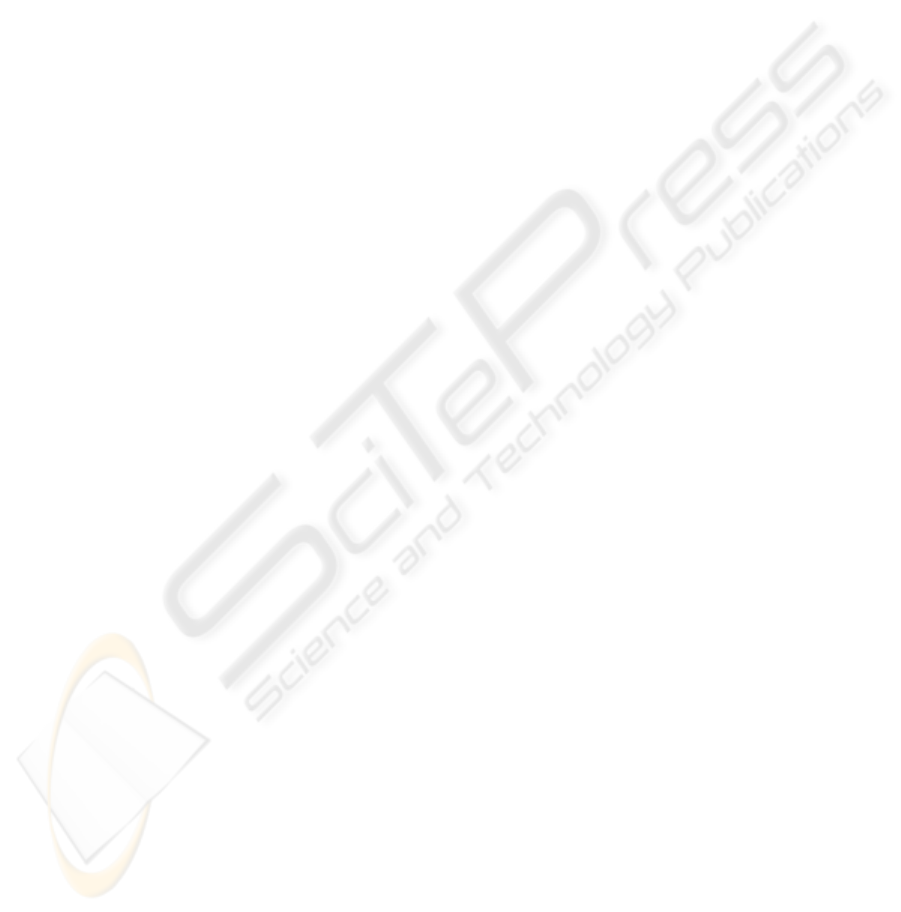
5. Connell, J. and Viola, P. (1990). Cooperative control of a semi-autonomous mobile robot. In
Proc. of the 1990 IEEE International Conf. on Robotics and Automation.
6. Duvivier, D., Preux, P., C.Fonlupt, Robilliard, D., and Talbi, E.-G. (1998). The fitness func-
tion and its impact on local search methods. In Proc. of the IEEE International Conf. on
Systems, Man, and Cybernetics, volume 3.
7. Floreano, D. and Mondada, F. (1994). Automatic creation of an autonomous agent: Genetic
evolution of a neural-network driven robot. In From Animals to Animats 3: Proc. of the Third
International Conf. on Simulation of Adaptive Behavior.
8. Hoque, T., Chetty, M., and Dooley, L. (2004). An efficient algorithm for computing the
fitness function of a hydrophobic-hydrophilic model. In Proc. of the IEEE International
Conf. on Evolutionary Computation, volume 1.
9. Houck, C., Joines, J., and Kay, M. (1995). A Genetic Algorithm for Function Optimization:
A Matlab Implementation. NCSU-IE TR 95-09.
10. Lippmann, R. P. (1989). Pattern classification using neural networks. In IEEE Communica-
tions Magazine.
11. Maher, M. and Poon, J. (1995). Co-evolution of the fitness function and design solution for
design exploration. In Proc. of the IEEE International Conf. on Evolutionary Computation,
volume 1.
12. Nehmzow, U. (2002). Physically embedded genetic algorithm learning in multi-robot sce-
narios: The pega algorithm. In Proc. of the Second International Workshop on Epigenetic
Robotics: Modeling Cognitive Development in Robotic Systems, volume 94, Lund, Sweden.
Lund University Cognitive Studies.
13. Ripley, B. D. (1994). Flexible non-linear approaches to classification. In Cherkassky, V.,
Friedman, J. H., and Wechsler, H., editors, From Statistics to Neural Networks. Theory and
Pattern Recognition Applications, Berlin. Springer Verlag.
14. Saxena, A. and Saad, A. (2004). Genetic algorithms for ann-based condition monitoring
system design for rotating mechanical systems. In 9th Online World Conf. on Soft Computing
in Industrial Applications.
15. Sorokin, S. N., Savelyev, V. V., Ivanchenko, E. V., and Oleynik, M. P. (2002). Fitness func-
tion calculation technique in yagi-uda antennas evolutionary design. In Proc. of the IEEE
International Conf. on Mathematical Methods in Electromagnetic Theory, volume 2.
16. Spears, W. M., DeJong, K. A., Baeck, T., Fogel, D. B., and deGaris, H. (1993). An overview
of evolutionary computation. In Proc. of the 1993 European Conf. on Machine Learning.
17. Stanley, K. O. and Miikkulainen, R. (2002). Evolving neural networks through augmenting
topologies. In Evolutionary Computation, volume 10, Cambridge. MIT Press.
18. Storm, T. (2004). KIKS is Khepera Simulator 2.2.0. (http://www.tstorm.se/projects/kiks/).
19. Wagner, A. and Arkin, R. C. (2003). Internalized plans for communication-sensitive robot
team behaviors. In Proc. of the IEEE/RSJ Conf. on Intelligent Robots and Systems.
20. Whitley, L. D. (1994). A genetic algorithm tutorial. In Statistics and Computing, volume 4.
21. Yao, X. (1999). Evolving artificial neural networks. In Proc. of the IEEE, volume 87.