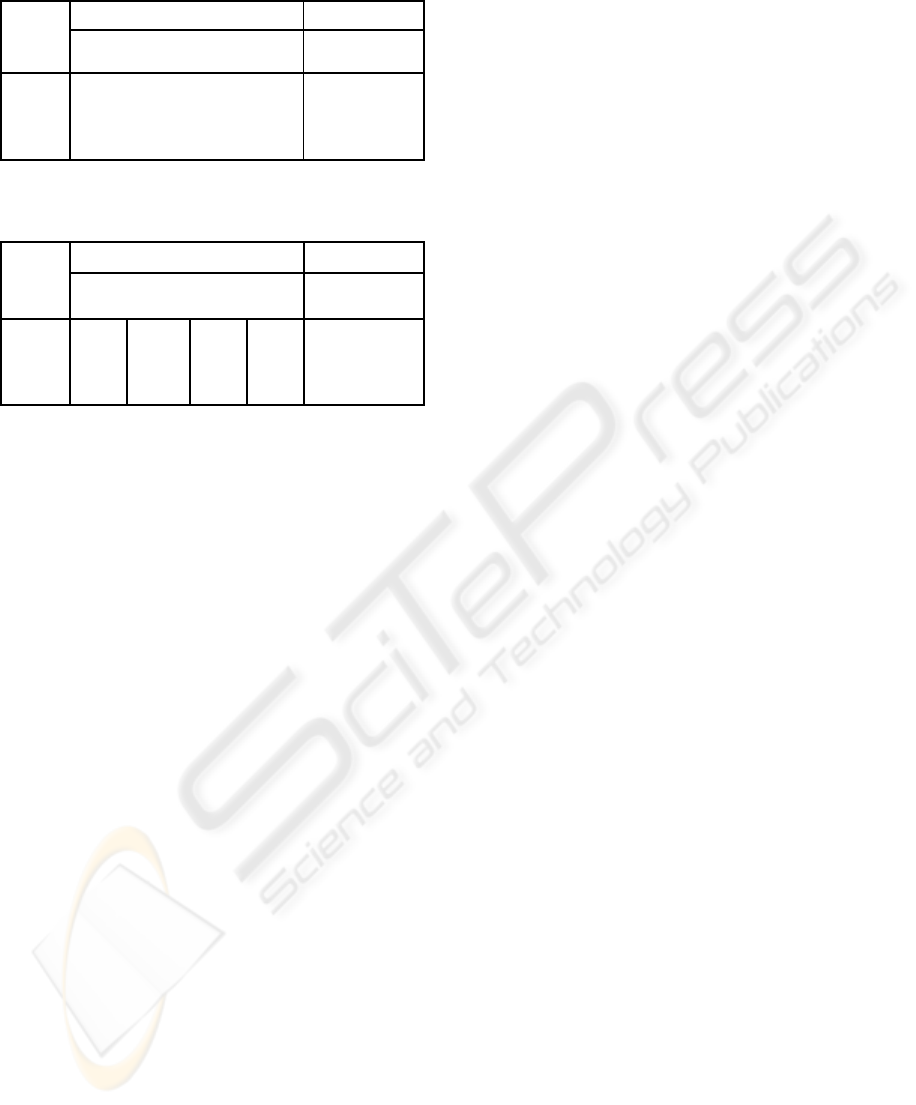
Table 4: Classification hit rates in % (C/S)
New approach Englehart
WT WPT
Sig.
length
/chnls
CO4 SY5 CO4 SY5
DWT
C4
WPT
S5
200/1 75.0 76.7 79.2 79.2 60.8 62.5
200/4 90.0 94.2 94.2 95.0 86.7 88.3
400/1 80.8 80.0 80.8 77.5 60.8 59.2
400/4 99.2 96. 7 98.3 97.5 88.3 93.3
Table 5: Classification hit rates in % (L/P)
New approach Englehart
WT WPT
Sig.
length
/chnls
CO4 SY5 CO4 SY5
WT WPT
200/1 73.3 81.7 81.7 83.3 56.7 53.3
200/4 91.7 96.7 90.0 98.3 80.0 93.3
400/1 96.7 95.0 93.3 91.7 56.7 63.3
400/4 99.9 99.9 99.9 99.9 88.3 95.0
11 CONCLUSIONS
A new approach of wavelet-based feature extraction
from temporal signals has been proposed. The ap-
proach extends the Englehart's discrete wavelet trans-
form and wavelet packet transform by subjecting the
two-scale, three-sequence wavelet coefficients to
temporal moment computation. This has helped re-
duce significantly the dimensionality of the resulting
feature vectors without loosing the essential informa-
tion in the original patterns. It was found experimen-
tally that first five raw moments represent a good
compromise. The new methods are applied to pre-
hensile EMG signals of various lengths and various
amounts of input signals (surface EMG channels)
and compared to the best approaches of Englehart, on
the same set of signals. For the comparison are used
two quantitative measures: Hotelling statistic and
classification hit rates. The classifier applied to the
extracted features was linear support vector machine,
which has exceptionally good performance in case of
large feature spaces and fewer training samples. The
results have shown superior performance of the new
approach. A brief complexity analysis also shows
that the new approach is more efficient time wise.
Although the methodology was demonstrated on
EMG signals, we believe the methodology can
equally successfully be applied to other temporal
signals.
REFERENCES
Christianini N., Shawe-Taylor, 2000. An Introduction to
Support Vector Machines. Cambridge Univ. Press.
Du S. and Vuskovic M., 2004. Temporal vs. Spectral
approach to Feature Extraction from Prehensile EMG
Signals. In IEEE Int. Conf. on Information Reuse and
Integration (IEEE IRI-2004), Las Vegas, Nevada.
Englehart K., Hudgins B., Parker P. and Stevenson M.,
1998. Time-frequency representation for classification
of the transient myoelectric signal. In ICEMBS’98.
Proceedings of the 20th Annual International Confer-
ence on Engineering in Medicine and Biology Society.
ICEMBS Press.
Englehart K., 1998a. Signal Representation for Classifica-
tion of the Transient Myoelectric Signal. Doctoral
Thesis. University of New Brunswick, Fredericton,
New Brunswick, Canada.
Englehart K., Hudgins B., Parker P. and Stevenson M.,
1999. Improving Myoelectric Signal Classification us-
ing Wavelet Packets and Principle Component Analy-
sis. In ICEMBS’99. Proceedings of the 21st Annual In-
ternational Conference on Engineering in Medicine
and Biology Society, ICEMBS Press.
Englehart K., Hudgins B., Parker P., 2001. A Wavelet –
Based Continuous Classification Scheme for Multi-
fucntion Myoelectric Control. In IEEE Transactions
on Biomedical Engineering, vol. 48, No. 3, pp. 302-
311.
Farry, K. A., Walker I. D., Baraniuk R. G., 1996.
Myoelectric Teleoperation of a Complex Robotic
Hand. IEEE Trans On Robotic and Automation, Vol.
12, No.5.
Gunn S.R., 1997. Support Vector Machines for Classifica-
tion and Regression. Technical Report, Image Speech
and Intelligent Systems Research Group, University of
Southampton.
Hannaford B. and Lehman S., 1986. Short Time Fourier
Analysis of the Electromyogram: Fast Movements and
Constant Contraction. In IEEE Transactions On Bio-
medical Engineering. BME-33,
Han-Pan Huang H-P, Chen C_Y., 1999. Development of a
Myoelectric Discrimination System for Multi-Degree
Prosthetic Hand. Proc. of the 1999 International Con-
ference on Robotics and Automation, Detroit, May pp.
2392-2397.
Hudgins, P. Parker and R.N. Scott, 1991. A Neural Net-
work Classifier for Multifunctional Myoelectric Con-
trol. Annual Int. Conf. Of the EMBS, Vol. 13, No. 3,
pp. 1454-1455.
Hudgins B., Parker P. and Scott R. N., 1993. A New Strat-
egy for Multifunctional Myoelectric Control. In IEEE
Transactions on Biomedical Engineering, vol. 40, No.
1, pp. 82-94.
Saito N. and Coifman R. R., 1995. Local Discriminant
Basis and their applications. J. Math. Imag. Vis., Vol.
5, no 4, pp. 337-358.
Nishikawa D, Yu W. Yokoi H, and Kakazu Y, 1991. EMG
Prosthetic Hand Controller using Real-Time Learning
WAVELET TRANSFORM MOMENTS FOR FEATURE EXTRACTION FROM TEMPORAL SIGNALS
77