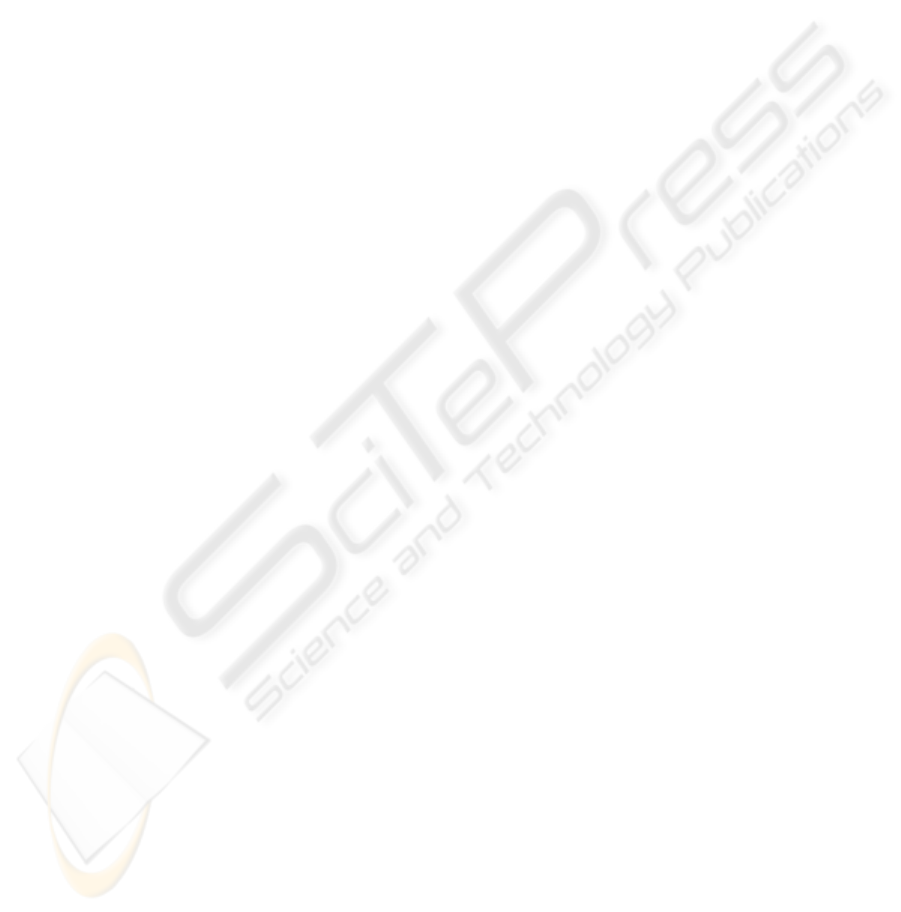
Table 1 shows the experimental results. The results of the testing show that with the
system described can classify the five vowels with an accuracy of up to 91%. The
higher classification accuracy is due to better discriminating ability of neural network
architecture and RMS of EMG as the features. At the present stage, the method has
been tested successfully with only three subjects. In order to evaluate the intra and
inter variability of the method, a study on a larger experimental population is
required. Fig.3-4 depicts the statistical bar diagrams of the three sub-auditory RMS of
EMG data. However, due to the small data bank, it is difficult to determine and
conclude the significance of the same.
6 Conclusion
This paper describes a study to recognise human sub-auditory speech signal based on
the EMG data extracted from the three articulatory facial muscles coupled with neural
networks. Test results show recognition accuracy of 91 %. The system is accurate
when compared to other attempts for EMG based sub-auditory speech recognition.
These preliminary results suggest that the study is suitable to develop a real-time
EMG based voiceless communication system.
7 Further Work
Authors are working with statistically larger population of experimental subjects.
References
1. M.S. Morse, Y.N. Gopalan, M. Wright: Speech recognition using myoelectric signals with
neural network, Annual International Conference of the IEEE Engineering in Medicine and
Biology Society, Vol.13, No.4, pp.1977-1878, 1991.
2. A.D.C. Chan, K.E., B. Hudgins, D.F. Lovely, Myo-electric signals to augment speech
recognition. Medical & Biological Engineering & Computing, 2001. 39: p. 500-504.
3. Hiroyuki Manabe, “Unvoiced Speech Recognition using EMG - Mime Speech Recognition
–“ Short Talks: Specialized Section CHI 2003: NEW HORIZONS Short Talk: Brains, Eyes
and Ears CHI 2003: NEW HORIZONS NTT DoCoMo
MultimediaLaboratories†manabe@mml.yrp.nttdocomo.co.jp
4. N. Sugie, K. Tsunoda,: A speech prosthesis employing a speech synthesizer. IEEE
Transaction on Biomedical Engineering, Vol.BME-32, No.7, pp.485- 490, 1985.
5. Chuck Jorgensen, Diana D Lee & Shane “Sub Auditory Speech Recognition Based on
EMG Signals” Agabon. , Proc. of the IEEE conference, 2003
6. Akira Hiraiwa NTT DoCoMo Multimedia Laboratories hiraiwa@mml.yrp.nttdocomo.co.jp
Toshiaki Sugimura NTT DoCoMo Multimedia Laboratories
sugi@mml.yrp.nttdocomo.co.jp
7. A J Fridlund, J.T.C., Guidelines for human electrographic research. Psycholphysiology,
1986. 23: p. 567-589.
8. A. Freeman and M. Skapura, Neural Networks: Algorithms, Applications, and
Programming Techniques, Addison-Wesley, Mass., 1991.
147