented
frequently by this technique (Spong, 1989).
Suspension of quarter-car model has been analyzed
by two-time-scale model (Salman, 1988). However,
the robustness in singularly perturbed suspension
has not much been addressed.
In this work, the magnetic suspension system is
investigated both in robust stability analysis and in
control synthesis. The parasitic parameter, that is
crucial in singular perturbation system, is found to
be strongly related to the inductance value of the
electromagnet. Unfortunately, some of the
parameters are not only of small values, but also
inherent in uncertainties. Therefore, Kharitonov
Theorem is introduced to analyze how robust the
closed-loop system will be, from the viewpoint of
stability with respect to system parameters
variations. Two kinds of controllers are synthesized
and compared in experimental simulations. It is
concluded that the designed controllers exhibit
dramatically robust enough to account for parameter
uncertainties up to
± 50% variation away from the
nominal values, while the performances of the
closed-loop system are not detrimentally degraded.
2 PROBLEM FORMULATION
The magnetic suspension system (Fujita, 1995),
shown in Figure 1, is inherently unstable so that a
closed-loop control strategy is required.
166
Tsai N. and Chen C. (2005).
ROBUST STABILITY ANALYSIS OF SINGULARLY PERTURBED MAGNETIC SUSPENSION SYSTEMS.
In Proceedings of the Second International Conference on Informatics in Control, Automation and Robotics - Signal Processing, Systems Modeling and
Control, pages 166-172
DOI: 10.5220/0001188001660172
Copyright
c
SciTePress